A Smoothing Proximal Gradient Algorithm for Matrix Rank Minimization Problem.
Computational optimization and applications(2022)
摘要
In this paper, we study the low-rank matrix minimization problem, where the loss function is convex but nonsmooth and the penalty term is defined by the cardinality function. We first introduce an exact continuous relaxation, that is, both problems have the same minimizers and the same optimal value. In particular, we introduce a class of lifted stationary points of the relaxed problem and show that any local minimizer of the relaxed problem must be a lifted stationary point. In addition, we derive lower bound property for the nonzero singular values of the lifted stationary point and hence also of the local minimizers of the relaxed problem. Then the smoothing proximal gradient (SPG) algorithm is proposed to find a lifted stationary point of the continuous relaxation model. Moreover, it is shown that any accumulating point of the sequence generated by SPG algorithm is a lifted stationary point. At last, numerical examples show the efficiency of the SPG algorithm.
更多查看译文
关键词
Low-rank approximation,Nonsmooth convex loss function,Smoothing method,15A03,15A83,90C30,65K05
AI 理解论文
溯源树
样例
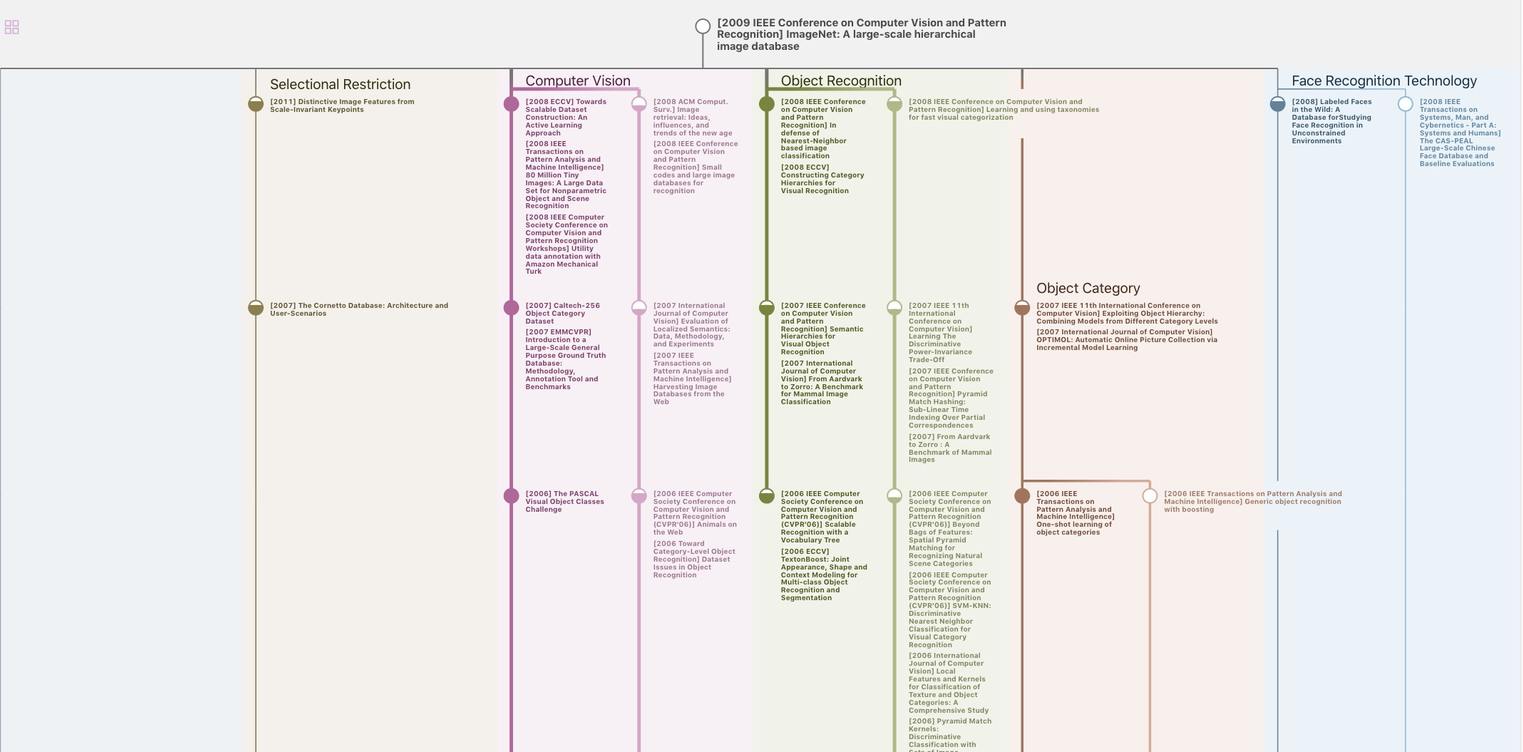
生成溯源树,研究论文发展脉络
Chat Paper
正在生成论文摘要