A small samples training framework for deep Learning-based automatic information extraction: Case study of construction accident news reports analysis
Advanced Engineering Informatics(2021)
摘要
Knowledge management is crucial for construction safety management. Widely collected and well-organized safety-related documents are recognized to be significant in raising the workers' security awareness and then to prevent hazards and accidents. To improve document processing efficiency, automatic information extraction plays an important role. However, currently, automatic information extraction modeling requires large scale training datasets. It is a big challenge for the engineering industry, especially for the fields which heavily rely on the experts’ knowledge. Limited data sources, and high time and labor costs make it not practical to establish a large-scale dataset. This work proposed a natural language data augmentation-based small samples training framework for automatic information extraction modeling. With the designed cross combination-based text data augmentation algorithm, the deep neural network can be employed to build up automatic information extraction models without large-scale raw data and manual annotations. Characters semantic coding is employed to avoid word segmentation and make sure that the framework can be utilized in different writing language systems. The BiLSTM-CRF model is adopted as the detection core to conduct character classification. Through a case study of two independent accident news report datasets analysis, the proposed framework has been validated. A reliable and robust automatic information extraction model can be established, even though with small samples training.
更多查看译文
关键词
Automatic information extraction,Small sample training,Cross combination-based text augmentation,Construction accident news reports
AI 理解论文
溯源树
样例
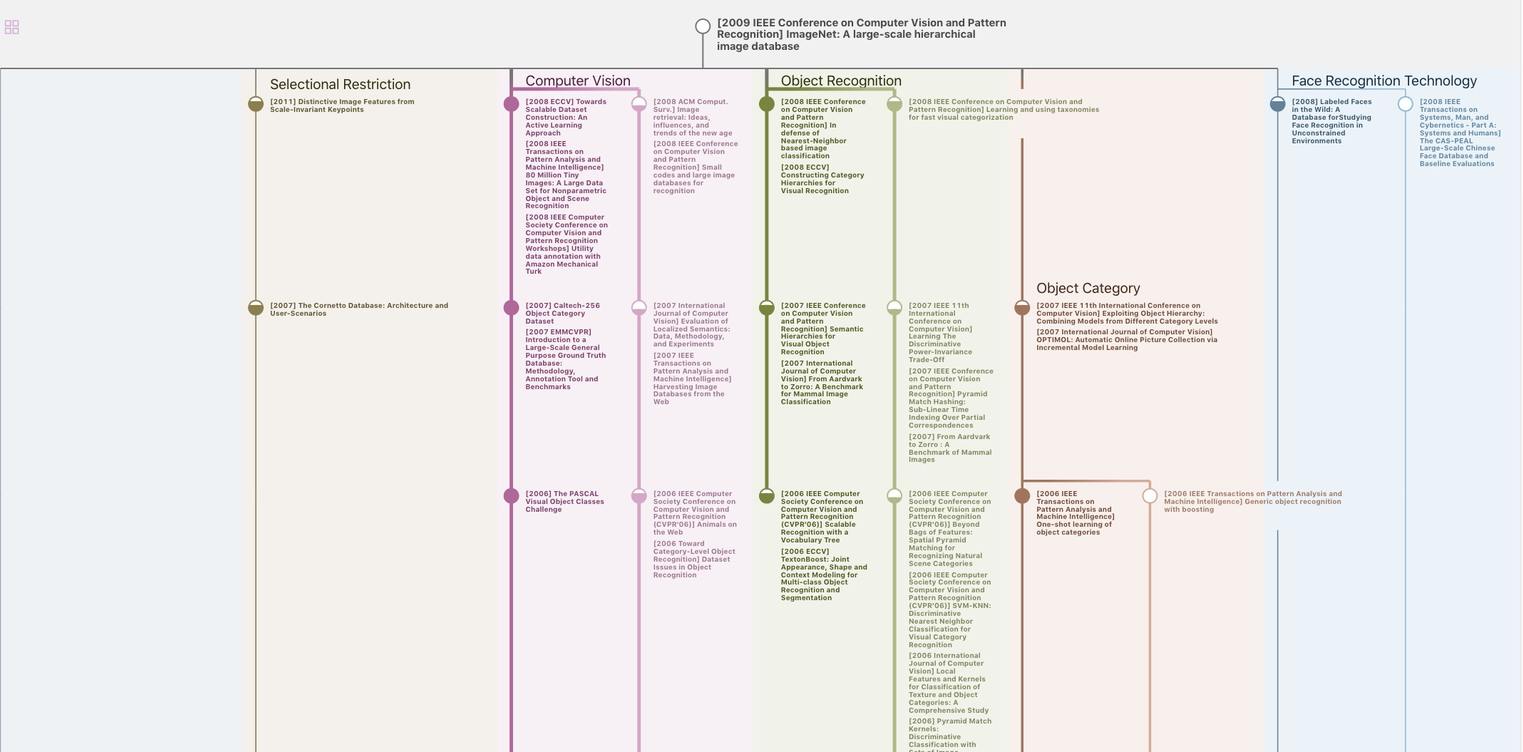
生成溯源树,研究论文发展脉络
Chat Paper
正在生成论文摘要