Automated feature-based grading and progression analysis of diabetic retinopathy.
Eye (London, England)(2021)
摘要
BACKGROUND:In diabetic retinopathy (DR) screening programmes feature-based grading guidelines are used by human graders. However, recent deep learning approaches have focused on end to end learning, based on labelled data at the whole image level. Most predictions from such software offer a direct grading output without information about the retinal features responsible for the grade. In this work, we demonstrate a feature based retinal image analysis system, which aims to support flexible grading and monitor progression.
METHODS:The system was evaluated against images that had been graded according to two different grading systems; The International Clinical Diabetic Retinopathy and Diabetic Macular Oedema Severity Scale and the UK's National Screening Committee guidelines.
RESULTS:External evaluation on large datasets collected from three nations (Kenya, Saudi Arabia and China) was carried out. On a DR referable level, sensitivity did not vary significantly between different DR grading schemes (91.2-94.2.0%) and there were excellent specificity values above 93% in all image sets. More importantly, no cases of severe non-proliferative DR, proliferative DR or DMO were missed.
CONCLUSIONS:We demonstrate the potential of an AI feature-based DR grading system that is not constrained to any specific grading scheme.
更多查看译文
AI 理解论文
溯源树
样例
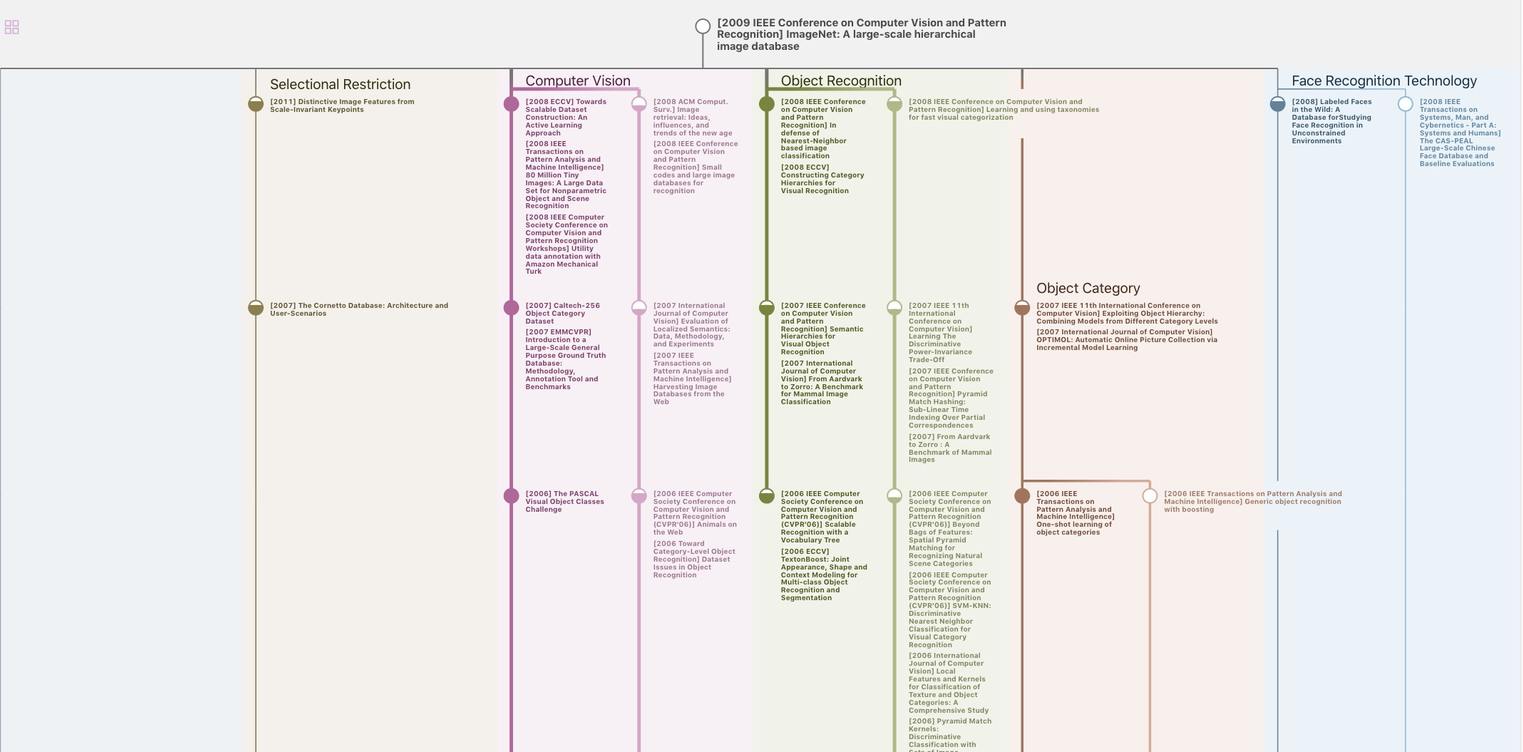
生成溯源树,研究论文发展脉络
Chat Paper
正在生成论文摘要