Robust Vision-Based Cheat Detection in Competitive Gaming
Proceedings of the ACM on Computer Graphics and Interactive Techniques(2021)
摘要
AbstractGame publishers and anti-cheat companies have been unsuccessful in blocking cheating in online gaming. We propose a novel, vision-based approach that captures the frame buffer's final state and detects illicit overlays. To this aim, we train and evaluate a DNN detector on a new dataset, collected using two first-person shooter games and three cheating software. We study the advantages and disadvantages of different DNN architectures operating on a local or global scale. We use output confidence analysis to avoid unreliable detections and inform when network retraining is required. In an ablation study, we show how to use Interval Bound Propagation (IBP) to build a detector that is also resistant to potential adversarial attacks and study IBP's interaction with confidence analysis. Our results show that robust and effective anti-cheating through machine learning is practically feasible and can be used to guarantee fair play in online gaming.
更多查看译文
关键词
neural networks, cheat detection, competitive gaming
AI 理解论文
溯源树
样例
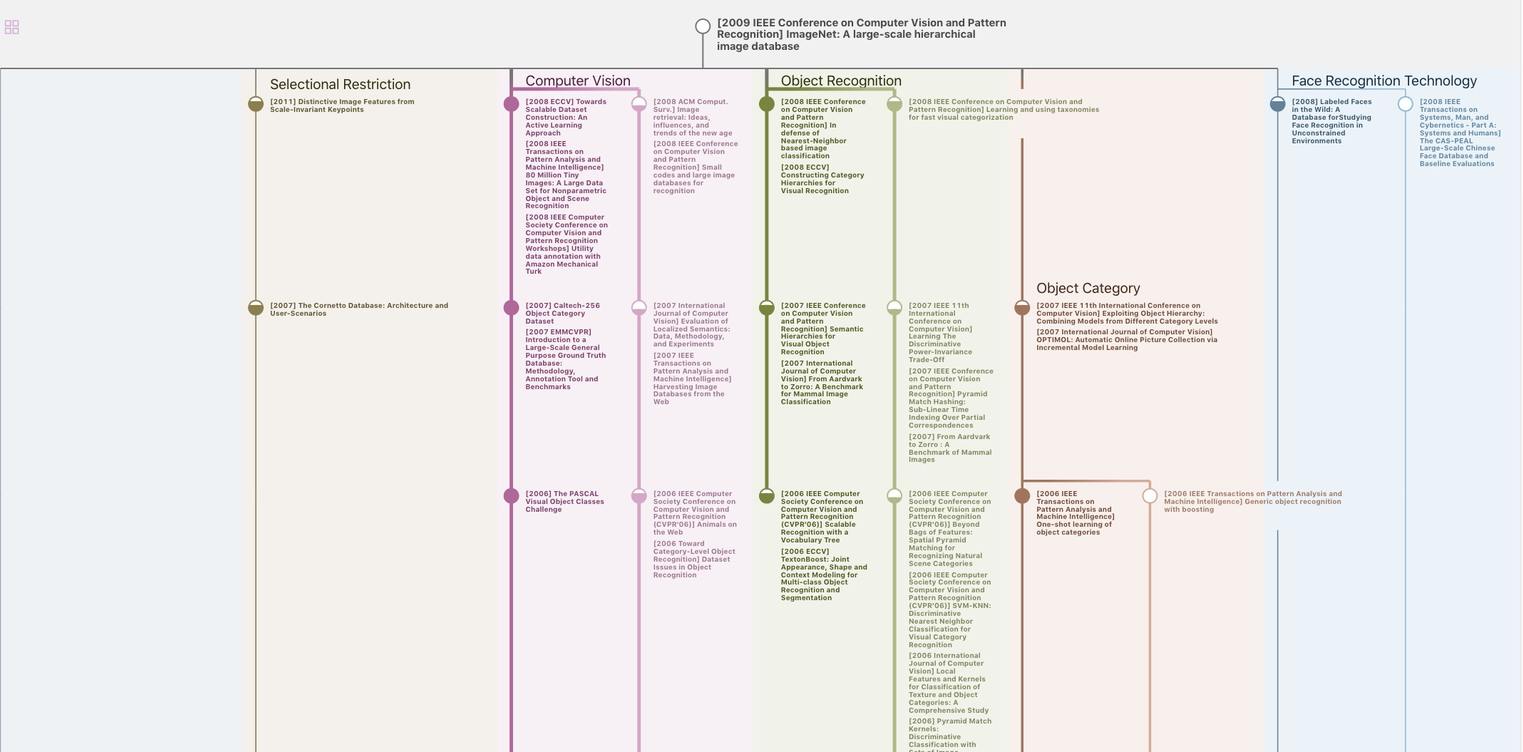
生成溯源树,研究论文发展脉络
Chat Paper
正在生成论文摘要