Identification of Partial-Differential-Equations-Based Models from Noisy Data via Splines
arxiv(2023)
摘要
We propose a two-stage method called \textit{Spline Assisted Partial Differential Equation based Model Identification (SAPDEMI)} to identify partial differential equation (PDE)-based models from noisy data. In the first stage, we employ the cubic splines to estimate unobservable derivatives. The underlying PDE is based on a subset of these derivatives. This stage is computationally efficient: its computational complexity is a product of a constant with the sample size; this is the lowest possible order of computational complexity. In the second stage, we apply the Least Absolute Shrinkage and Selection Operator (Lasso) to identify the underlying PDE-based model. Statistical properties are developed, including the model identification accuracy. We validate our theory through various numerical examples and a real data case study. The case study is based on a National Aeronautics and Space Administration (NASA) data set.
更多查看译文
关键词
partial-differential-equations-based partial-differential-equations-based partial-differential-equations-based,splines,models,partial-differential-equations-based partial-differential-equations-based,noisy
AI 理解论文
溯源树
样例
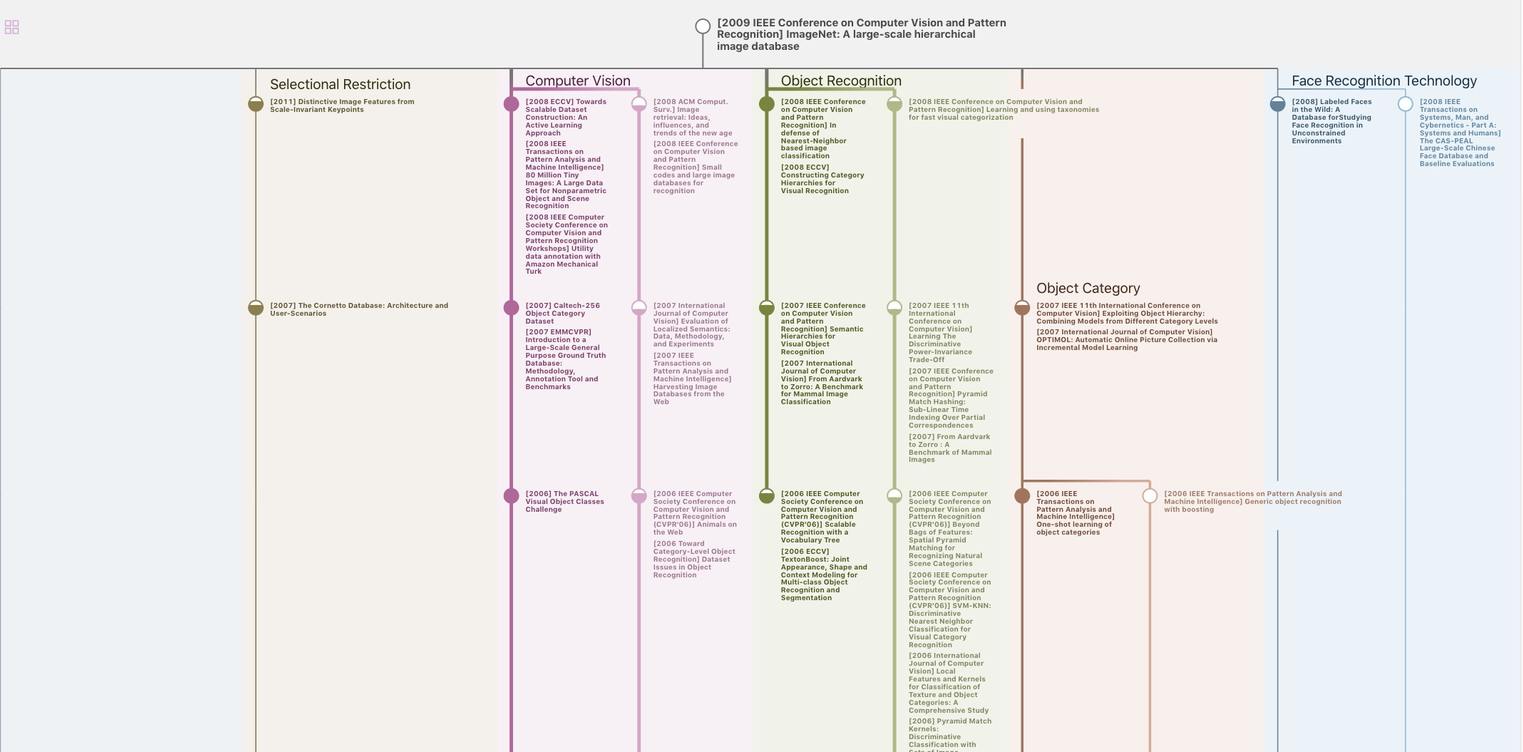
生成溯源树,研究论文发展脉络
Chat Paper
正在生成论文摘要