Effect Of Learning Dataset For Identification Of Active Molecules: A Case Study Of Integrin Alpha Iib Beta 3 Inhibitors
MOLECULAR INFORMATICS(2021)
摘要
Efficient in silico approaches are needed to identify strong integrin alpha IIb beta 3 inhibitors through a small number of measurements. To address the challenge, we investigated the effect of learning dataset on the classification performance of machine learning models focusing on weak and inactive compounds. The structure and activity information of the compounds were obtained from ChEMBL, and pCHEMBL values were used to classify them as active, inactive, or weak. Datasets with various imbalance levels from active:inactive=1 : 1 to 1 : 1000 were used for the machine learning. The prediction scores of the weak samples were found to lie between the predictive values of active and inactive compounds. In addition, another dataset that consists of 149 actives and 6.9 million inactives was screened; the results indicated that the number of positive predictions decreased for models trained with a higher number of inactives. Although there is a trade-off between false positives and false negatives, for determination of compounds with strong activity using a reduced number of measurements, it is better to use a large number of inactives for learning and identifying compounds that score higher than the weak samples.
更多查看译文
关键词
Machine learning, integrin α, IIbβ, 3, in-silico screening
AI 理解论文
溯源树
样例
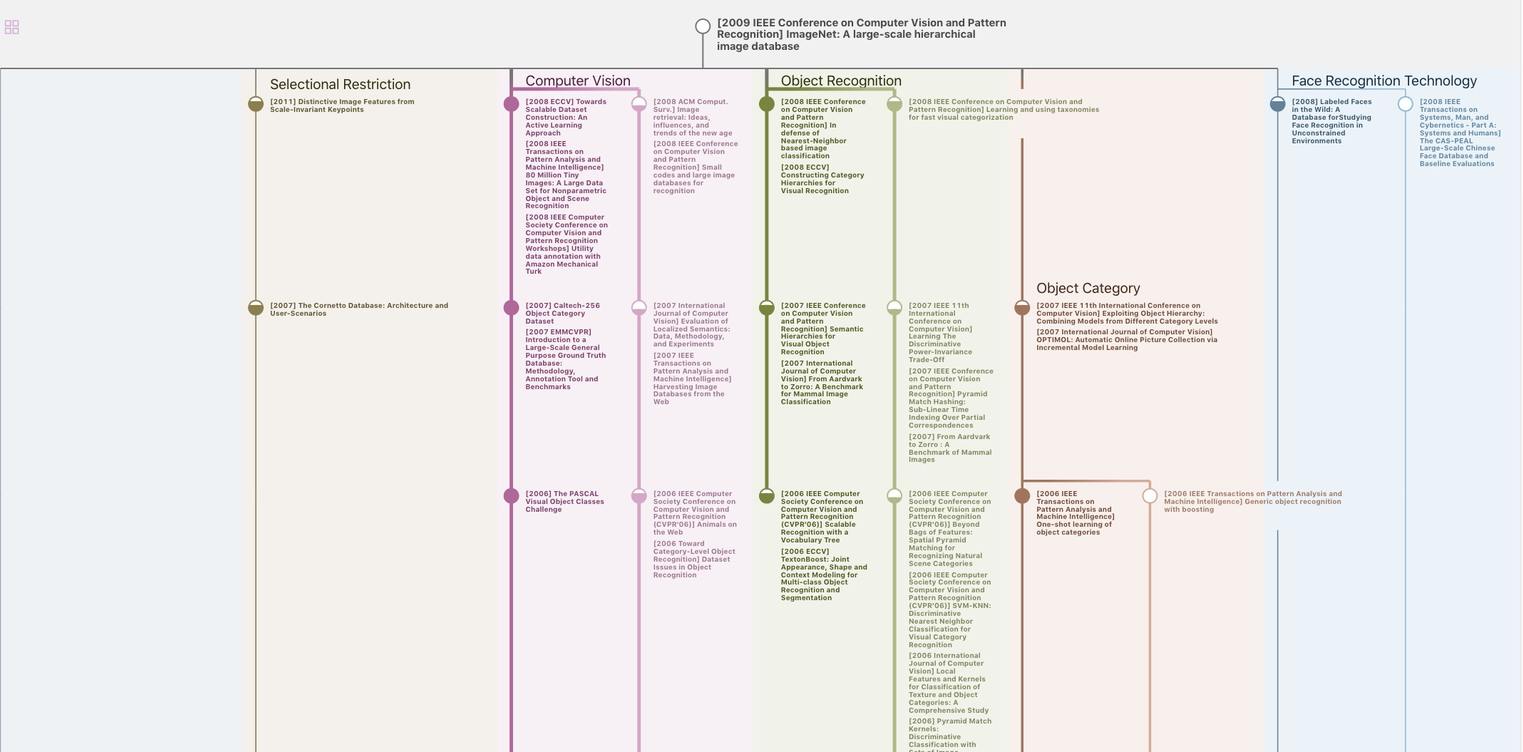
生成溯源树,研究论文发展脉络
Chat Paper
正在生成论文摘要