A time-resolved proteomic and diagnostic map characterizes COVID-19 disease progression and predicts outcome
user-5f8411ab4c775e9685ff56d3(2020)
摘要
Abstract COVID-19 is highly variable in its clinical presentation, ranging from asymptomatic infection to severe organ damage and death. There is an urgent need for predictive markers that can guide clinical decision-making, inform about the effect of experimental therapies, and point to novel therapeutic targets. Here, we characterize the time-dependent progression of COVID-19 through different stages of the disease, by measuring 86 accredited diagnostic parameters and plasma proteomes at 687 sampling points, in a cohort of 139 patients during hospitalization. We report that the time-resolved patient molecular phenotypes reflect an initial spike in the systemic inflammatory response, which is gradually alleviated and followed by a protein signature indicative of tissue repair, metabolic reconstitution and immunomodulation. Further, we show that the early host response is predictive for the disease trajectory and gives rise to proteomic and diagnostic marker signatures that classify the need for supplemental oxygen therapy and mechanical ventilation, and that predict the time to recovery of mildly ill patients. In severely ill patients, the molecular phenotype of the early host response predicts survival, in two independent cohorts and weeks before outcome. We also identify age-specific molecular response to COVID-19, which involves increased inflammation and lipoprotein dysregulation in older patients. Our study provides a deep and time resolved molecular characterization of COVID-19 disease progression, and reports biomarkers for risk-adapted treatment strategies and molecular disease monitoring. Our study demonstrates accurate prognosis of COVID-19 outcome from proteomic signatures recorded weeks earlier.
更多查看译文
关键词
Molecular Response,Biomarker (medicine),Disease,Asymptomatic,Cohort,Inflammation,Oncology,Mechanical ventilation,Phenotype,Medicine,Internal medicine
AI 理解论文
溯源树
样例
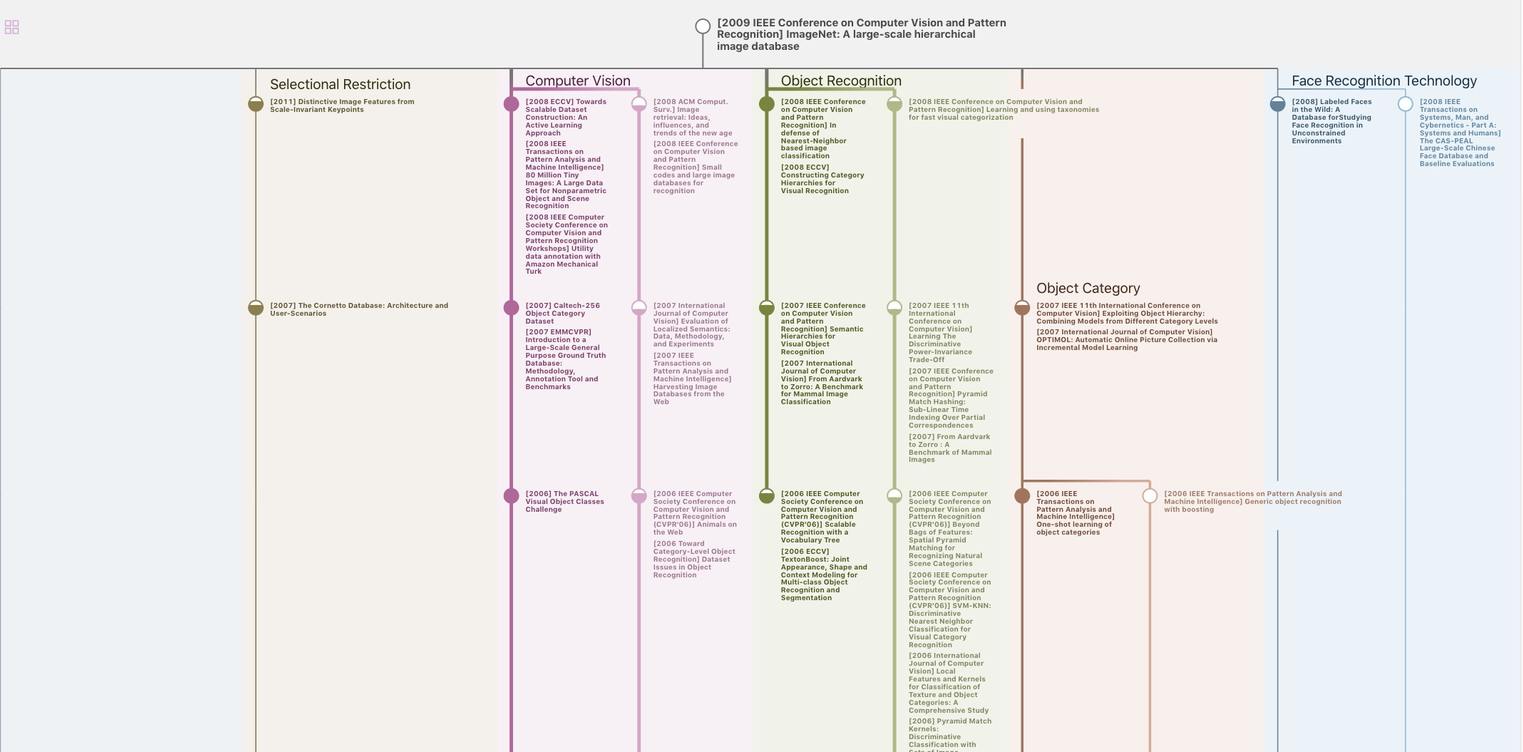
生成溯源树,研究论文发展脉络
Chat Paper
正在生成论文摘要