Controllable Generation from Pre-trained Language Models via Inverse Prompting
Knowledge Discovery and Data Mining(2021)
摘要
ABSTRACTLarge-scale pre-trained language models have demonstrated strong capabilities of generating realistic texts. However, it remains challenging to control the generation results. Previous approaches such as prompting are far from sufficient, and lack of controllability limits the usage of language models. To tackle this challenge, we propose an innovative method, inverse prompting, to better control text generation. The core idea of inverse prompting is to use generated text to inversely predict the prompt during beam search, which enhances the relevance between the prompt and the generated text and thus improves controllability. Empirically, we pre-train a large-scale Chinese language model to perform a systematic study using human evaluation on the tasks of open-domain poem generation and open-domain long-form question answering. Results demonstrate that our proposed method substantially outperforms the baselines and that our generation quality is close to human performance on some of the tasks.
更多查看译文
关键词
Language Modeling, Machine Question Answering, Poem Generation, Controllable Generation, Beam Search
AI 理解论文
溯源树
样例
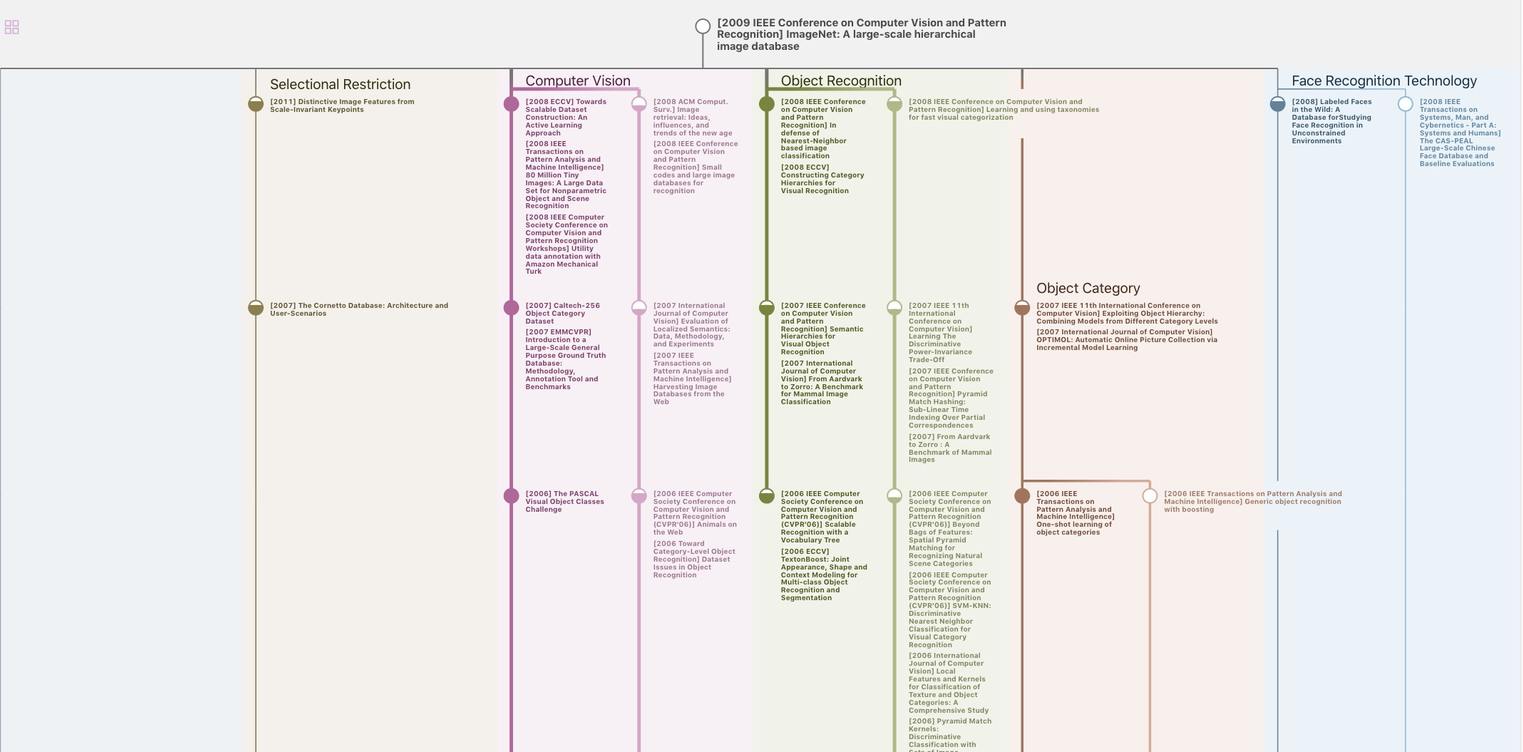
生成溯源树,研究论文发展脉络
Chat Paper
正在生成论文摘要