A First Step Towards Automated Species Recognition from Camera Trap Images of Mammals Using AI in a European Temperate Forest.
CISIM(2021)
摘要
Camera traps are used worldwide to monitor wildlife. Despite the increasing availability of Deep Learning (DL) models, the effective usage of this technology to support wildlife monitoring is limited. This is mainly due to the complexity of DL technology and high computing requirements. This paper presents the implementation of the light-weight and state-of-the-art YOLOv5 architecture for automated labeling of camera trap images of mammals in the Bialowieza Forest (BF), Poland. The camera trapping data were organized and harmonized using TRAPPER software, an open source application for managing large-scale wildlife monitoring projects. The proposed image recognition pipeline achieved an average accuracy of 85% F1-score in the identification of the 12 most commonly occurring medium-size and large mammal species in BF using a limited set of training and testing data (a total 2659 images with animals). Based on the preliminary results, we concluded that the YOLOv5 object detection and classification model is a promising light-weight DL solution after the adoption of transfer learning technique. It can be efficiently plugged in via an API into existing web-based camera trapping data processing platforms such as e.g. TRAPPER system. Since TRAPPER is already used to manage and classify (manually) camera trapping datasets by many research groups in Europe, the implementation of AI-based automated species classification may significantly speed up the data processing workflow and thus better support data-driven wildlife monitoring and conservation. Moreover, YOLOv5 developers perform better performance on edge devices which may open a new chapter in animal population monitoring in real time directly from camera trap devices.
更多查看译文
关键词
species recognition,camera trap images,mammals,temperate forest
AI 理解论文
溯源树
样例
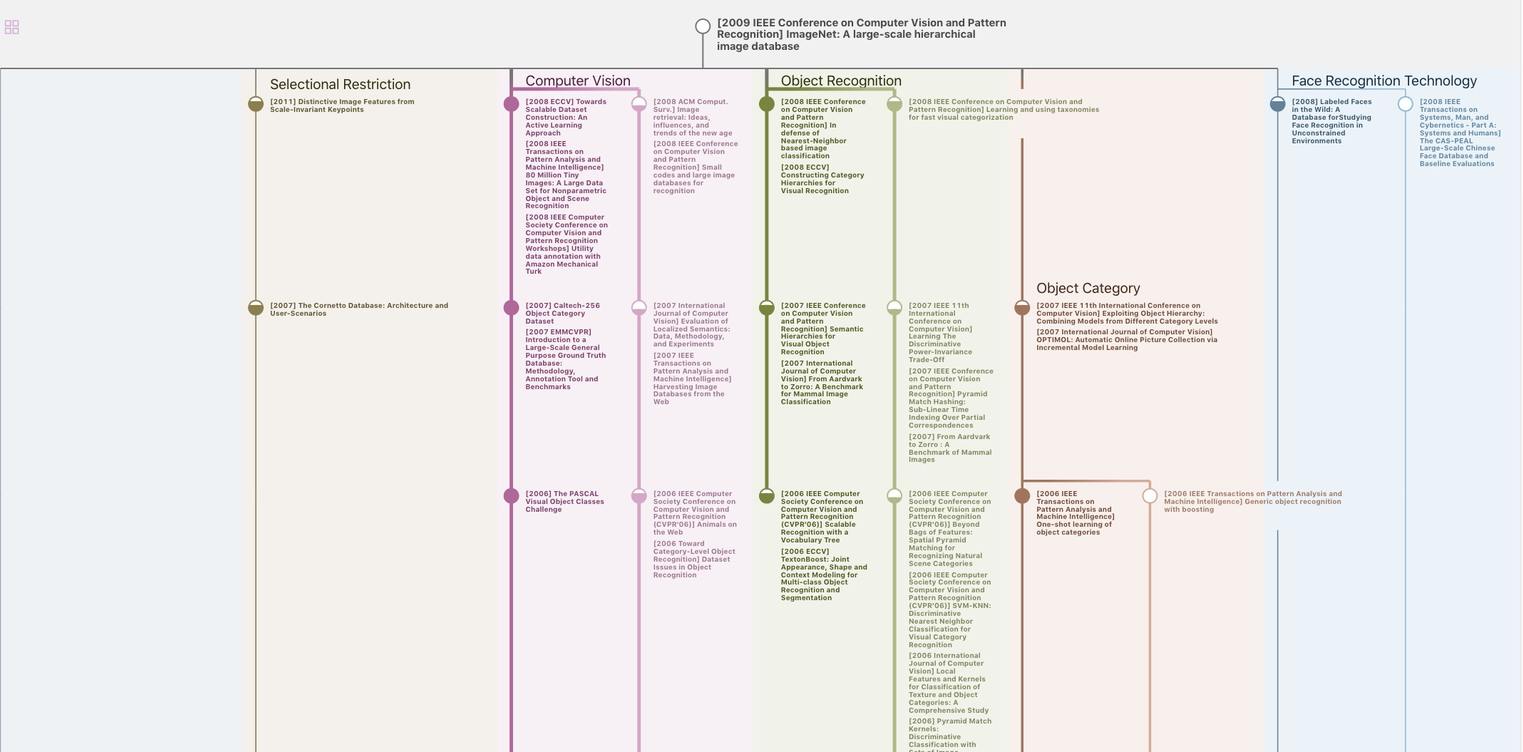
生成溯源树,研究论文发展脉络
Chat Paper
正在生成论文摘要