ActivationNet: Representation Learning to Predict Contact Quality of Interacting 3D Surfaces in Engineering Designs
JOURNAL OF MECHANICAL DESIGN(2022)
摘要
Engineering simulations for analysis of structural and fluid systems require information of contacts between various 3D surfaces of the geometry to accurately model the physics between them. In machine learning applications, 3D surfaces are most suitably represented with point clouds or meshes and learning representations of interacting geometries form point-based representations is challenging. The objective of this study is to introduce a machine learning algorithm, ActivationNet, that can learn from point clouds or meshes of interacting 3D surfaces and predict the quality of contact between these surfaces. The ActivationNet generates activation states from point-based representation of surfaces using a multidimensional binning approach. The activation states are further used to contact quality between surfaces using deep neural networks. The performance of our model is demonstrated using several experiments, and we show that the contact quality predictions of ActivationNet agree well with the expectations.
更多查看译文
关键词
computer-aided design, design evaluation, design representation, machine learning
AI 理解论文
溯源树
样例
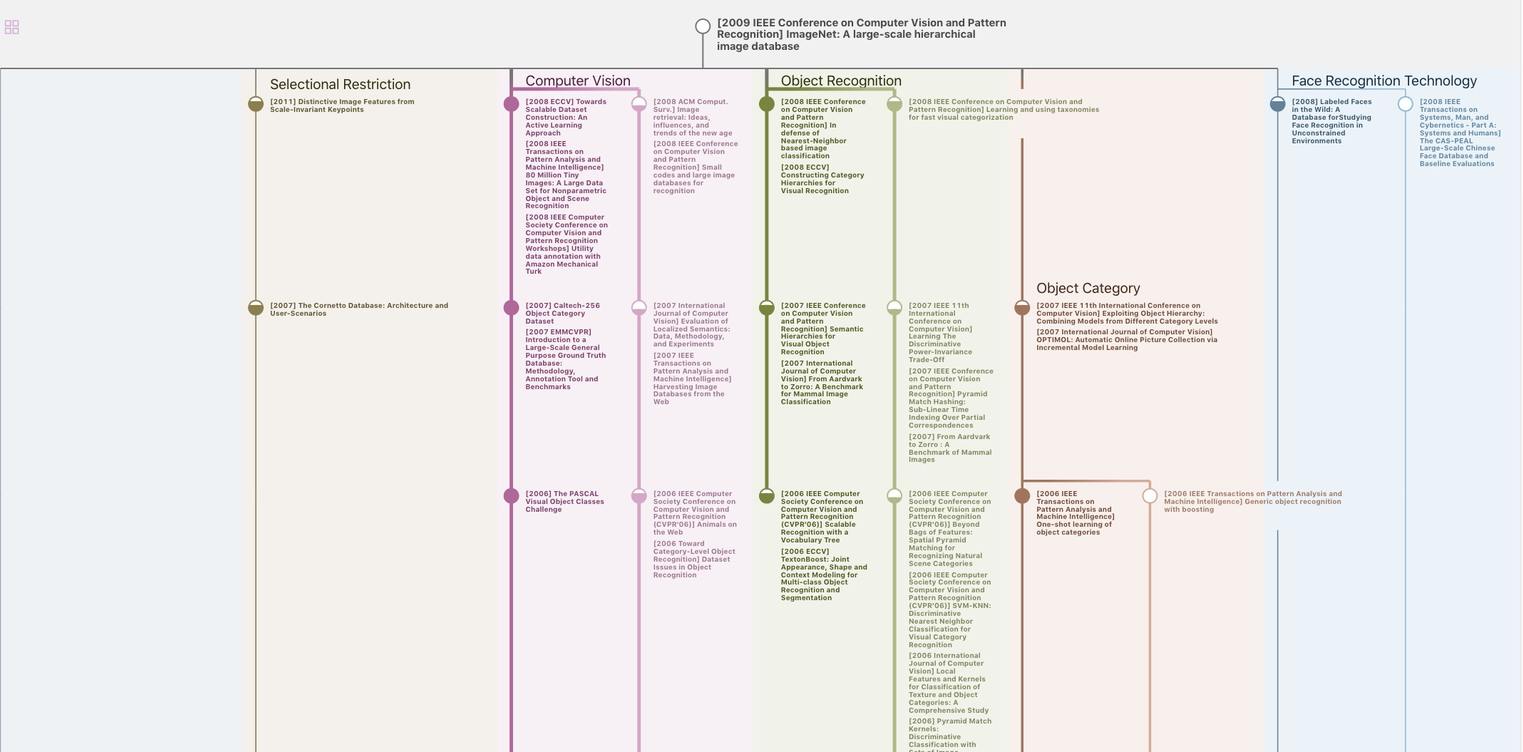
生成溯源树,研究论文发展脉络
Chat Paper
正在生成论文摘要