Supervised Principal Component Regression for Functional Responses with High Dimensional Predictors
JOURNAL OF COMPUTATIONAL AND GRAPHICAL STATISTICS(2024)
摘要
We propose a supervised principal component regression method for relating functional responses with high-dimensional predictors. Unlike the conventional principal component analysis, the proposed method builds on a newly defined expected integrated residual sum of squares, which directly makes use of the association between the functional response and the predictors. Minimizing the integrated residual sum of squares gives the supervised principal components, which is equivalent to solving a sequence of nonconvex generalized Rayleigh quotient optimization problems. We reformulate the nonconvex optimization problems into a simultaneous linear regression with a sparse penalty to deal with high dimensional predictors. Theoretically, we show that the reformulated regression problem can recover the same supervised principal subspace under certain conditions. Statistically, we establish nonasymptotic error bounds for the proposed estimators when the covariate covariance is bandable. We demonstrate the advantages of the proposed method through numerical experiments and an application to the Human Connectome Project fMRI data. Supplementary materials for this article are available online.
更多查看译文
关键词
Functional data,Nonasymptotic error bound,Sparsity,Supervised principal subspace
AI 理解论文
溯源树
样例
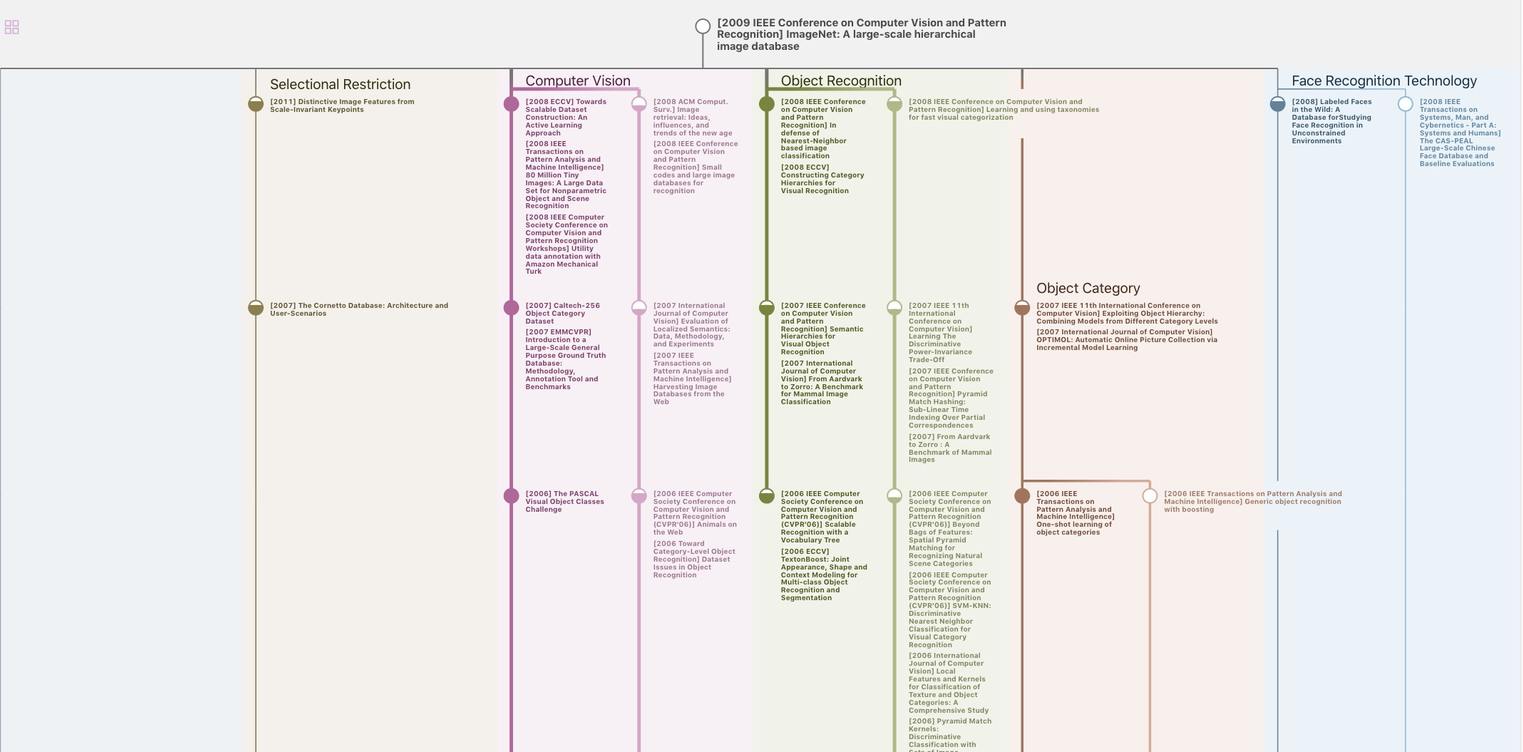
生成溯源树,研究论文发展脉络
Chat Paper
正在生成论文摘要