Deep Neural Networks Learn Meta-Structures to Segment Fluorescence Microscopy Images
arxiv(2021)
摘要
Fluorescence microscopy images play the critical role of capturing spatial or spatiotemporal information of biomedical processes in life sciences. Their simple structures and semantics provide unique advantages in elucidating learning behavior of deep neural networks (DNNs). It is generally assumed that accurate image annotation is required to train DNNs for accurate image segmentation. In this study, however, we find that DNNs trained by label images in which nearly half (49%) of the binary pixel labels are randomly flipped provide largely the same segmentation performance. This suggests that DNNs learn high-level structures rather than pixel-level labels per se to segment fluorescence microscopy images. We refer to these structures as meta-structures. In support of the existence of the meta-structures, when DNNs are trained by a series of label images with progressively less meta-structure information, we find progressive degradation in their segmentation performance. Motivated by the learning behavior of DNNs trained by random labels and the characteristics of meta-structures, we propose an unsupervised segmentation model. Experiments show that it achieves remarkably competitive performance in comparison to supervised segmentation models.
更多查看译文
关键词
segment fluorescence microscopy images,neural networks,meta-structures
AI 理解论文
溯源树
样例
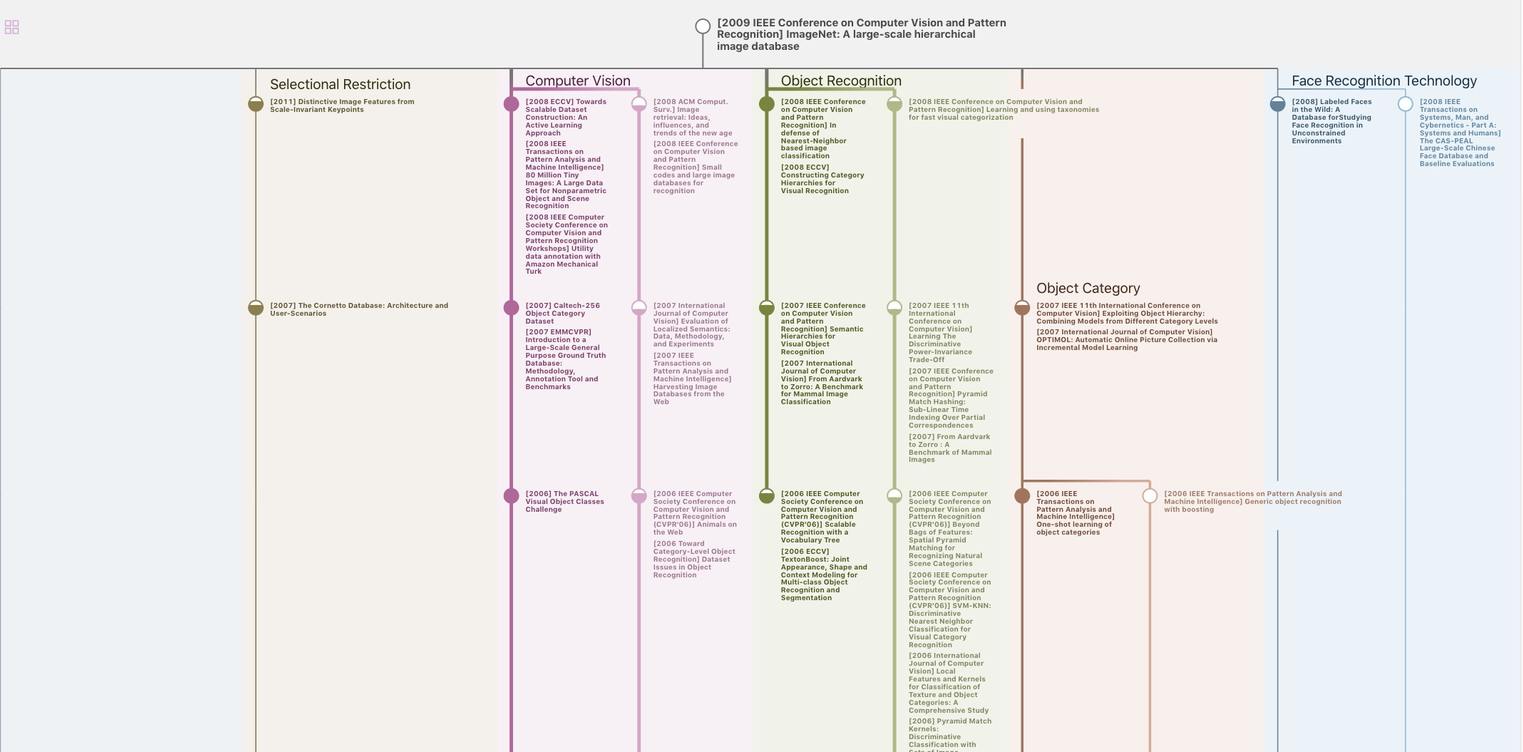
生成溯源树,研究论文发展脉络
Chat Paper
正在生成论文摘要