Fem: Feature Extraction And Mapping For Radio Modulation Classification
PHYSICAL COMMUNICATION(2021)
摘要
Due to the stochastic nature of wireless channels, the received radio signal is noised during transmission causing difficulty in classifying radio modulation categories. Deep learning methods are applied to radio modulation classification for its promising performance compared with traditional approaches. However, deep learning models have relied on a large number of labeled data as training data. Collecting and labeling radio signals in various environments require a lot of manpower and material resources, which will limit the application of deep learning models in modulation recognition. To improve the efficiency of labeling radio signals, in this paper, we propose a feature extraction and mapping algorithm (FEM) to extract and map radio signals to images for further classification. Specifically, we propose an LSTM-based feature extraction network to extract radio features from radio signals, which are further mapped to different categories of images via a feature mapping network. The mapping between radio features and images is automatically learned by a generative adversarial network. Experiment results show that different modulation categories can be obviously discriminated though the mapped images. Furthermore, the modulation classification accuracy based on mapped images is slighted improved comparing with the original radio signals. (C) 2021 Elsevier B.V. All rights reserved.
更多查看译文
关键词
Mapping, Radio signal, Feature extraction, Modulation classification, Deep learning
AI 理解论文
溯源树
样例
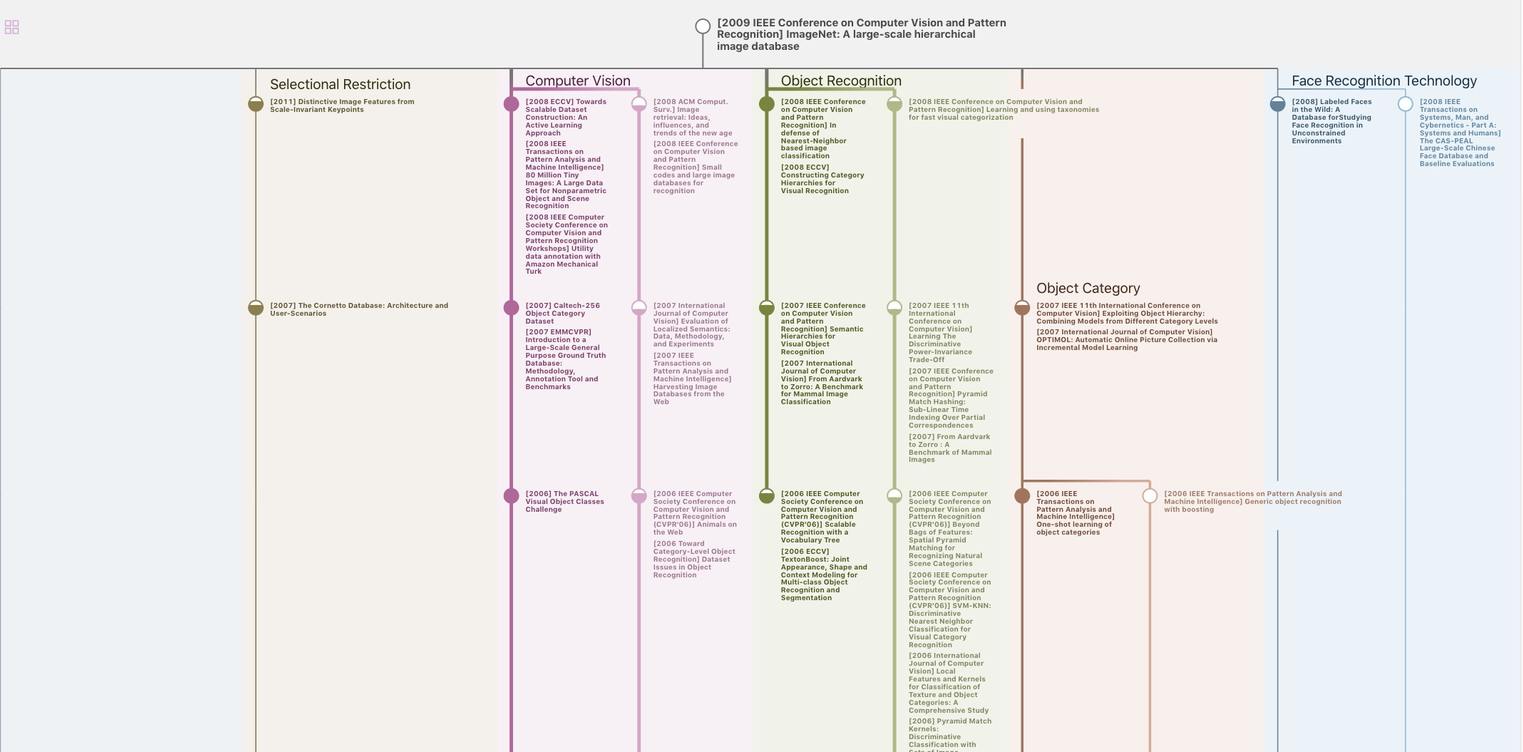
生成溯源树,研究论文发展脉络
Chat Paper
正在生成论文摘要