Scalable Bayesian Modelling For Smoothing Disease Risks In Large Spatial Data Sets Using Inla
SPATIAL STATISTICS(2021)
摘要
Several methods have been proposed in the spatial statistics literature to analyse big data sets in continuous domains. However, new methods for analysing high-dimensional areal data are still scarce. Here, we propose a scalable Bayesian modelling approach for smoothing mortality (or incidence) risks in high-dimensional data, that is, when the number of small areas is very large. The method is implemented in the R add-on package bigDM and it is based on the idea of "divide and conquer". Although this proposal could possibly be implemented using any Bayesian fitting technique, we use INLA here (integrated nested Laplace approximations) as it is now a well-known technique, computationally efficient, and easy for practitioners to handle. We analyse the proposal's empirical performance in a comprehensive simulation study that considers two model-free settings. Finally, the methodology is applied to analyse male colorectal cancer mortality in Spanish municipalities showing its benefits with regard to the standard approach in terms of goodness of fit and computational time. (C) 2021 Elsevier B.V. All rights reserved.
更多查看译文
关键词
High-dimensional data, Hierarchical models, Mixture models, Spatial epidemiology
AI 理解论文
溯源树
样例
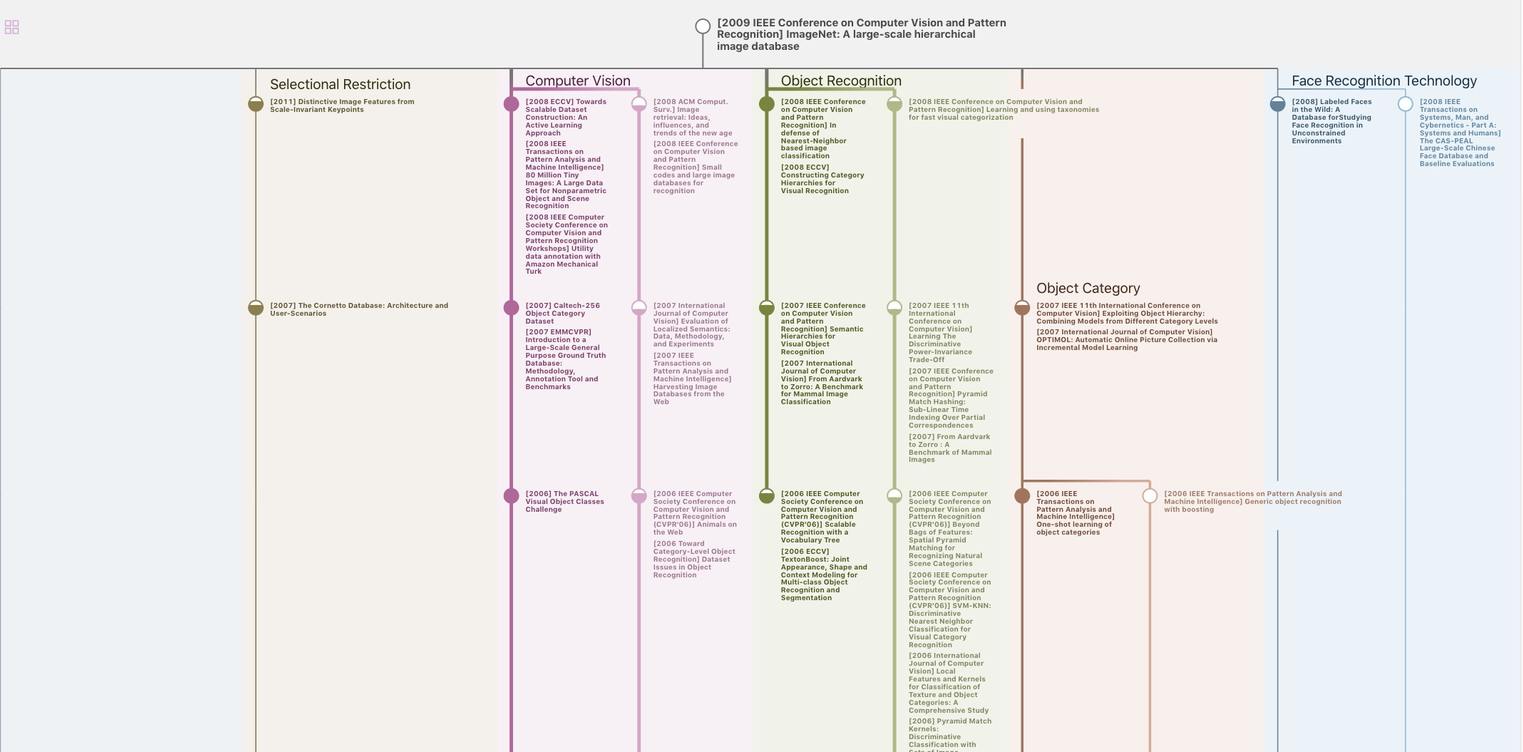
生成溯源树,研究论文发展脉络
Chat Paper
正在生成论文摘要