New Approach For Predicting Nitrification And Its Fraction Of N2o Emissions In Global Terrestrial Ecosystems
ENVIRONMENTAL RESEARCH LETTERS(2021)
摘要
Nitrification is a major pathway of N2O production in aerobic soils. Measurements and model simulations of nitrification and associated N2O emission are challenging. Here we innovatively integrated data mining and machine learning to predict nitrification rate (R-nit) and the fraction of nitrification as N2O emissions (f(N2ONit)). Using our global database on R-nit and fN(2)ONit, we found that the machine-learning based stochastic gradient boosting (SGB) model outperformed three widely used process-based models in estimating R-nit and N2O emission from nitrification. We then applied the SGB technique for global prediction. The potential R-nit was driven by long-term mean annual temperature, soil C/N ratio and soil pH, whereas f(N2ONi)t by mean annual precipitation, soil clay content, soil pH, soil total N. The global f(N2ONit) varied by over 200 times (0.006%-1.2%), which challenges the common practice of using a constant value in process-based models. This study provides insights into advancing process-based models for projecting N dynamics and greenhouse gas emissions using a machine learning approach.
更多查看译文
关键词
gross nitrification rate, N2O from nitrification, machine learning, nitrogen cycle, climate change, modelling
AI 理解论文
溯源树
样例
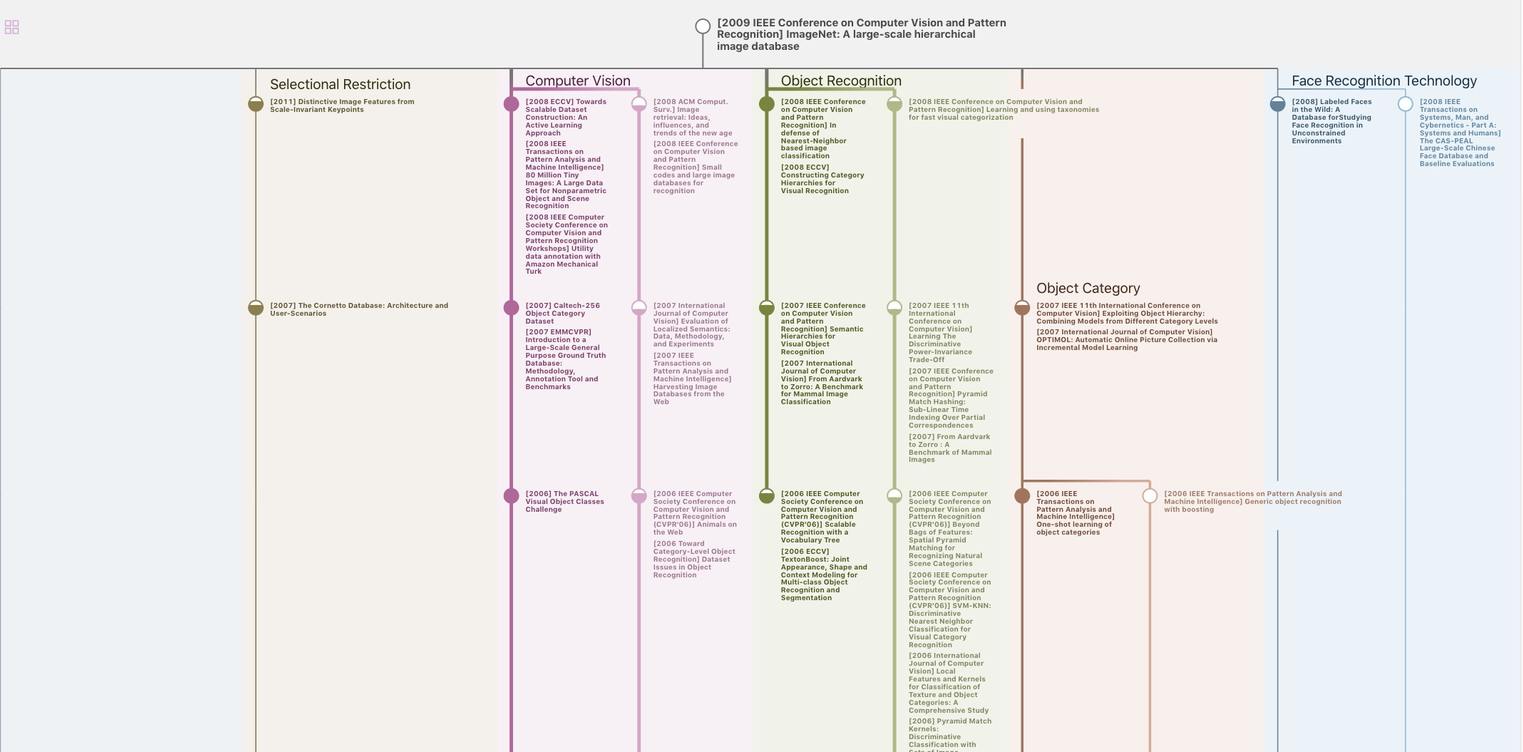
生成溯源树,研究论文发展脉络
Chat Paper
正在生成论文摘要