A deep learning approach for real-time rebar counting on the construction site based on YOLOv3 detector
AUTOMATION IN CONSTRUCTION(2021)
摘要
Counting steel bars is a routine daily task for steel manufacturers and most building construction sites. Currently, counting is usually performed manually, which is laborious, time-consuming, and error-prone. This study proposes a deep learning approach based on YOLOv3 detector for automatic steel bars detection and counting through images. Three new measures, including an additional feature pyramid, complete intersection over union (IoU) loss and focal loss, and bag of freebies, were introduced to improve rebar detection and counting performance. A dataset containing 74,824 rebar sections from real construction sites was constructed and utilized to evaluate the proposed approach. The application results demonstrate that the suggested measures can significantly improve the performance of the YOLOv3 detector to high average precision of 99.7% at IoU of 0.5. Comparisons with other detectors show that the proposed approach is fast, accurate, and robust at rebar counting under different construction site conditions. It can be facilitated as the basis of a real-time, cost-effective rebar counting scheme.
更多查看译文
关键词
Rebar counting,Construction sites,YOLOv3,Deep learning,Civil engineering
AI 理解论文
溯源树
样例
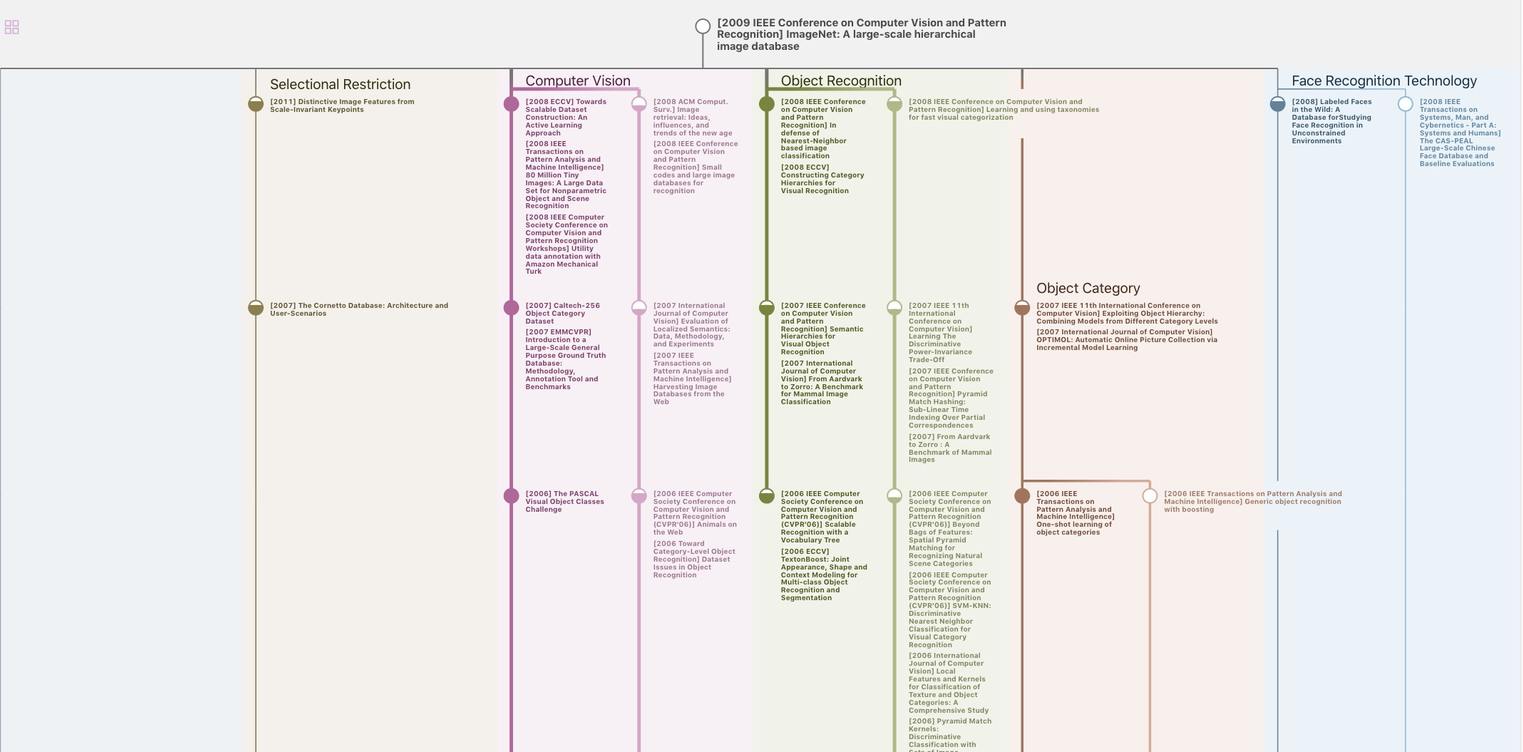
生成溯源树,研究论文发展脉络
Chat Paper
正在生成论文摘要