Solving a Joint Pricing and Inventory Control Problem for Perishables Via Deep Reinforcement Learning
Complexity(2021)
摘要
We study a joint pricing and inventory control problem for perishables with positive lead time in a finite horizon periodic-review system. Unlike most studies considering a continuous density function of demand, in our paper the customer demand depends on the price of current period and arrives according to a homogeneous Poisson process. We consider both backlogging and lost-sales cases, and our goal is to find a simultaneously ordering and pricing policy to maximize the expected discounted profit over the planning horizon. When there is no fixed ordering cost involved, we design a deep reinforcement learning algorithm to obtain a near-optimal ordering policy and show that there are some monotonicity properties in the learned policy. We also show that our deep reinforcement learning algorithm achieves a better performance than tabular-based Q-learning algorithms. When a fixed ordering cost is involved, we show that our deep reinforcement learning algorithm is effective and efficient, under which the problem of “curse of dimension” is circumvented.
更多查看译文
AI 理解论文
溯源树
样例
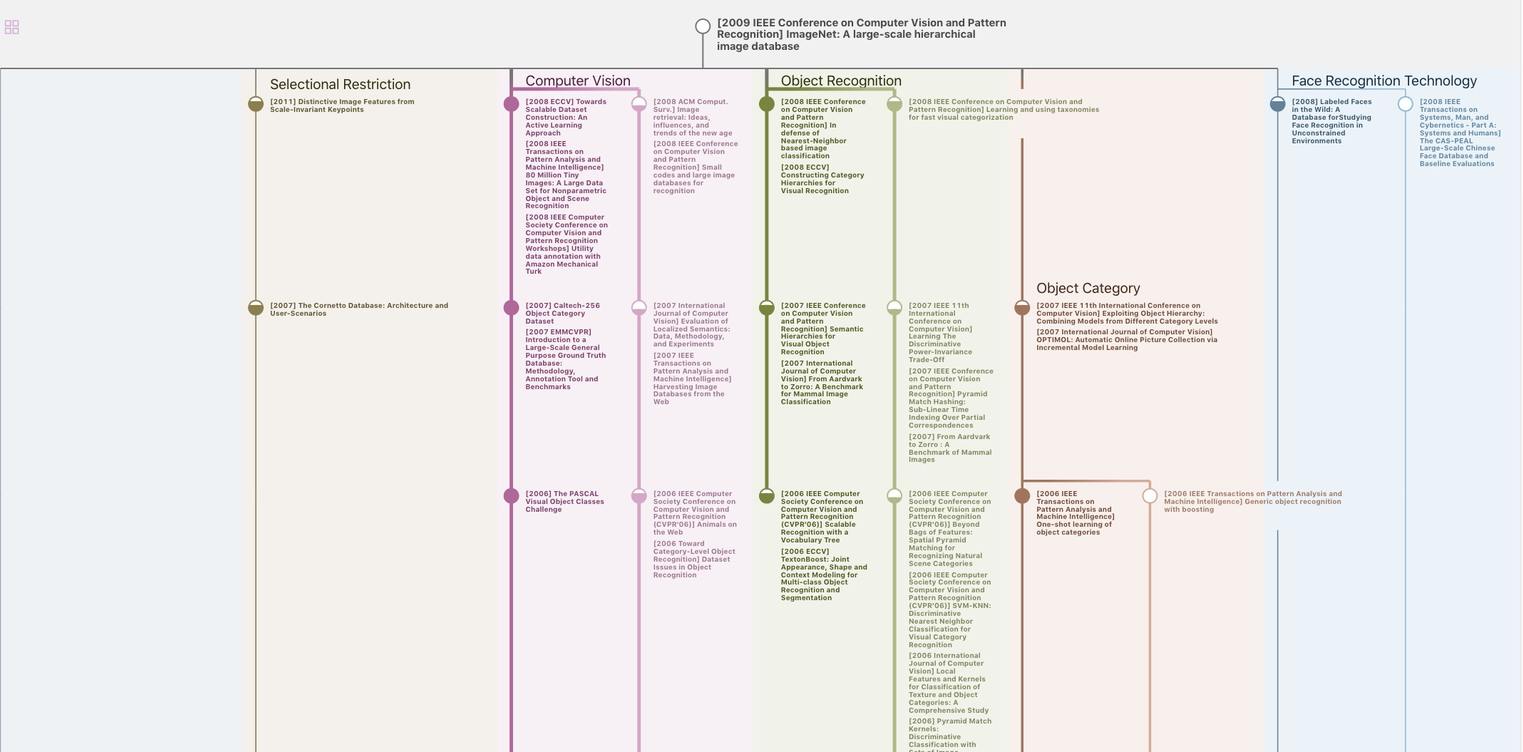
生成溯源树,研究论文发展脉络
Chat Paper
正在生成论文摘要