Multi-attention DeepEMD for Few-Shot Learning in Remote Sensing
ieee joint international information technology and artificial intelligence conference(2020)
摘要
Currently in the field of remote sensing (RS), scene classification is a crucial research subject. The usual method is to gather plenty of scene classification data manually annotated by experts in the field, and then use the dataset to train a scene classification model. Typically, the better the deep learning model requires more labeled data. Therefore, how to obtain a model with good classification performance when there is only a small amount of labeled data, this becomes a well-known few-shot classification problem. In this study, a new few-shot learning scheme is developed for RS scene classification. Our method is based on deepEMD with spatial and channel attention modules(CBAM) to capture subtle but distinguishing features. To get the significant weights of pixels in the EMD formulation, an attention-reference mechanism is designed, which can sufficiently leverage subtle local features and minimize the influence posed by the cluttered background. To avoid overfitting, label smoothing that can improve the generalization performance of the model is introduced. Comprehensive experiments are conducted to validate the method and achieve new state-of-the-art performance on three popular RS scene datasets, namely UC Merced, AID and OPTIMAL-31. Furthermore, two ablative studies also indicate that the components are conducive to fast convergence and high accuracy.
更多查看译文
关键词
remote sensing,scene classification,few-shot learning,attention mechanism,deepEMD
AI 理解论文
溯源树
样例
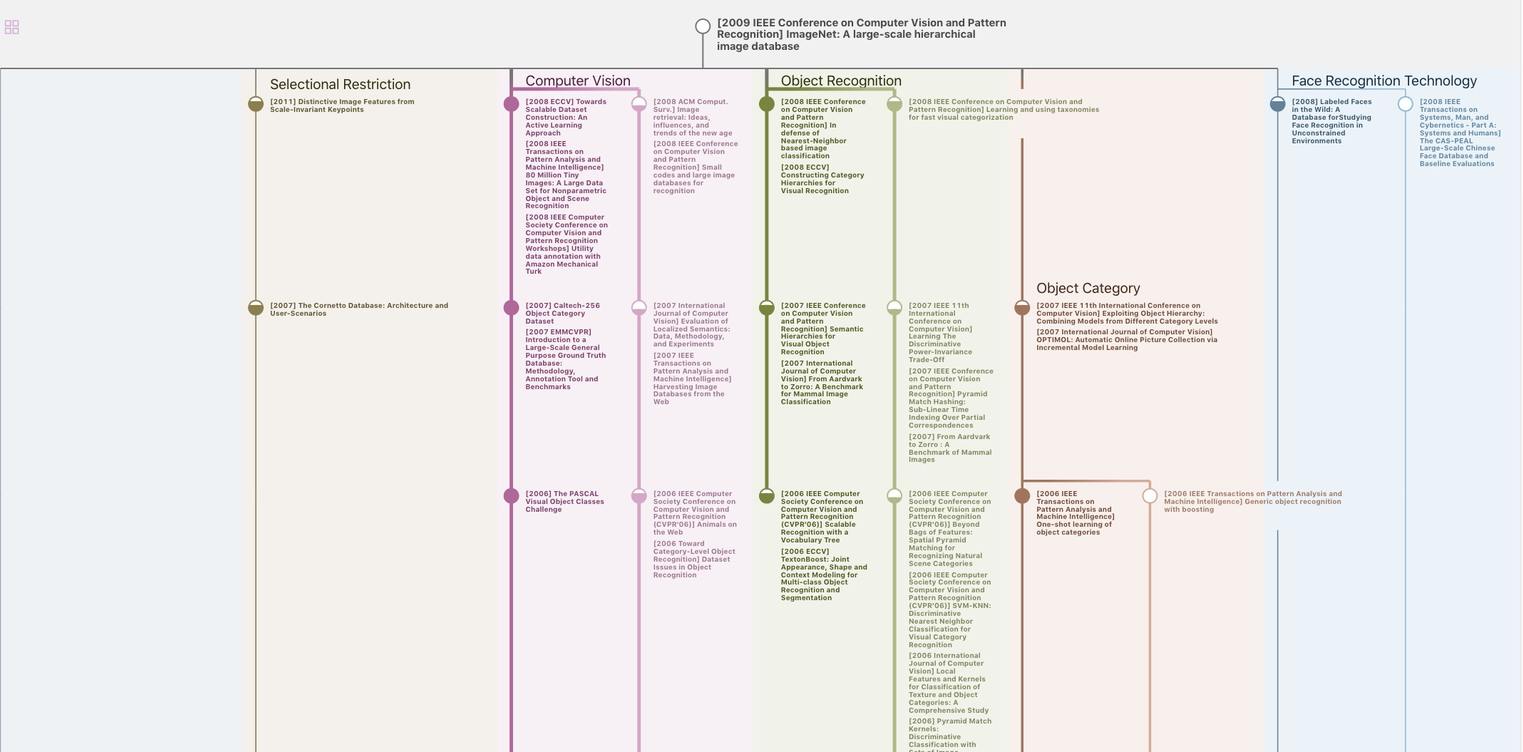
生成溯源树,研究论文发展脉络
Chat Paper
正在生成论文摘要