PRIMA++: A Probabilistic Framework for User Choice Modelling With Small Data
IEEE Transactions on Signal Processing(2021)
摘要
We face choice problems every day, where we have to choose from options with multiple conflicting attributes, and learning a user's personal preference in choice problems is particularly important. For example, in E-commerce applications, this study may help make personal recommendations and sales prediction, and may offer important guidelines on sellers' pricing and discount strategies. Prior works either do not consider the competition among different options, or cannot handle the convex hull problem where a user selects an option that is regarded as unlikely to be the user's best choice by the models. Those supervised learning methods assume that a huge amount of data is available for training, while in reality, we may only have just a few records. In this work, we propose PRIMA++, a probabilistic framework that jointly considers the inter-attribute tradeoff and the inter-item competition, and a novel method to learn the user's personal preference from just a few past records. It can effectively address the convex hull problem and significantly improves the performance. We also borrow the concept of indifference curve from microeconomics and theoretically analyze a user's decision-making process and the competition among different available options. Real user test results show that our proposed framework can learn the user's preference from just a few records and achieves better performance than state-of-the-art works, especially when the data available for training are limited.
更多查看译文
关键词
Choice modelling,preference learning,probabilistic ranking
AI 理解论文
溯源树
样例
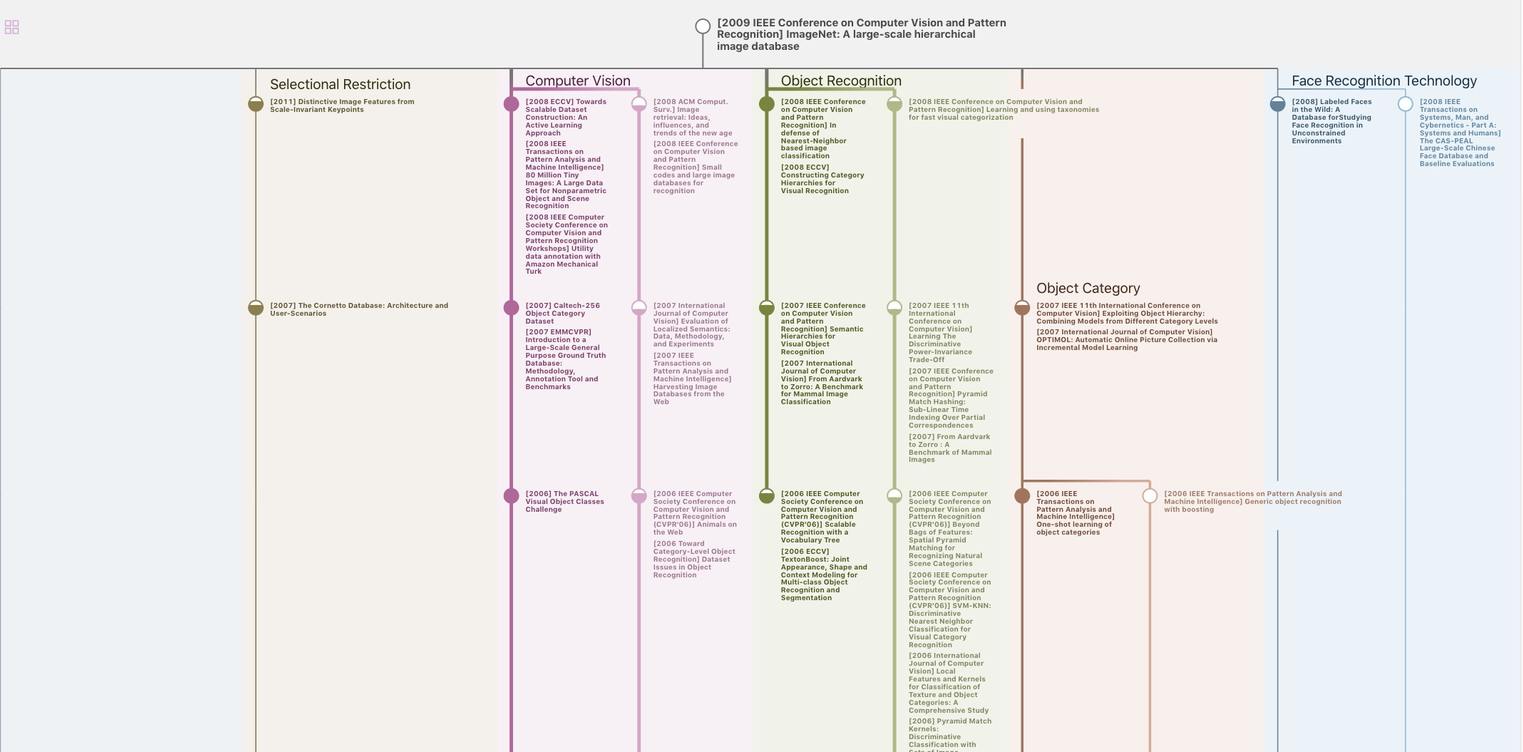
生成溯源树,研究论文发展脉络
Chat Paper
正在生成论文摘要