Short-Term Traffic Flow Prediction: An Integrated Method of Econometrics and Hybrid Deep Learning
IEEE Transactions on Intelligent Transportation Systems(2022)
摘要
This study proposes a short-term traffic flow prediction framework. The vector autoregression (VAR) model based on econometric theory and the CNN-LSTM hybrid neural network model based on deep learning are employed in the analysis. An intrinsic association among traffic variables is first evaluated using the VAR model, and the predictable relationship of these variables is determined. Then the multi-features speed prediction for one spatial location using the CNN-LSTM hybrid neural network model is conducted, the prediction results prove that prediction with multi-feature is better than that with a single feature. Subsequently, several popular deep learning models and other shallow predicted models are proposed to be compared with the constructed CNN-LSTM network model, and the comparison illustrates that the model performance of the developed CNN-LSTM network model is superior to other models in forecasting the short-term traffic flow. Then the multi-feature speed predictions for a group of spatial locations are further conducted using the CNN-LSTM model. The result demonstrates the predictive accuracies are associated with the spatial correlation of traffic flow. Finally, a heatmap is produced to visualize the predicted speed, from which the spatial-temporal traffic condition can be presented clearly. The research results have the potential to be applied to the travel information releasing and traffic congestion management.
更多查看译文
关键词
Short-term traffic flow,vector autoregression,CNN-LSTM hybrid neural network,multi-feature,spatiotemporal heatmap
AI 理解论文
溯源树
样例
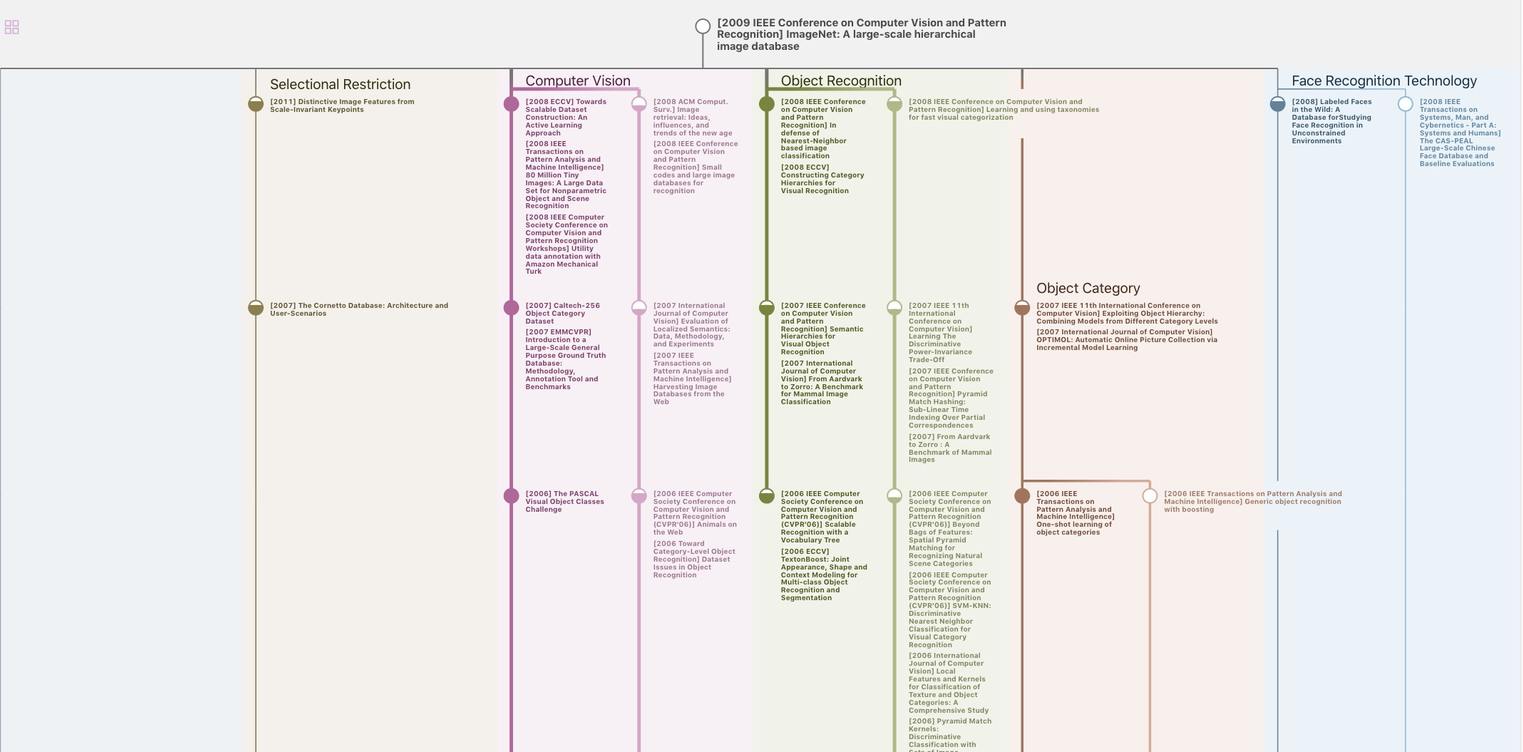
生成溯源树,研究论文发展脉络
Chat Paper
正在生成论文摘要