ULPT: A User-Centric Location Privacy Trading Framework for Mobile Crowd Sensing
IEEE Transactions on Mobile Computing(2022)
摘要
Mobile crowd sensing (MCS) arises as a promising data collection paradigm that leverages the power of ubiquitous mobile devices to acquire rich information regarding their surrounding environment. In many location-based sensing tasks, workers are required to associate their sensing reports with corresponding geographic coordinates. Such information leaves a trail of worker's historical location record which thus poses a severe threat to their location privacy. On the other hand, individual workers may perceive location privacy differently. Instead of following conventional solutions that aim to perfectly hide user privacy, this paper adopts a novel alternative approach. A
u
ser-centric
l
ocation
p
rivacy
t
rading framework, called ULPT, is constructed to facilitate location privacy trading between workers and the platform. Each worker can decide how much location privacy to disclose to the platform in an MCS task based on its own location privacy leakage budget
$\xi$
. The higher
$\xi$
is, the more privacy its reported location discloses. Accordingly, it receives higher payment from the platform as compensation. Besides, ULPT enables the platform to select a suitable set of winning workers to achieve desirable MCS service accuracy while taking into account of its budget limit and worker privacy requirements. For this purpose, a heuristic algorithm is devised with a bounded optimality gap. As formally proved in this manuscript, ULPT guarantees a series of nice properties, including
$\xi$
-
privacy
,
$(\alpha, \beta)$
-
accuracy
,
budget feasibility
. Moreover, both rigorous theoretical analysis and extensive simulations are conducted to evaluate tradeoffs among these three.
更多查看译文
关键词
Location privacy,mobile crowd sensing,privacy trading
AI 理解论文
溯源树
样例
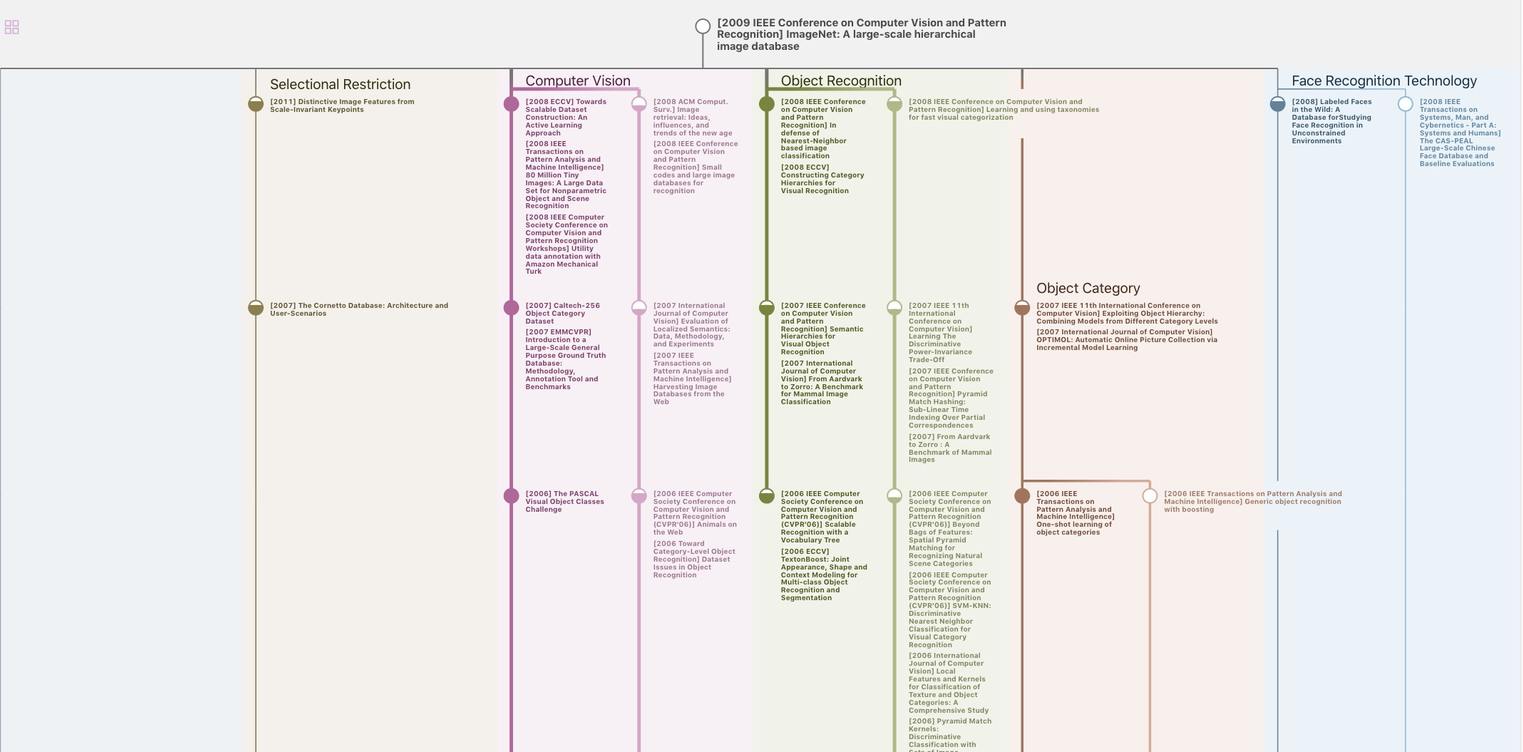
生成溯源树,研究论文发展脉络
Chat Paper
正在生成论文摘要