Improving the accuracy of energy predictive models for multicore CPUs by combining utilization and performance events model variables
Journal of Parallel and Distributed Computing(2021)
摘要
Energy predictive modeling is the leading method for determining the energy consumption of an application. Performance monitoring counters (PMCs) and resource utilizations have been the principal source of model variables primarily due to their high positive correlation with energy consumption. Performance events, however, have come to dominate the landscape due to their better prediction accuracy compared to utilization variables. Recently, the theory of energy of computing has been proposed whose practical implications for constructing accurate and reliable linear energy predictive models are unified in a consistency test that includes a selection criterion of additivity for model variables. In this work, we analyze the prediction accuracy of models employing utilization variables only, PMCs only, and combination of both utilization variables and PMCs, through the lens of this theory for modern multicore CPU platforms. We discover that employing utilization variables only in linear energy predictive models does not capture all the energy-consuming activities during an application execution. However, combination of utilization variables with PMCs that are highly additive and highly correlated with energy consumption, gives the most accurate linear energy predictive model. Our experimental results show that application-specific and platform-level models using both utilization variables and PMCs exhibit up to 3.6× and 2.6× better average prediction accuracy respectively when compared with models employing utilization variables only and highly additive PMCs only.
更多查看译文
关键词
Performance monitoring counters,CPU utilization,Energy modeling,Energy predictive models,Multicore CPU
AI 理解论文
溯源树
样例
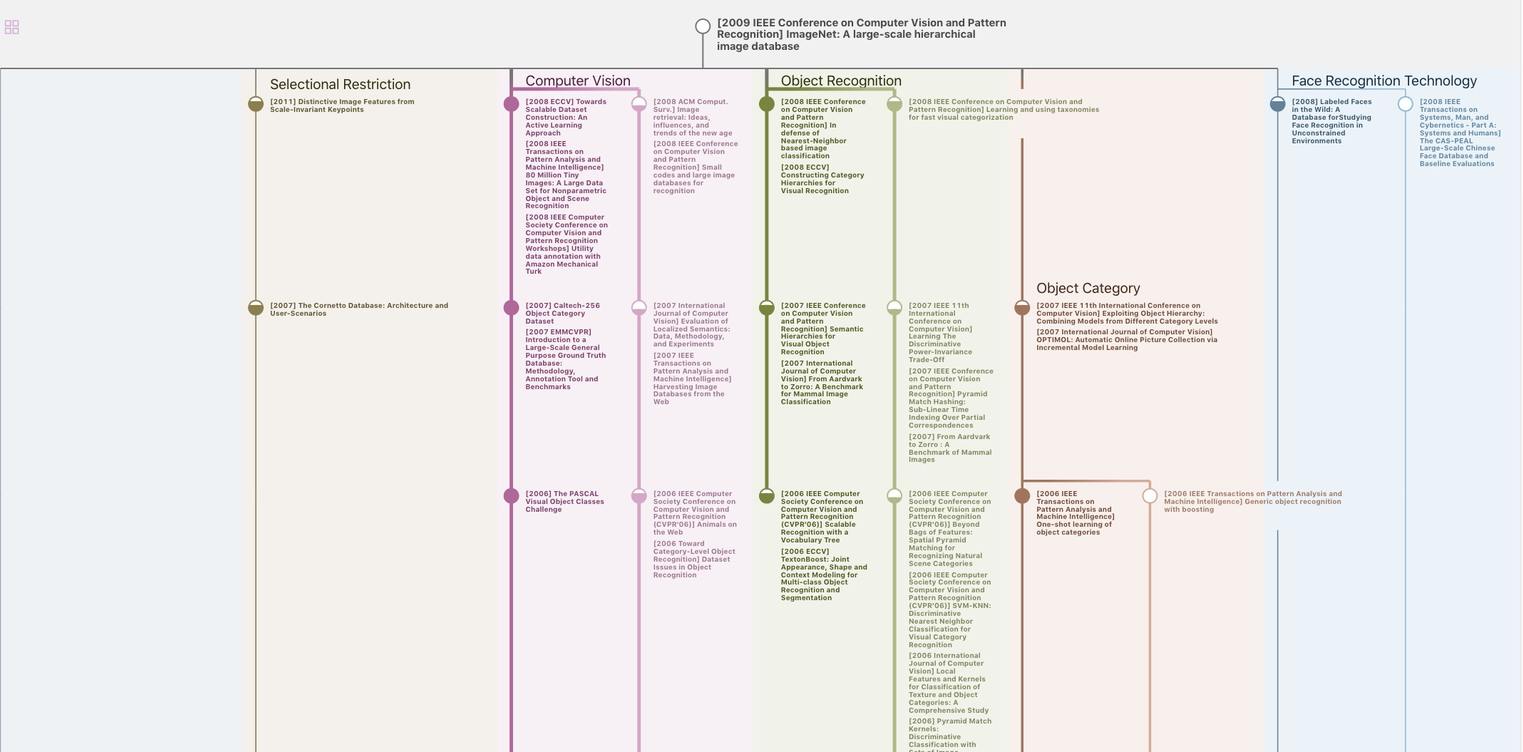
生成溯源树,研究论文发展脉络
Chat Paper
正在生成论文摘要