Excellent Pattern Recognition Accuracy of Neural Networks Using Hybrid Synapses and Complementary Training
IEEE Electron Device Letters(2021)
摘要
To overcome the performance degradation in hardware neural networks (NNs) with non-ideal synapse devices, we proposed a novel neuromorphic architecture with both TiO
x
-based interfacial RRAM and CBRAM-based filamentary RRAM for highly accurate NN training and long-term inference reliability. We used a threshold-triggered training scheme, in which interfacial and filamentary RRAMs were programmed in a complementary fashion. This took advantage of the long retention time of the filamentary RRAM and the high-resolution, symmetric weight update in the interfacial RRAM. Additional evaluation of device parameters, such as linearity, precision, variation, and retention time, was conducted. An excellent pattern recognition accuracy of ~97% was achieved during training with the MNIST dataset. Thus, reliable inference accuracy after training was maintained using the filamentary RRAM.
更多查看译文
关键词
Crossbar array,neural networks,synapse device
AI 理解论文
溯源树
样例
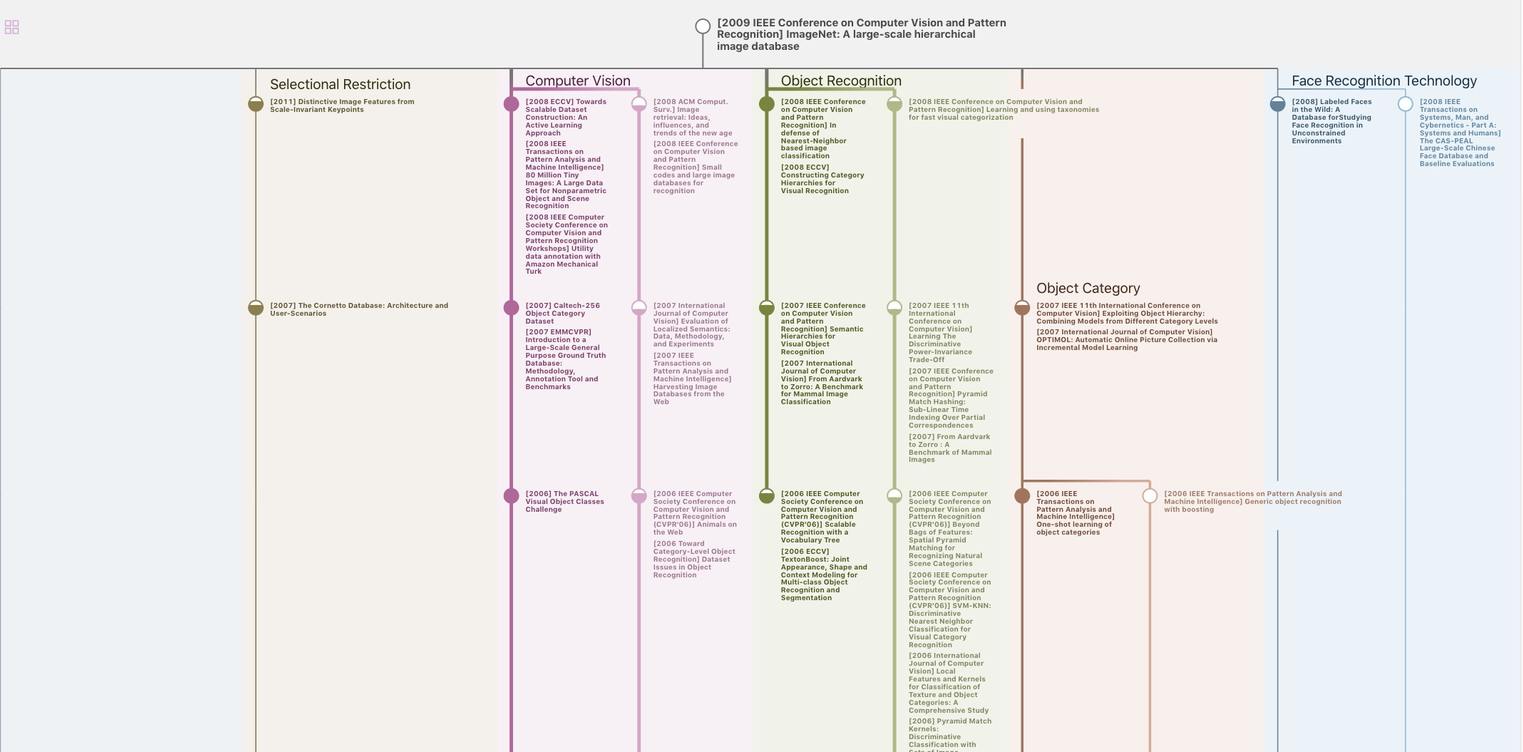
生成溯源树,研究论文发展脉络
Chat Paper
正在生成论文摘要