The NLMS Is Steady-State Schur-Convex
IEEE Signal Processing Letters(2021)
摘要
In this work, we study the impact of input-spread on the steady-state excess mean squared error (EMSE) of the normalized least mean squares (NLMS) algorithm. First, we use the concept of majorization to order the input-regressors according to their spread. Second, we use Schur-convexity to show that the majorization order of the input-regressors is preserved in the EMSE. Effectively, we provide an analytical justification of the increase in steady-state EMSE as the input-spread increases.
更多查看译文
关键词
Adaptive filters,input-spread,majorization,mean squared error,Schur-convexity
AI 理解论文
溯源树
样例
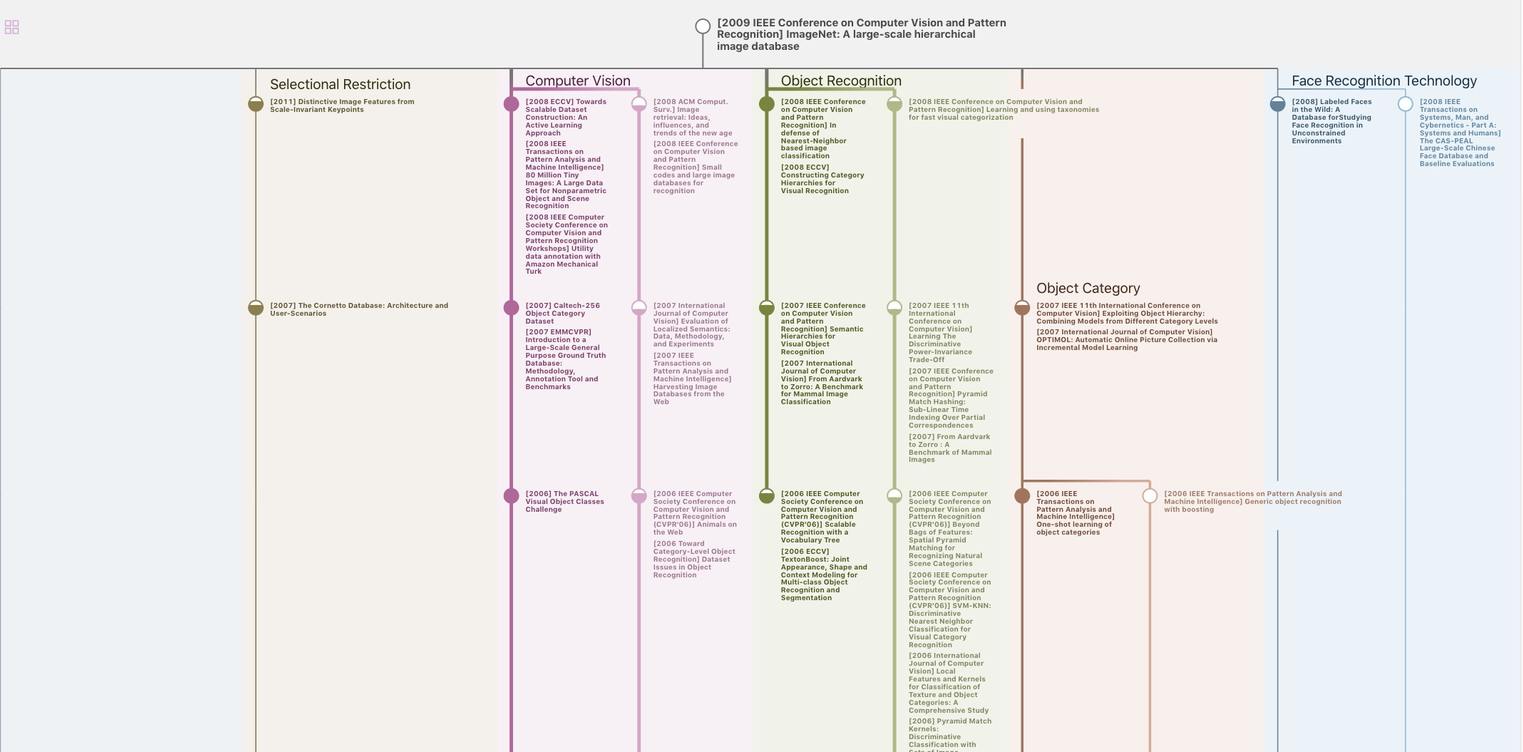
生成溯源树,研究论文发展脉络
Chat Paper
正在生成论文摘要