Dimension reduction for covariates in network data
BIOMETRIKA(2022)
摘要
A problem of major interest in network data analysis is to explain the strength of connections using context information. To achieve this, we introduce a novel approach, called network-supervised dimension reduction, in which covariates are projected onto low-dimensional spaces to reveal the linkage pattern without assuming a model. We propose a new loss function for estimating the parameters in the resulting linear projection, based on the notion that closer proximity in the low-dimension projection corresponds to stronger connections. Interestingly, the convergence rate of our estimator is found to depend on a network effect factor, which is the smallest number that can partition a graph in a manner similar to the graph colouring problem. Our method has interesting connections to principal component analysis and linear discriminant analysis, which we exploit for clustering and community detection. The proposed approach is further illustrated by numerical experiments and analysis of a pulsar candidates dataset from astronomy.
更多查看译文
关键词
Clustering, Community detection, Dimension reduction, Graph, Network
AI 理解论文
溯源树
样例
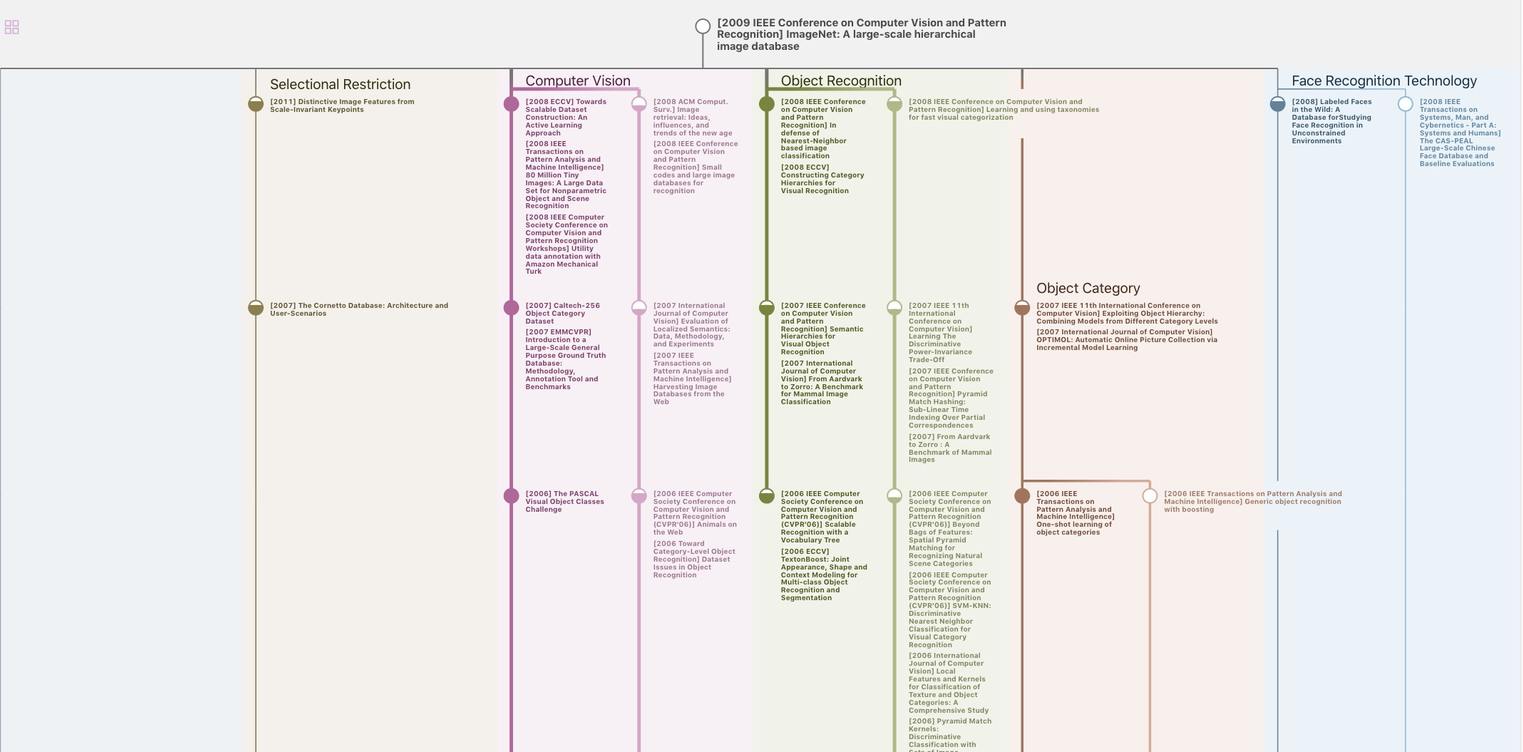
生成溯源树,研究论文发展脉络
Chat Paper
正在生成论文摘要