Unsupervised Gaze: Exploration of Geometric Constraints for 3D Gaze Estimation
MMM (2)(2021)
Abstract
Eye gaze estimation can provide critical evidence for people attention, which has extensive applications on cognitive science and computer vision areas, such as human behavior analysis and fake user identification. Existing typical methods mostly place the eye-tracking sensors directly in front of the eyeballs, which is hard to be utilized in the wild. And recent learning-based methods require prior ground truth annotations of gaze vector for training. In this paper, we propose an unsupervised learning-based method for estimating the eye gaze in 3D space. Building on top of the existing unsupervised approach to regress shape parameters and initialize the depth, we propose to apply geometric spectral photometric consistency constraint and spatial consistency constraints across multiple views in video sequences to refine the initial depth values on the detected iris landmark. We demonstrate that our method is able to learn gaze vector in the wild scenes more robust without ground truth gaze annotations or 3D supervision, and show our system leads to a competitive performance compared with existing supervised methods.
MoreTranslated text
Key words
Unsupervised learning,3D gaze estimation,Geometric constraints
AI Read Science
Must-Reading Tree
Example
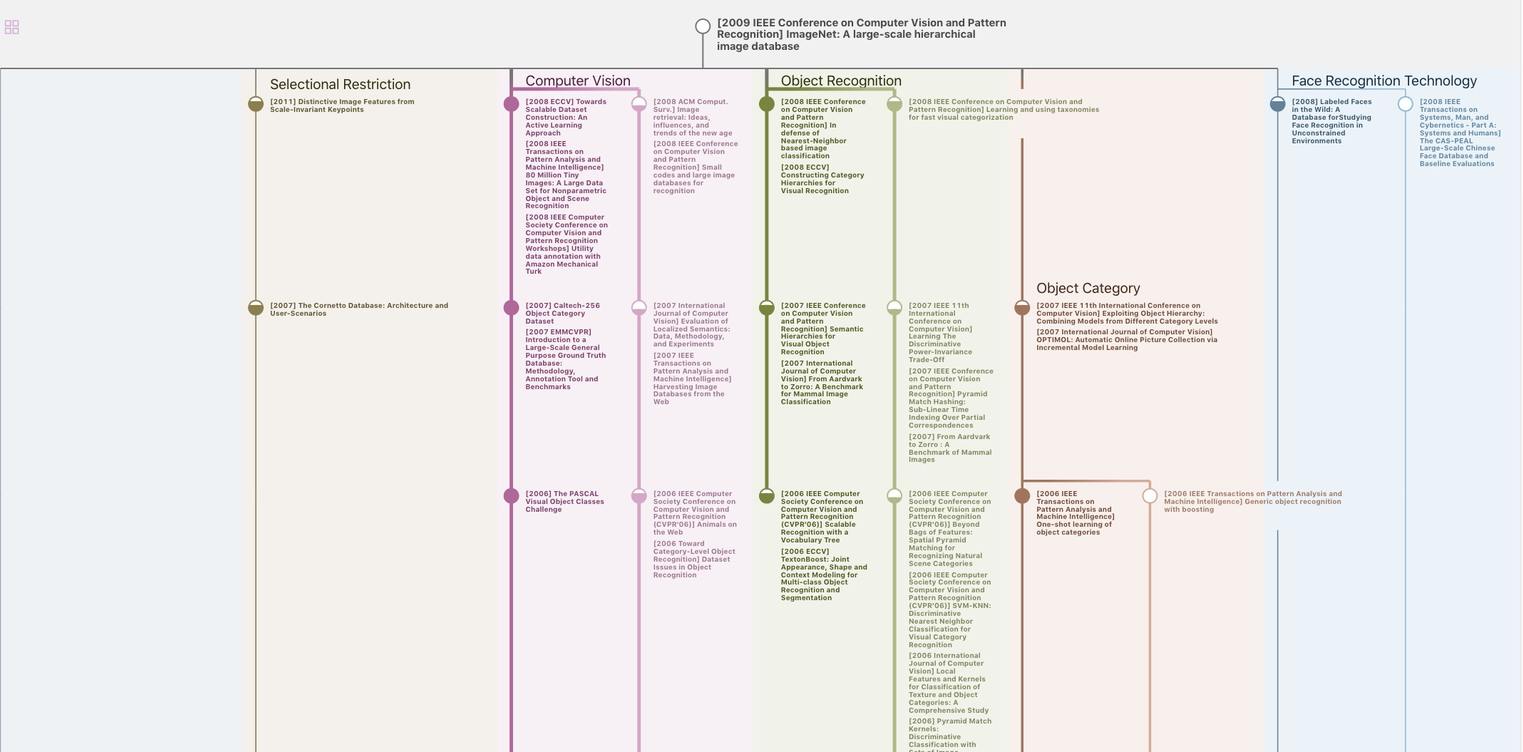
Generate MRT to find the research sequence of this paper
Chat Paper
Summary is being generated by the instructions you defined