Active optical sensor measurements and weather variables for predicting winter wheat yield (vol 113, pg 2742, 2015)
AGRONOMY JOURNAL(2022)
摘要
Accurate winter wheat (Triticum aestivum L.) grain yield prediction is vital for improving N management decisions. Currently, most N optimization algorithms use in-season estimated yield (INSEY) as a sole variable for predicting grain yield potential (YP). Although evidence suggests that this works, the yield prediction accuracy could be further improved by including other predictors in the model. The objective of this work was to evaluate INSEY, pre-plant N rate, total rainfall, and average air temperature from September to December as predictors of winter wheat YP. An 8-yr (2012-2019) data set for grain yield was obtained from Experiment 502, Lahoma, OK. The experiment was designed as a randomized complete block with four replications and N applied at 0, 45, 67, 90, and 112 kg ha(-1). Weather data was obtained from the Oklahoma Mesonet (). The data were analyzed using R statistical computing platform. The best model was selected using least absolute shrinkage and selection operator. Root mean square error (RMSE) was obtained using k-fold cross-validation. The model selection algorithm produced the full model as the best model for yield prediction with an R-2 of .79 and RMSE of 0.54 Mg ha(-1). The best one-variable model - as expected - used INSEY as the predictor and had the highest RMSE of 0.72 Mg ha(-1) and an R-2 of .62. Mid-season YP prediction accuracy could be improved by including pre-plant N rate, mean air temperature, and total rainfall from September to December in a model already containing INSEY.
更多查看译文
AI 理解论文
溯源树
样例
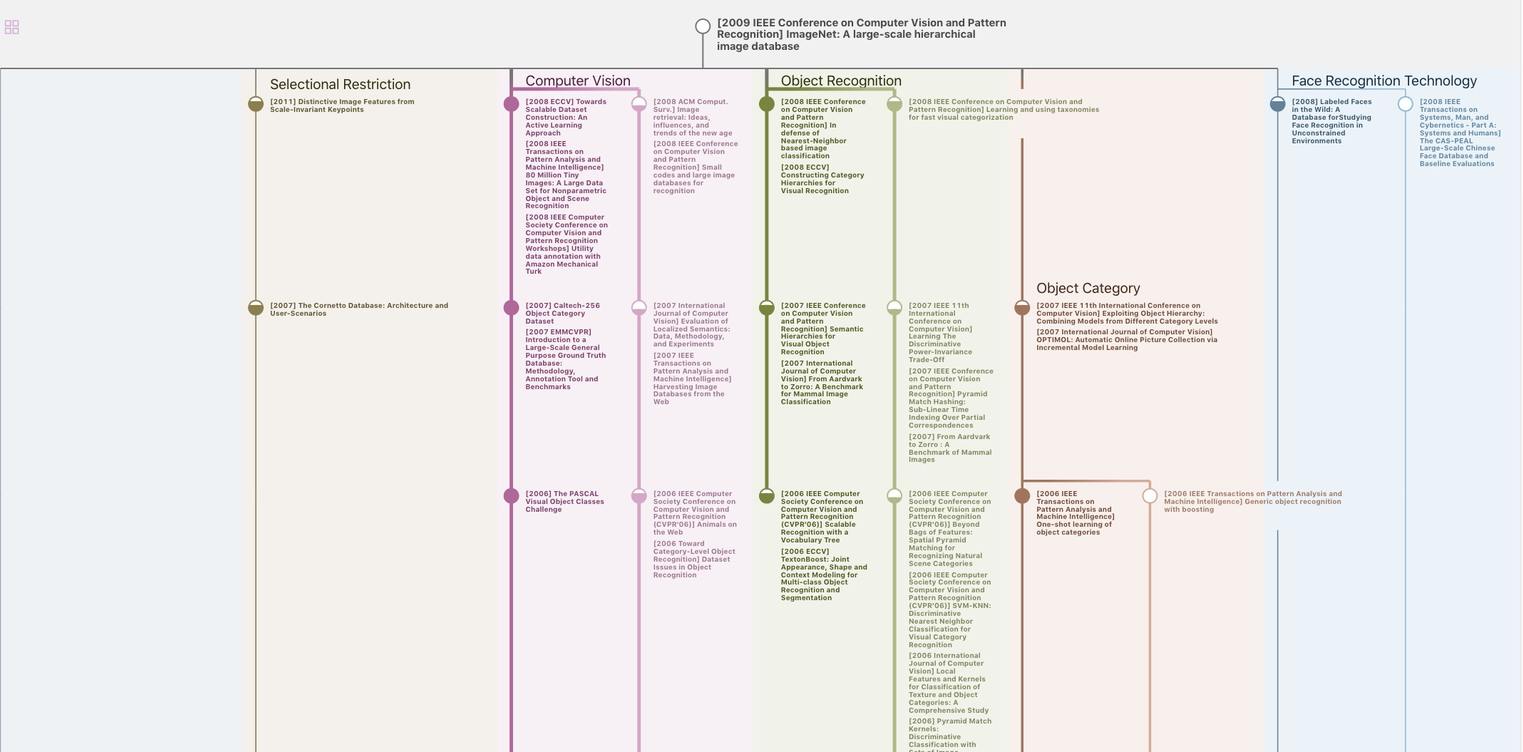
生成溯源树,研究论文发展脉络
Chat Paper
正在生成论文摘要