Motion Planning For Dual-Arm Robot Based On Soft Actor-Critic
IEEE ACCESS(2021)
摘要
In this paper, a motion planning method based on the Soft Actor-Critic (SAC) is designed for a dual-arm robot with two 7-Degree-of-Freedom (7-DOF) arms so that the robot can effectively avoid self-collision and at the same time can avoid the joint limits and singularities of the arm. The left-arm and right-arm of the dual-arm robot each have a neural network to control its position and orientation. Dual-agent training, distributed training structure, and progressive training environment are used to train neural networks. During the training process, the motion of one arm is regarded as the environment of the other arm, and the two agents are trained at the same time. In the input part of the neural network of the proposed method, all parameters come from the angle of each axis and kinematic calculation, no additional sensors are needed, so the method is easier to transplant to different dual-arm robots. With some appropriate neural network inputs and reward functions design, the robot can perform the expected self-collision avoidance and effectively avoid the joint limits and singularities of the arm. Finally, some experiments of the simulation tests in the Gazebo simulator and actual tests in a laboratory-made dual-arm robot are presented to illustrate the proposed SAC-based motion planning method is feasible and practicable in the avoidance of self-collision, joint limits, and singularities.
更多查看译文
关键词
Robots, Training, Planning, Collision avoidance, Manipulators, Reinforcement learning, Task analysis, Dual-arm robot, soft actor-critic (SAC), deep reinforcement learning (DRL), motion planning, self-collision avoidance
AI 理解论文
溯源树
样例
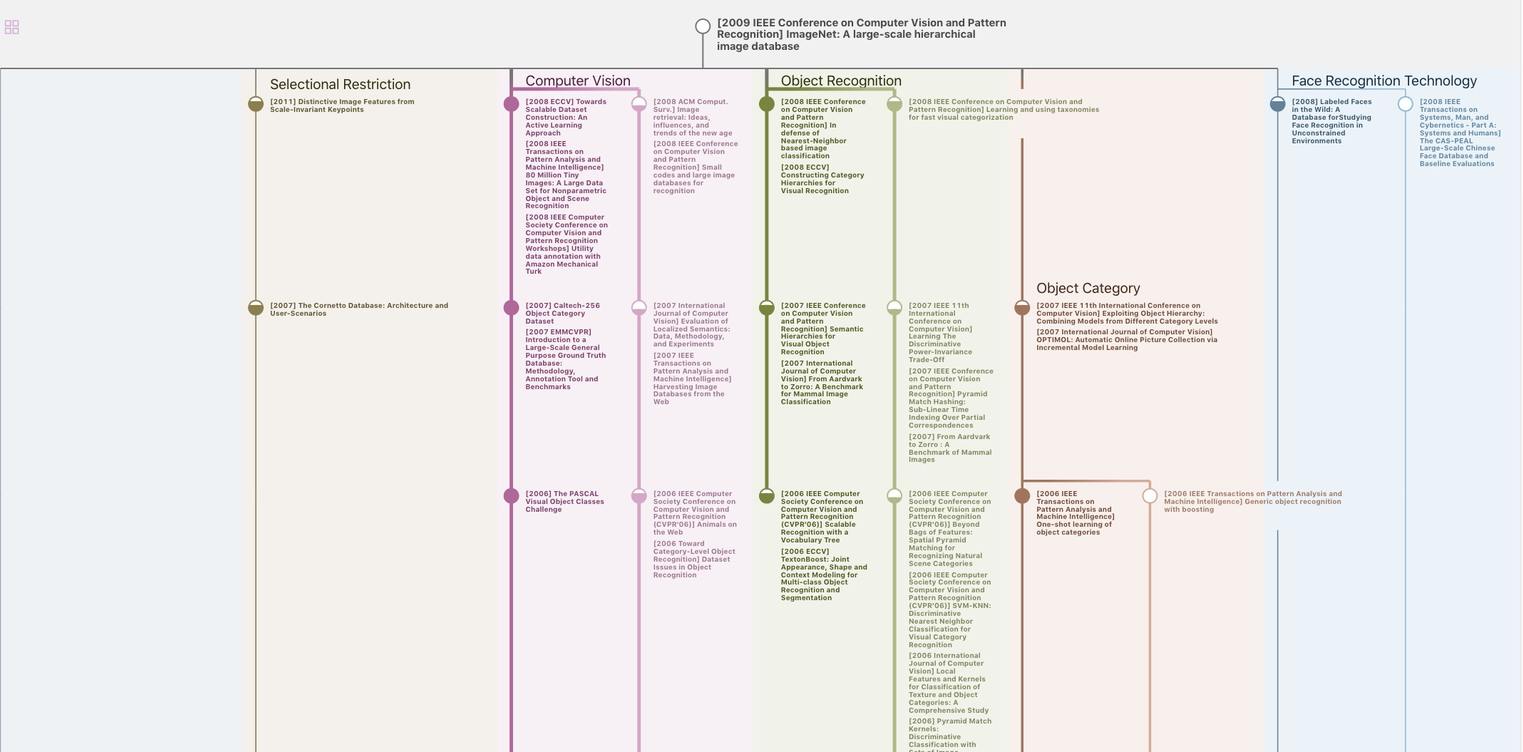
生成溯源树,研究论文发展脉络
Chat Paper
正在生成论文摘要