Adversarial Training for Session-based Item Recommendations
ieee joint international information technology and artificial intelligence conference(2020)
摘要
Deep neural networks (DNN) have become the mainstream in session-based recommendations (SR) due to their capability of learning feature representations from scratch and capturing the dynamic non-linear user-item relationships. Despite their popularity, these DNN-based SR models tend to be unstable, and small scale perturbations to the inputs may lead to incorrect predictions. In this paper, we propose two adversarial training (AT) methods to construct adversarial perturbations and add them to the DNN-based SR models so as to improve their robustness against adversarial perturbations and overfitting. The generated adversarial perturbations are applicable to any DNN-based SR models, which can be treated as common techniques for further improving the performance of DNN-based SR models. We investigate the effectiveness of the constructed adversarial perturbations by applying them to the two most important DNN architectures (recurrent neural network and convolutional neural network) on two benchmark datasets. Experimental results demonstrate that the AT can significantly improve the performance of the baseline methods.
更多查看译文
关键词
Item recommendation,adversarial training,virtual adversarial training
AI 理解论文
溯源树
样例
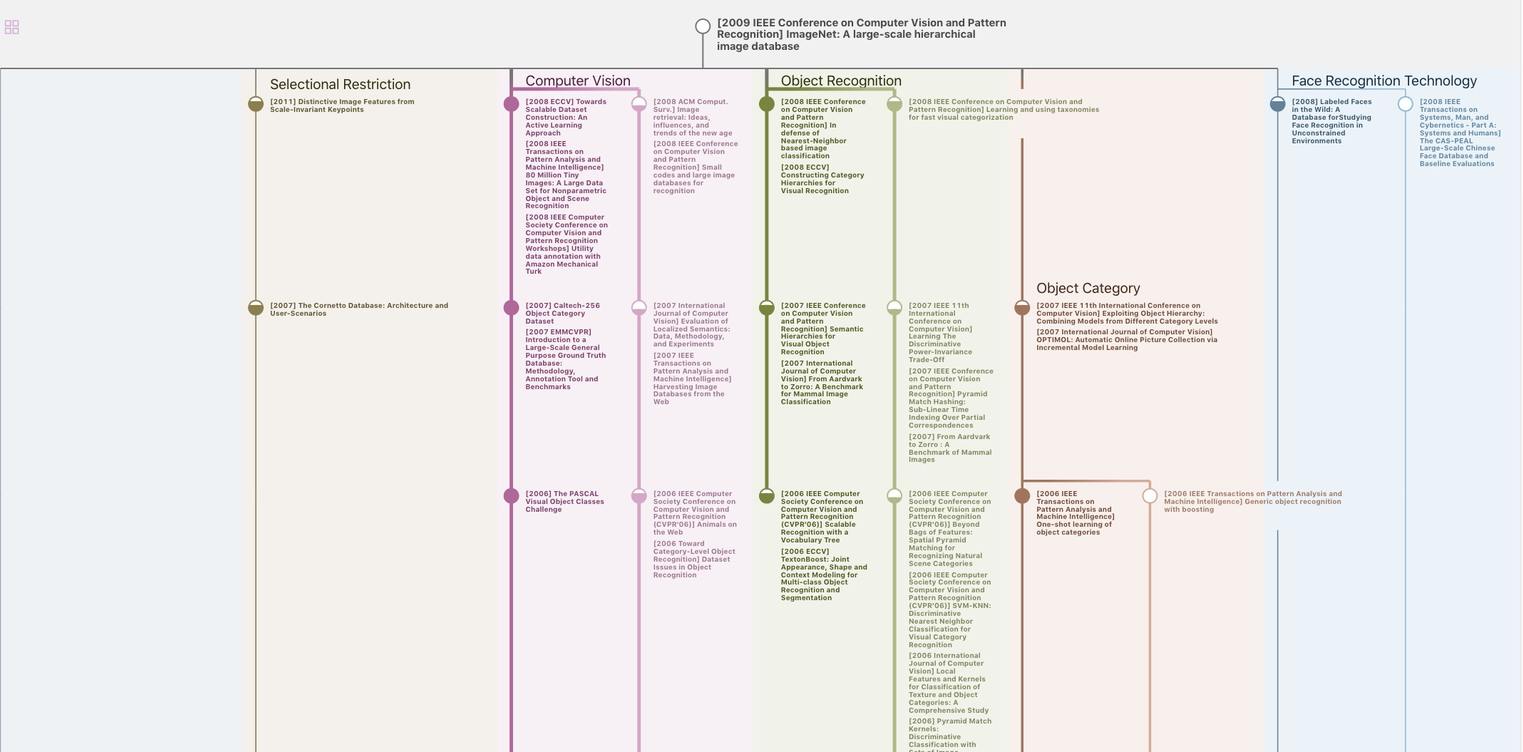
生成溯源树,研究论文发展脉络
Chat Paper
正在生成论文摘要