A Prediction Model Based on Artificial Neural Network for the Temperature Performance of a Hydrodynamic Retarder in Constant-Torque Braking Process
IEEE ACCESS(2021)
摘要
Excessively high brake temperature of hydrodynamic retarders may lead to brake fading and failure, resulting in a decrease in brake effectiveness. However, the temperature performance modeling of hydrodynamic retarders is a challenge because of the non-linear characteristics of the system. In this study, a temperature model based on an artificial neural network is constructed to predict the temperature performance of a hydrodynamic retarder in constant-torque braking process. The model is developed from a back-propagation neural network trained with the Levenberg-Marquardt algorithm. Before the application of the neural network, computational fluid dynamics is used to obtain the controllable region where experimental tests were performed to collect data for neural network training and validation. The linear regression method is adopted to check the quality of the training. It is shown that the constructed back-propagation neural network model is within 98% accuracy. Furthermore, the temperature model of the hydrodynamic retarder, which consists of the back-propagation neural network and thermal balance models, is simulated for 1500N.m, 2000N.m, and 2500N.m constant-torque braking processes. The simulation results are in agreement with experimental data within 2.87% error. The proposed temperature model can predict the temperature of the hydrodynamic retarder accurately and provide theoretical guidance for brake control strategy and thermal management.
更多查看译文
关键词
Hydraulic systems,Hydrodynamic retarder,back-propagation neural network,constant-torque braking,temperature performance
AI 理解论文
溯源树
样例
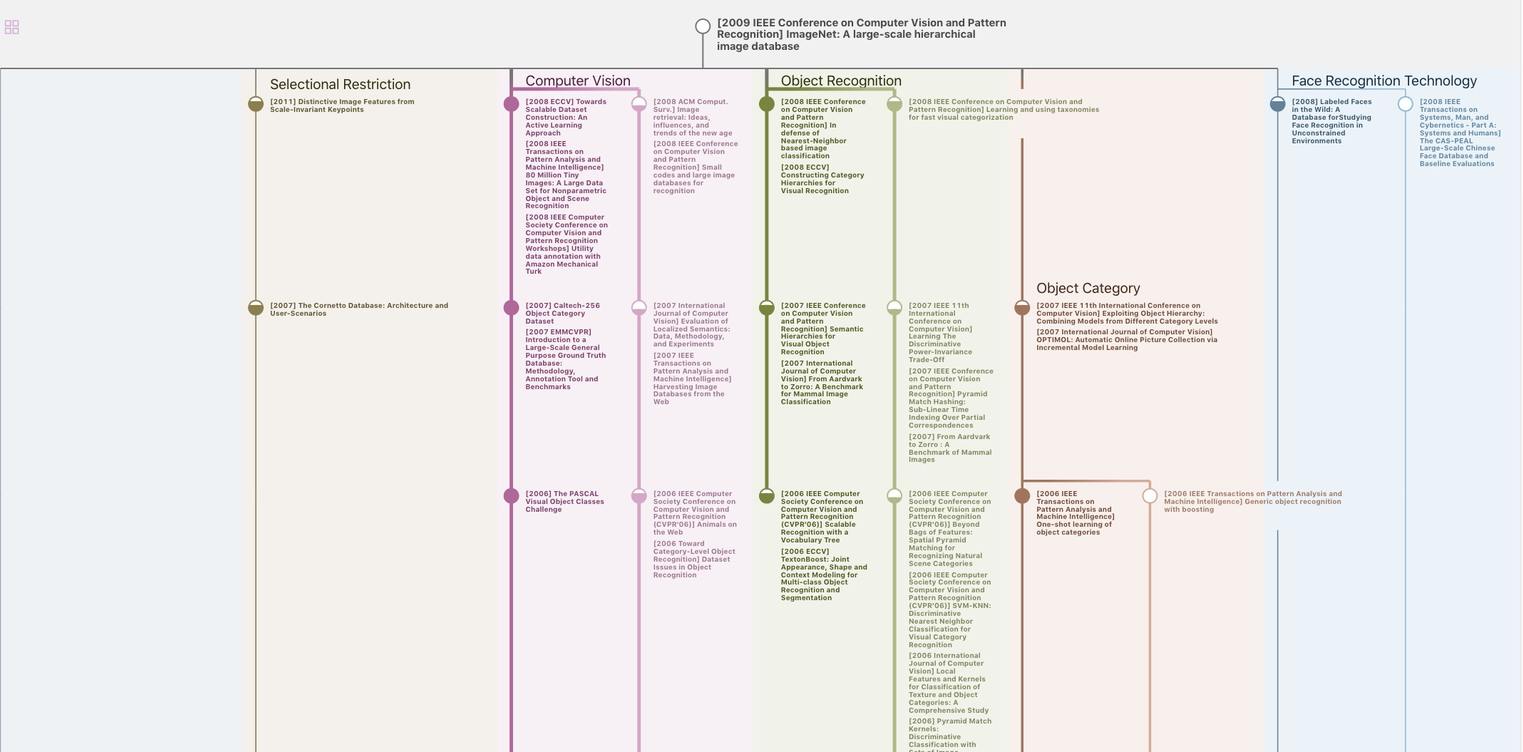
生成溯源树,研究论文发展脉络
Chat Paper
正在生成论文摘要