A Feature-Cascaded Correntropy Lstm For Tourists Prediction
IEEE ACCESS(2021)
摘要
Forecasting the number of tourists is significant to public safety, which can enable the government to control the sudden influx of tourists timely. The temporal dependence (closeness and period), external factors such as holidays, government policy, as well as outliers in real data, make the prediction challenging. Our data obtain a mixture of short-term contact and long-term repeating patterns and external factors, for which Autoregressive, Exponential Smoothing models and Extreme Learning Machine may fail. In our paper, we propose a novel Feature-Cascaded framework with Correntropy criterion for Long Short-Term Memory network (FC-C-LSTM). For temporal dependence and external factors, we first extract feature information from data in close dates and corresponding period, then integrate them as an independent input of LSTM to simulate temporal pattern and solve the problem of time-lag. In view of an important and unavoidable feature of real datasets, there are amounts of outliers. We adopt the correntropy of Gaussian kernel instead of mean square error as cost function, so that outliers get smaller weights and suppress the influence of outliers back-propagation. Experiments on real tourism datasets of several cities in Yunnan Province show that FC-C-LSTM model achieves the better performance than that of other baselines.
更多查看译文
关键词
Predictive models, Feature extraction, Time series analysis, Forecasting, Data models, Task analysis, Recurrent neural networks, Prediction, LSTM, correntropy, outliers
AI 理解论文
溯源树
样例
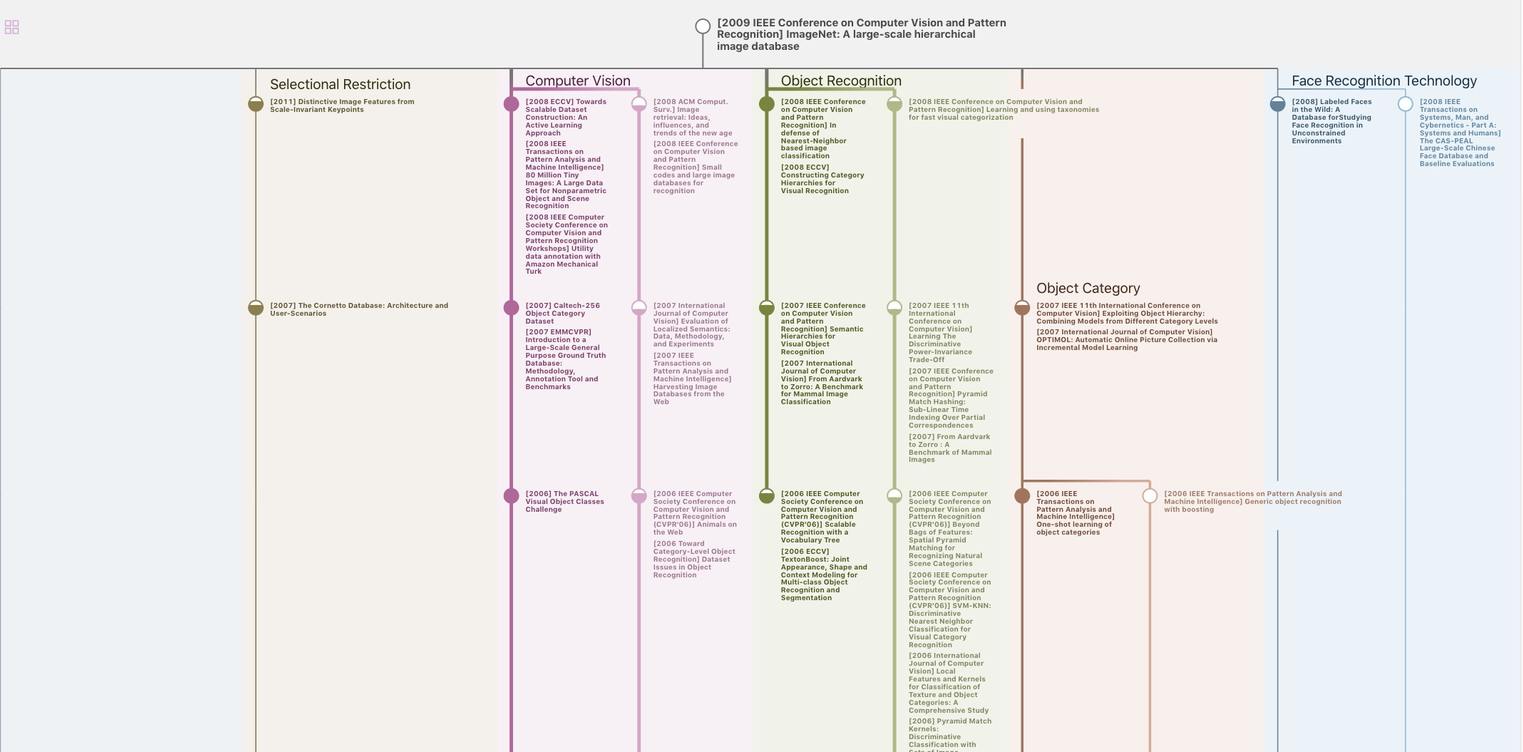
生成溯源树,研究论文发展脉络
Chat Paper
正在生成论文摘要