A Wavelet-Based Asymmetric Convolution Network For Single Image Super-Resolution
IEEE ACCESS(2021)
摘要
Recently, deep convolutional neural networks (CNNs) have been widely explored in single image super-resolution(SISR) and obtained remarkable performance. However, most of the existing CNN-based SISR methods tend to produce over-smoothed outputs and miss some textural details. To address these issues, we propose a wavelet-based asymmetric convolution network (WACN). Different from conventional CNN methods that directly infer HR images, our approach firstly learns to predict the LR's corresponding series of HR's wavelet coefficients before reconstructing HR images from them. This helps to capture more structural information in images to preserve texture information and avoid artifacts. To enhance the ability of feature extraction, we propose an asymmetric convolution block (ACB) structure to form a very deep network. In the training phase, ACB can provide different receptive fields to enrich feature information. In the inference phase, ACB's asymmetric convolution kernel can be equivalently fused into the standard square-kernel layers, such that no extra computational burdens are introduced in the inference phase. Furthermore, we propose a variance-based channel attention (VCA) mechanism to adaptively rescale channel-wise features by considering interdependencies among channels. Extensive experimental results demonstrate the superiority of the proposed WACN in comparison with the state-of-the-art methods.
更多查看译文
关键词
Licenses, Feature extraction, Convolution, Image reconstruction, Wavelet domain, Wavelet coefficients, Optical wavelength conversion, Asymmetric convolution network, single super-resolution, variance-based channel attention, wavelet
AI 理解论文
溯源树
样例
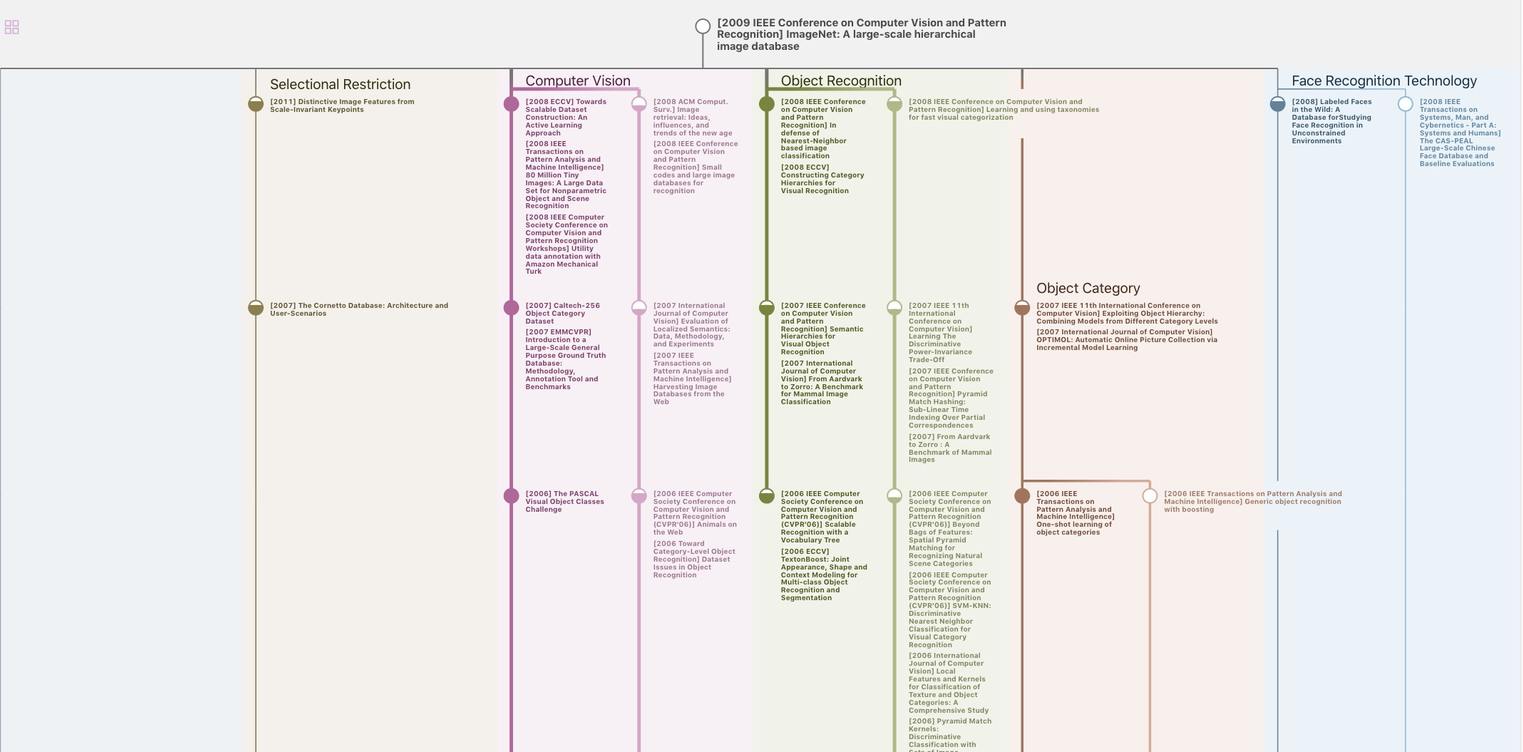
生成溯源树,研究论文发展脉络
Chat Paper
正在生成论文摘要