Automated Bughole Detection And Quality Performance Assessment Of Concrete Using Image Processing And Deep Convolutional Neural Networks
CONSTRUCTION AND BUILDING MATERIALS(2021)
摘要
Concrete is ubiquitous in the modern construction and building industry, bugholes are one of the most typical imperfections on concrete surface, and traditional manual inspection techniques are inefficient and difficult to rapidly deploy. With the development of image processing and deep learning techniques, new opportunities and possible solutions are available for bughole detection. This paper not only dedicates to propose a novel approach to automatically extract bugholes in the concrete surface images with high fidelity by combining the advantages of image processing and deep convolutional neural network (DCNN), but also attempts to assess the quality performance of concrete based on the detection results. Firstly, a series of image processing procedures were performed to highlight the characteristics of bugholes in the acquired images and reduce the data size. Secondly, a DCNN was proposed to automatically detect bugholes in the images, an inception module consisting of multiple convolutions and a max pooling operation was introduced into the network to efficiently extract multiscale features and reduce the model parameters. Favorable detection results were obtained in the experimental tests, especially the small bugholes (i.e., diameter ranging from 0.3 mm to 1 mm) can be accurately detected. Finally, the quality performance of concrete specimens was assessed based on the detection results, the potential relationship between the attributes of bugholes and compressive strength of concrete specimens was revealed through a fitting function. The proposed method makes both the detection of bugholes and assessment of concrete automatable, efficient, and low cost, potentially aiding engineers in inspecting concrete structures in a fast and reliable manner. (C) 2021 Elsevier Ltd. All rights reserved.
更多查看译文
关键词
Concrete, Bughole, Image processing, Deep convolutional neural network, Quality performance assessment, Structural health monitoring
AI 理解论文
溯源树
样例
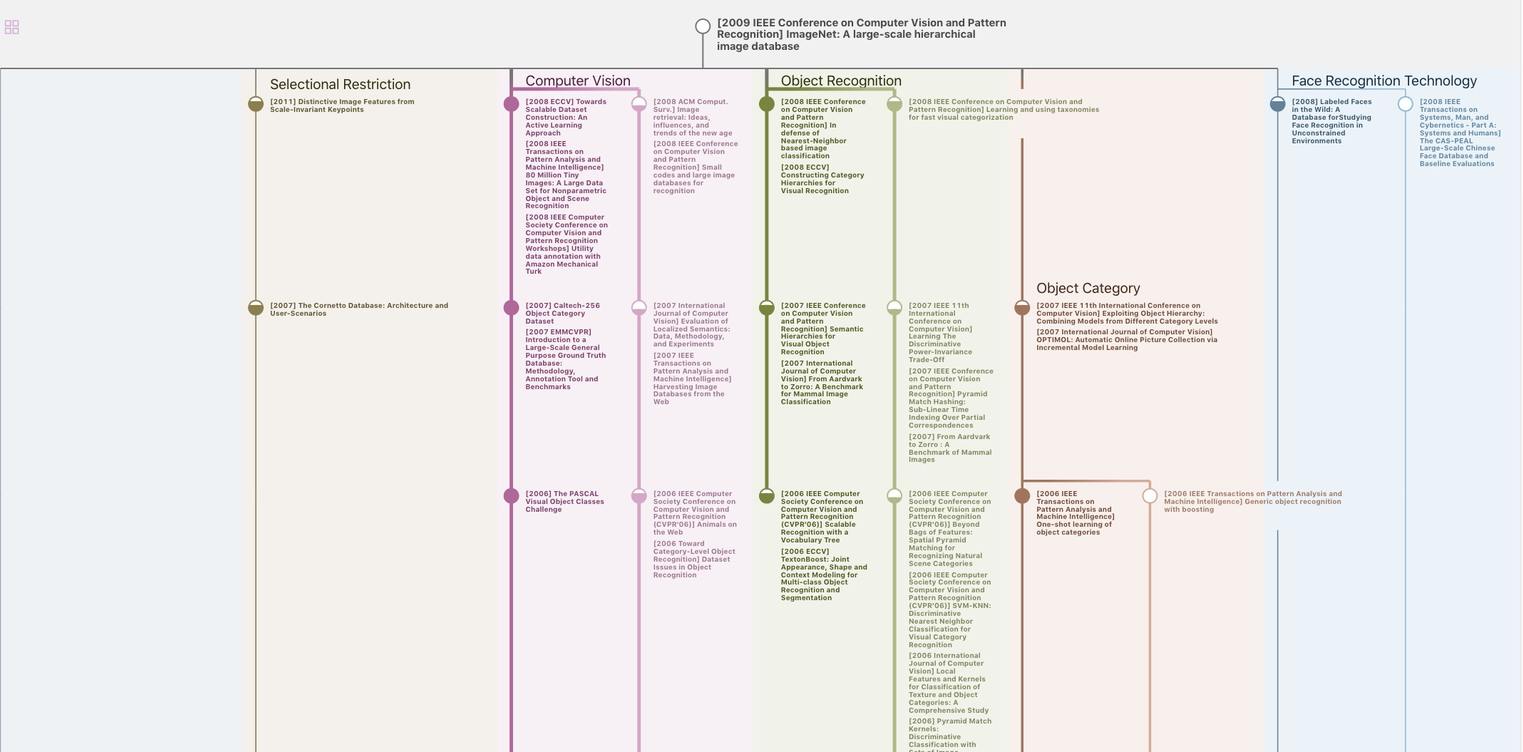
生成溯源树,研究论文发展脉络
Chat Paper
正在生成论文摘要