A hybrid approach for short-term traffic flow forecasting based on similarity identification
MODERN PHYSICS LETTERS B(2021)
摘要
Short-term traffic flow forecasting is a key component of intelligent transportation system, yet difficult to be forecasted reliably, and accurately. A novel hybrid forecasting model is proposed by combining three predictors, namely, the autoregressive integrated moving average (ARIMA), back propagation neural network (BPNN) and support vector regression (SVR). First, it is assumed that all previous intervals can have influence on the predicted interval and then the entropy-based gray relation analysis method is applied to analyze the correlation and determine the length of time constrain window. Second, an improved Euclidean distance is employed to identify the similarity. Furthermore, the rank-exponent method is utilized to rank the results according to the similarity and fuse the predicted values of the predictors. Finally, a numerical experiment is implemented, which indicates that the performance of forecasting results is superior to the conventional ones.
更多查看译文
关键词
Traffic flow,short-term traffic forecasting,similarity identification,time constrain window,entropy-based gray relation analysis,rank-exponent method
AI 理解论文
溯源树
样例
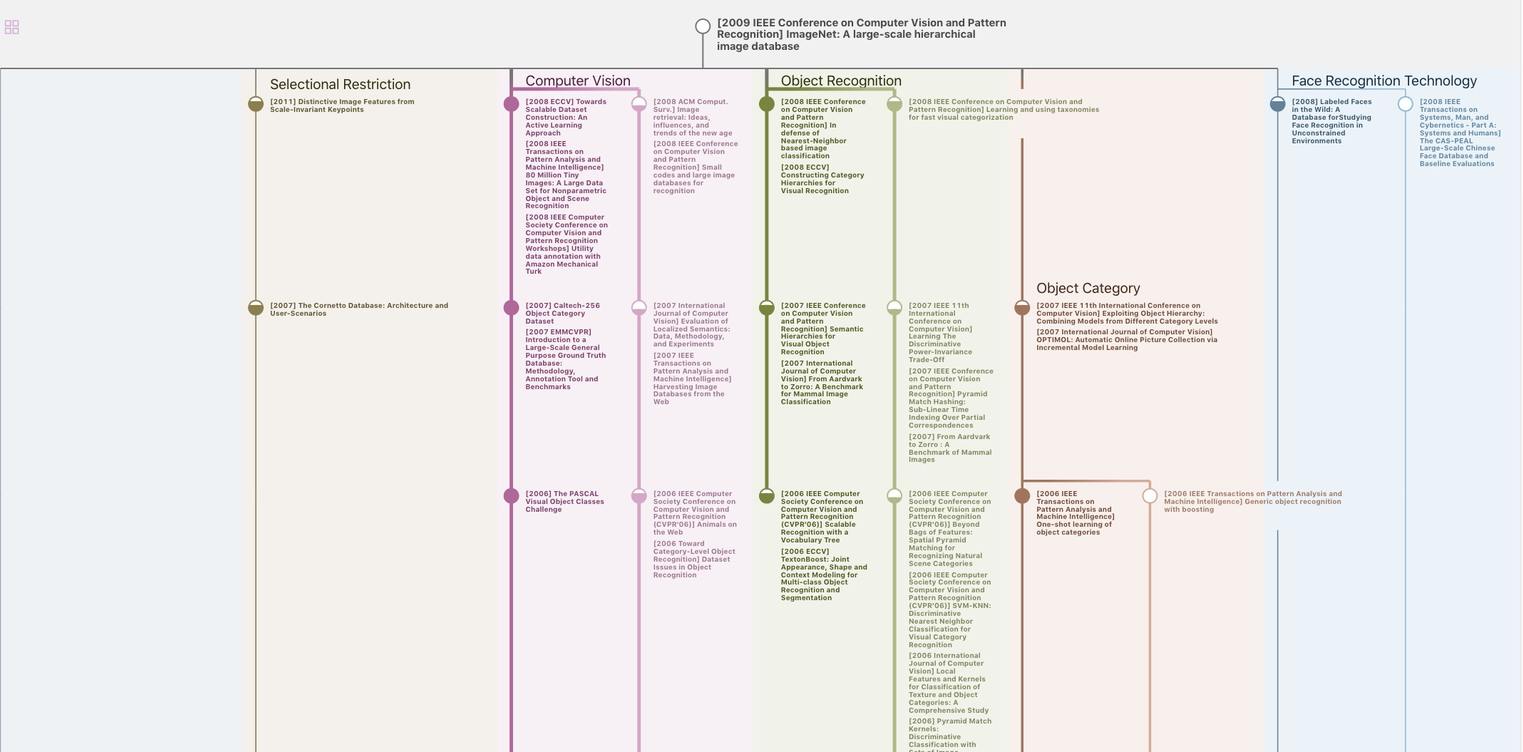
生成溯源树,研究论文发展脉络
Chat Paper
正在生成论文摘要