Supervised Versus Self-Supervised Assistant For Surveillance Of Harbor Fronts
VISAPP: PROCEEDINGS OF THE 16TH INTERNATIONAL JOINT CONFERENCE ON COMPUTER VISION, IMAGING AND COMPUTER GRAPHICS THEORY AND APPLICATIONS - VOL. 5: VISAPP(2021)
摘要
Drowning in harbors and along waterfronts is a serious problem, worsened by the challenge of achieving timely rescue efforts. To address this problem, we propose a privacy-friendly assistant surveillance system for identifying potentially hazardous situations (human activities near the water's edge) in order to give early warning. This will allow lifeguards and first responders to react proactively with a basis in accurate information. In order to achieve this, we develop and compare two vision-based solutions. One is a supervised approach based on the popular object detection framework, which allows us to detect humans in a defined area near the water's edge. The other is a self-supervised approach where anomalies are detected based on the reconstruction error from an autoencoder. To best comply with privacy requirements both solutions rely on thermal imaging captured in an active harbor environment. With a dataset having both safe and risky scenes, the two solutions are evaluated and compared, showing that the detector-based method wins in terms of performances, while the autoencoder-based method has the benefit of not requiring expensive annotations.
更多查看译文
关键词
Safety, Drowning, Surveillance, Thermal Imaging, Deep Learning, Human Detection, Anomaly Detection
AI 理解论文
溯源树
样例
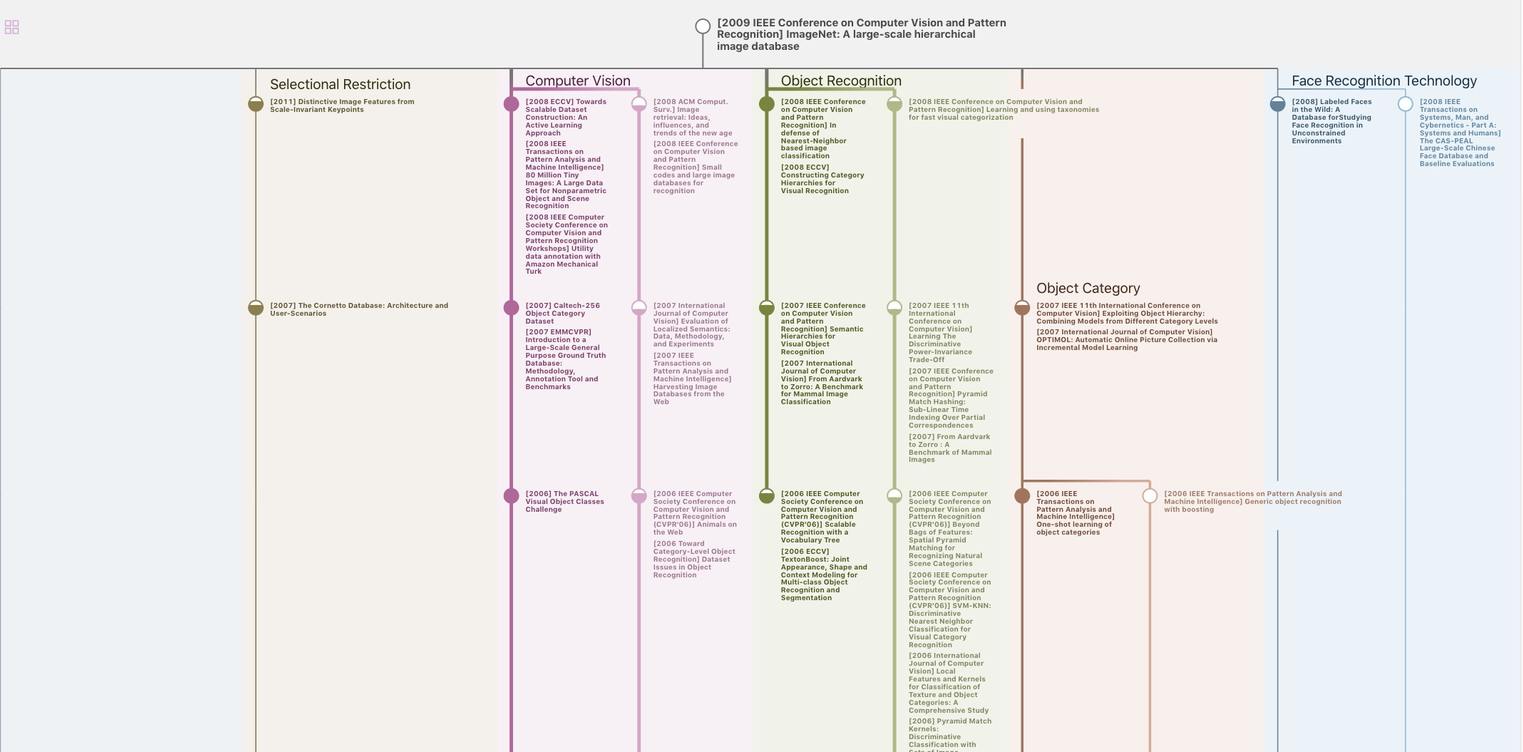
生成溯源树,研究论文发展脉络
Chat Paper
正在生成论文摘要