A Disturbance Rejection Control Method Based On Deep Reinforcement Learning For A Biped Robot
APPLIED SCIENCES-BASEL(2021)
摘要
The disturbance rejection performance of a biped robot when walking has long been a focus of roboticists in their attempts to improve robots. There are many traditional stabilizing control methods, such as modifying foot placements and the target zero moment point (ZMP), e.g., in model ZMP control. The disturbance rejection control method in the forward direction of the biped robot is an important technology, whether it comes from the inertia generated by walking or from external forces. The first step in solving the instability of the humanoid robot is to add the ability to dynamically adjust posture when the robot is standing still. The control method based on the model ZMP control is among the main methods of disturbance rejection for biped robots. We use the state-of-the-art deep-reinforcement-learning algorithm combined with model ZMP control in simulating the balance experiment of the cart-table model and the disturbance rejection experiment of the ASIMO humanoid robot standing still. Results show that our proposed method effectively reduces the probability of falling when the biped robot is subjected to an external force in the x-direction.
更多查看译文
关键词
biped robot, model zero-moment-point control, deep reinforcement learning
AI 理解论文
溯源树
样例
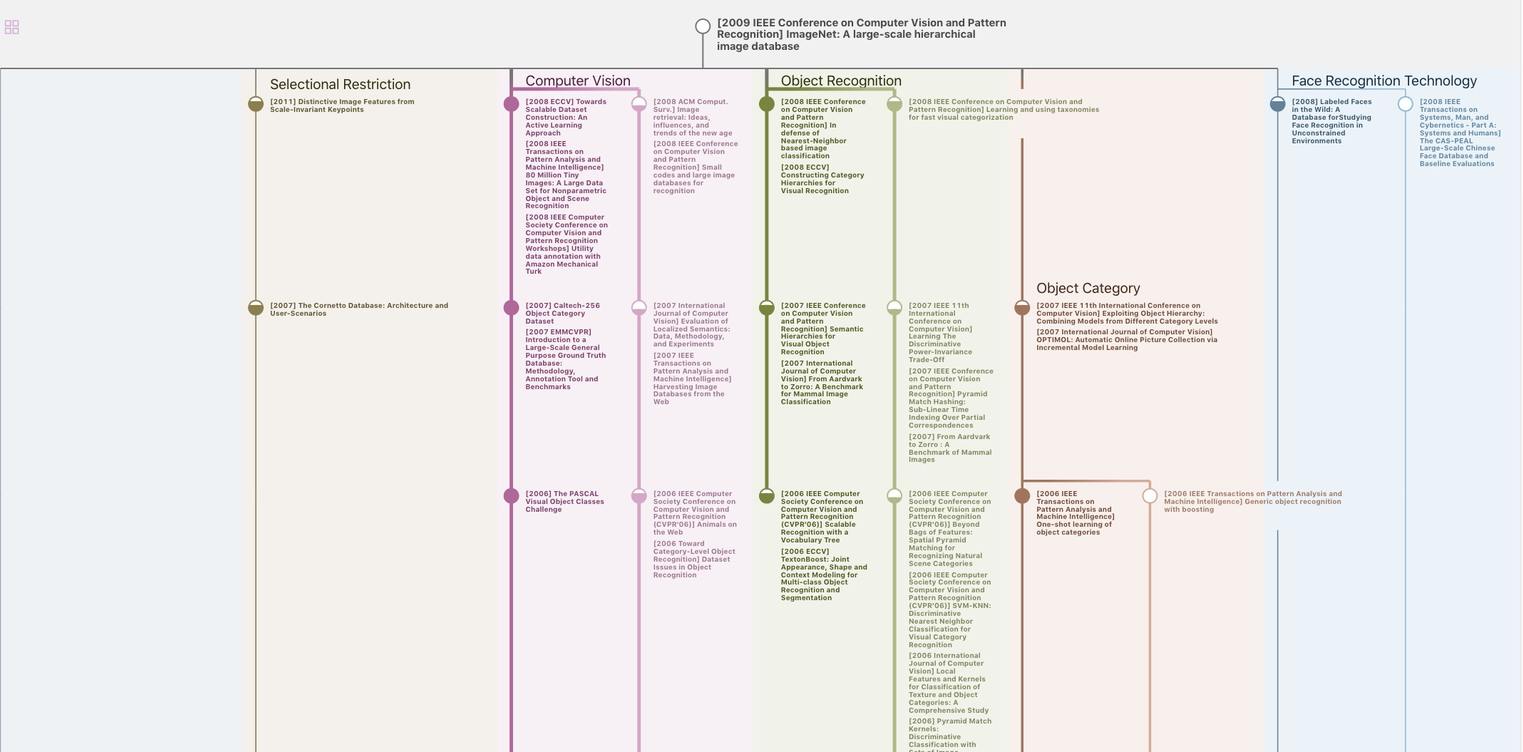
生成溯源树,研究论文发展脉络
Chat Paper
正在生成论文摘要