Knowledge Graph Embedding by Double Limit Scoring Loss
IEEE Transactions on Knowledge and Data Engineering(2022)
摘要
Knowledge graph embedding is an effective way to represent knowledge graph, which greatly enhance the performances on knowledge graph completion tasks, e.g., entity or relation prediction. For knowledge graph embedding models, designing a powerful loss framework is crucial to the discrimination between correct and incorrect triplets. Margin-based ranking loss is a commonly used negative sampling framework to make a suitable margin between the scores of positive and negative triples. However, this loss can not ensure ideal low scores for the positive triplets and high scores for the negative triplets, which is not beneficial for knowledge completion tasks. In this paper, we present a double limit scoring loss to separately set upper bound for correct triplets and lower bound for incorrect triplets, which provides more effective and flexible optimization for knowledge graph embedding. Upon the presented loss framework, we present several knowledge graph embedding models including TransE-SS, TransH-SS, TransD-SS, ProjE-SS and ComplEx-SS. The experimental results on link prediction and triplet classification show that our proposed models have the significant improvement compared to state-of-the-art baselines.
更多查看译文
关键词
Knowledge graph,embedding,representation learning,knowledge graph completion,loss function
AI 理解论文
溯源树
样例
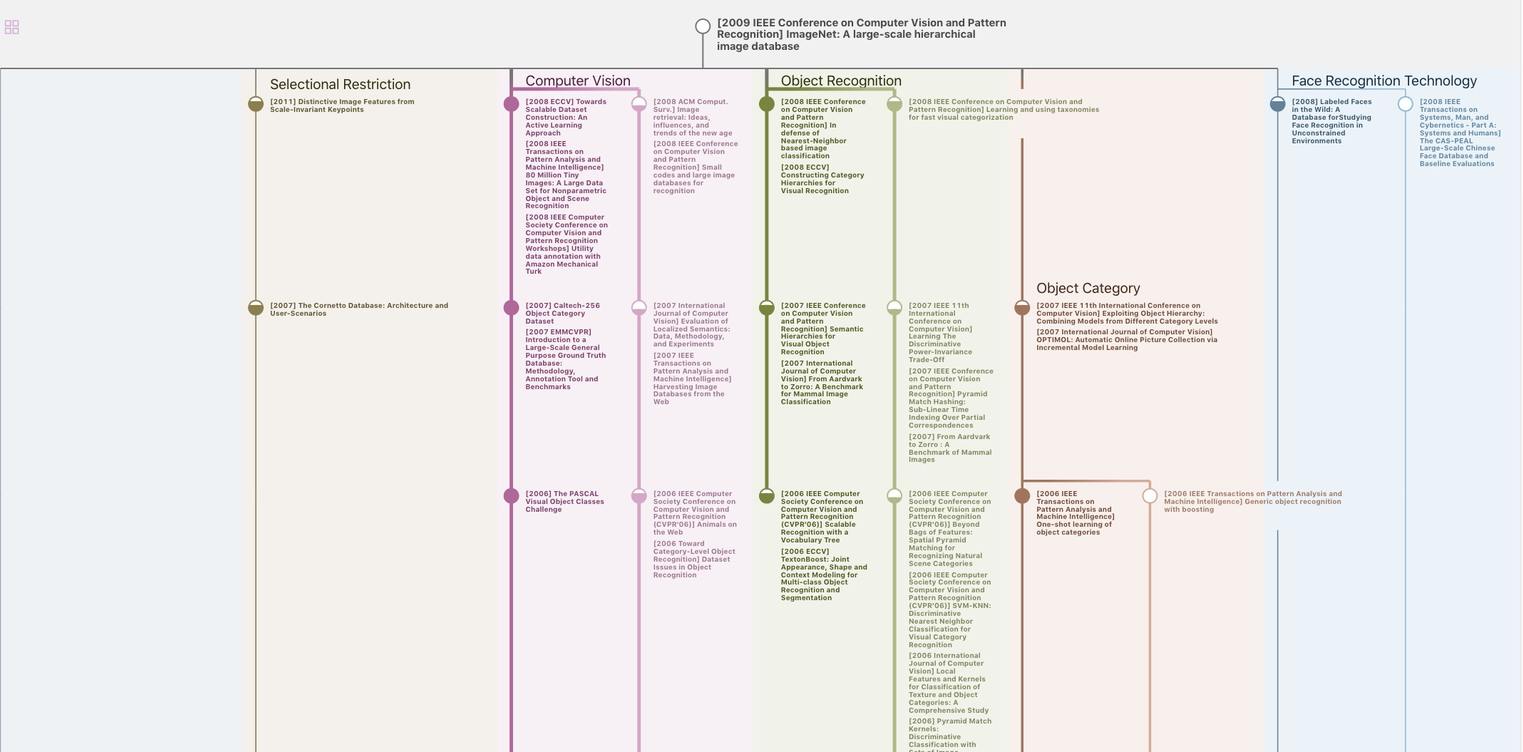
生成溯源树,研究论文发展脉络
Chat Paper
正在生成论文摘要