Learning to locate for fine-grained image recognition
Computer Vision and Image Understanding(2021)
摘要
In this paper, we propose an end-to-end weakly supervised method for fine-grained image recognition called bounding box-part location method(BBPL), which can locate the object and part precisely without part annotations. The proposed method includes three modules: object detection, ObjectMask, and classification. Firstly, the object detection module predicts the bounding boxes, and the predicted bounding boxes are employed to generate a mask through ObjectMask module. The generated mask can suppress the background interference during recognition. Secondly, the classification module can be further divided into two branches, which are global feature classification and local feature classification. In global feature classification branch, global feature is extracted to get global classification result. While in local feature classification branch, salient point is first detected through our novel salient point detection module, which can greatly reduce the consuming-time compared with the most existing local feature extraction methods. Further, the local feature is extracted in these detected salient points, and local classification result is obtained by local feature classification branch. Finally, we get the final result by fusing the results of two classification branches together. With experiments on three widely used fine-grained image recognition datasets (CUB-200-2011, Stanford Cars, Stanford Dogs), our method can achieve the state-of-the-art performance.
更多查看译文
关键词
Background suppression,CNN,Feature extraction,Fine-grained image recognition,Salient point detection,Weakly supervised
AI 理解论文
溯源树
样例
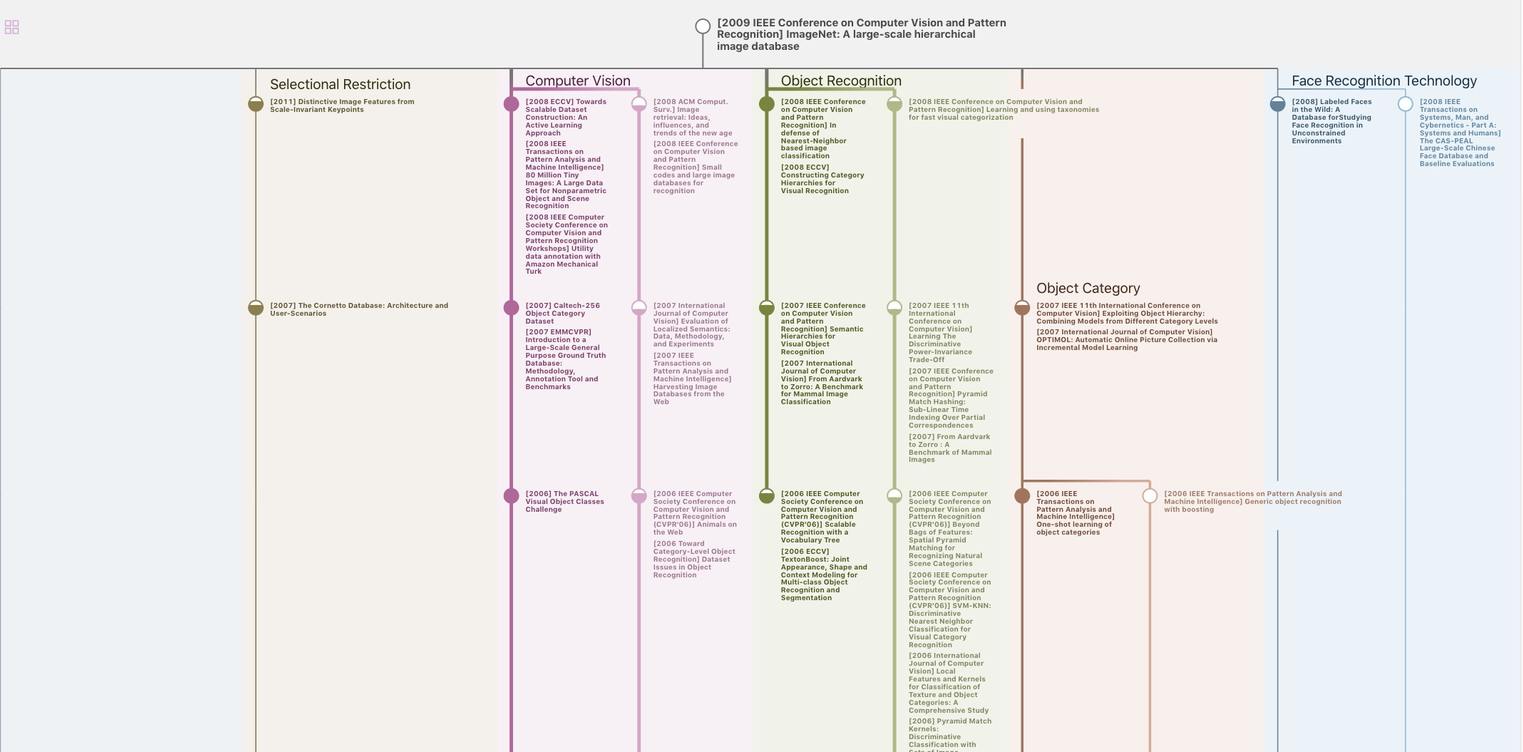
生成溯源树,研究论文发展脉络
Chat Paper
正在生成论文摘要