Generic User-Guided Interaction Paradigm For Precise Post-Slice-Wise Processing Of Tomographic Deep Learning Segmentations Utilizing Graph Cut And Graph Segmentation
VISAPP: PROCEEDINGS OF THE 16TH INTERNATIONAL JOINT CONFERENCE ON COMPUTER VISION, IMAGING AND COMPUTER GRAPHICS THEORY AND APPLICATIONS - VOL. 4: VISAPP(2021)
摘要
State of the art deep learning (DL) manifested in image processing as an accurate segmentation method. Nevertheless, its black-box nature hardly allows user interference. In this paper, we present a generic Graph cut (GC) and Graph segmentation (GS) approach for user-guided interactive post-processing of segmentations resulting from DL. The GC fitness function incorporates both, the original image characteristics and DL segmentation results, combining them with weights optimized by evolution strategy optimization. To allow for accurate user-guided processing, the fore- and background seeds of the Graph cut are automatically selected from the DL segmentations, but implementing effective features for expert input for adaptions of position and topology. The seamless integration of DL with GC/GS leads to marginal trade-off in quality, namely Jaccard (JI) 1.3% for automated GC and JI 0.46% for GS only. Yet, in specific areas where a well-trained DL model may potentially fail, precise adaptions at a low demand for user-interaction become feasible and thus even outperforming the original DL results. The potential of GC/GS is shown running on ground-truth seeds thereby outperforming DL by 0.44% JI for the GC and even by 1.16% JI for the GS. Iterative slice-by-slice progression of the post-processed and improved results keeps the demand for user-interaction low.
更多查看译文
关键词
Graph Cut, Graph Segmentation, U-Net, Deep Learning Image Segmentation, Evolution-strategy, User-guided Medical Image Analysis
AI 理解论文
溯源树
样例
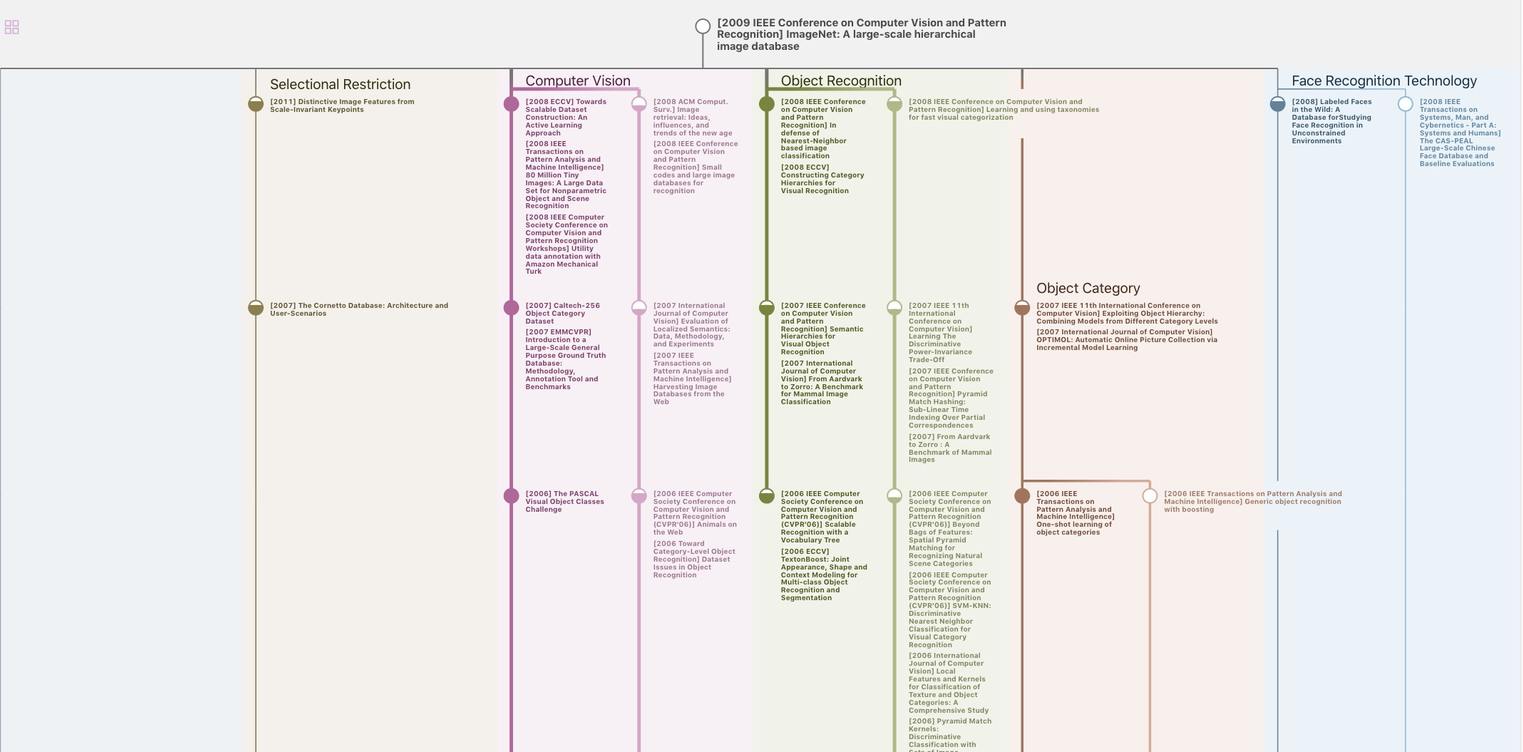
生成溯源树,研究论文发展脉络
Chat Paper
正在生成论文摘要