Machine Learning Enabled Phase Unwrapping for Digitalholographic Microscopy
Biophysical journal(2021)
摘要
Digital holographic microscopy (DHM) extrapolate volumetric information from one-shot 2D hologram measurements, allowing for quantitative biophotonic phase imaging. The temporal resolution advantage of DHM facilitates label-free insight intospecific physical properties of1 and morphological changes inside the cell2-4 for in-situ imaging of multi-cellular dynamics.One of the key processes in the 3D reconstruction pipeline of DHM is two-dimensional phase unwrapping (2D PU), aiming to restore the original phase information from the wrapped phase confined in intervals of 2π. However, 2D PU, as conventionally carried out through mathematical computation, lack robustness to hologram speckle noise and fringe artifact. Classical 2D PU methods are also susceptible to estimation error for abrupt phase changes exceeding the wavelength of the illumination source, eminently rectifiable by multi-wavelength sources.To address these problems, we propose a solution employing a Convolutional Neural Network (CNN) based deep learning framework for robust, high-SNR 2D PU. Our preliminary results demonstrate robustness against noise and phase discontinuities. We explored the performance of this method using HeLa cells and artificial micro-beads. This technology has the potential to cover more sample types and advance DHM's image quality further.
更多查看译文
AI 理解论文
溯源树
样例
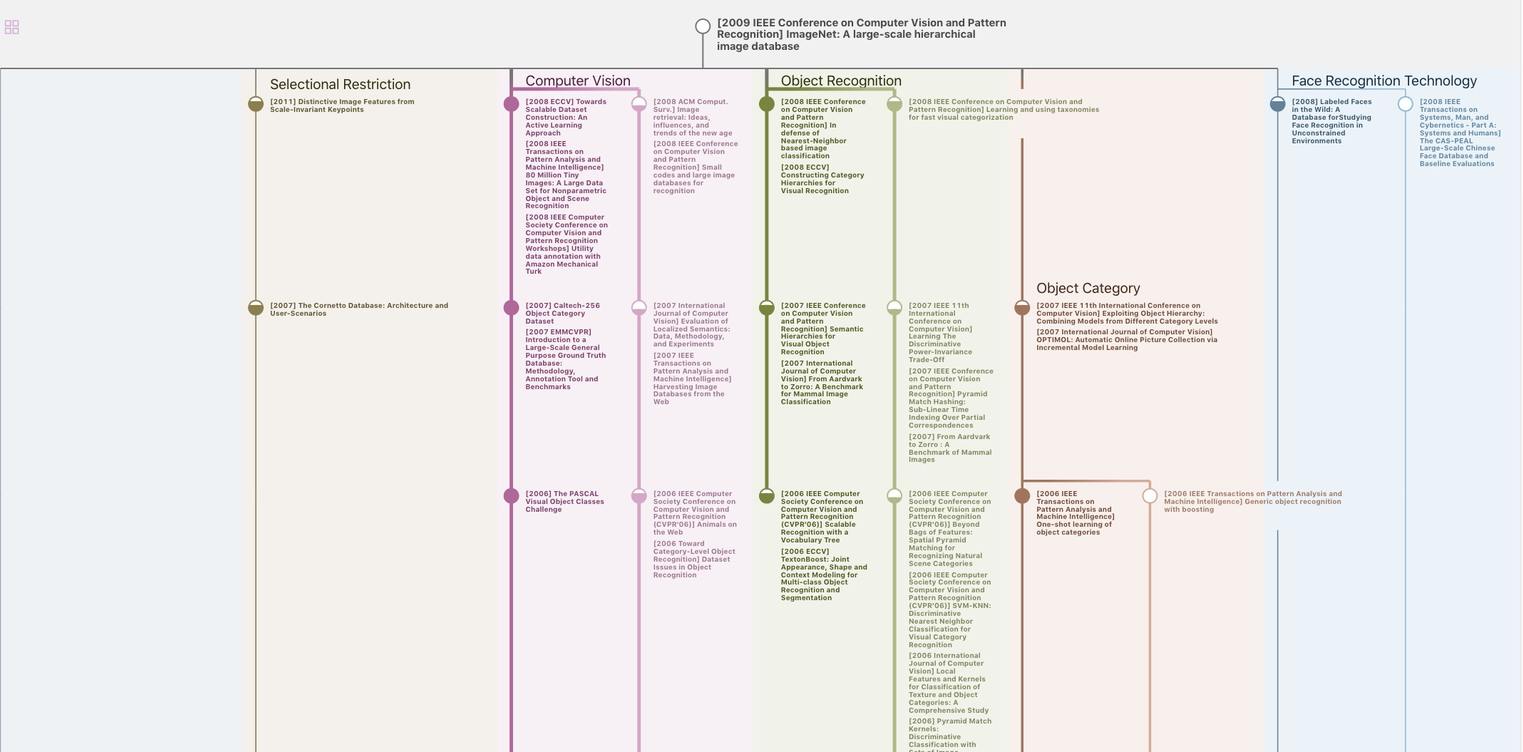
生成溯源树,研究论文发展脉络
Chat Paper
正在生成论文摘要