Driving Mode Predictor-Based Real-Time Energy Management for Dual-Source Electric Vehicle
IEEE Transactions on Transportation Electrification(2021)
摘要
To minimize battery aging of electric vehicles (EVs), it is paramount to manage efficiently their energy consumption. An energy management strategy (EMS) has recently been developed, where batteries and supercapacitors (SCs) are coordinated as a function of the driving mode which is determined manually by the driver. In the present article, we improve the EMS by developing a driving mode predictor (DMP) that determines automatically in real-time the driving mode simply from the speed history of the vehicle. The DMP is designed using supervised learning (SL), a branch of machine learning (ML). A strength of our approach is that it is applicable to predict the driving modes of any EV. To predict the driving modes during a trip made by a given EV, the DMP needs to follow the speed evolution of the EV and know its maximum reachable speed. The integration of the DMP into the EMS results in an enhanced EMS called DMP-based EMS that determines automatically in real-time the power to take from each source of energy of the EV as a function of its speed history. The results obtained from real driving cycles confirm the prediction quality of the DMP and the energy efficiency of the proposed DMP-based EMS.
更多查看译文
关键词
Driving mode predictor (DMP),dual-source electric vehicle (EV),energy consumption of EV (ECEV),energy management strategy (EMS),machine learning (ML),supervised learning (SL)
AI 理解论文
溯源树
样例
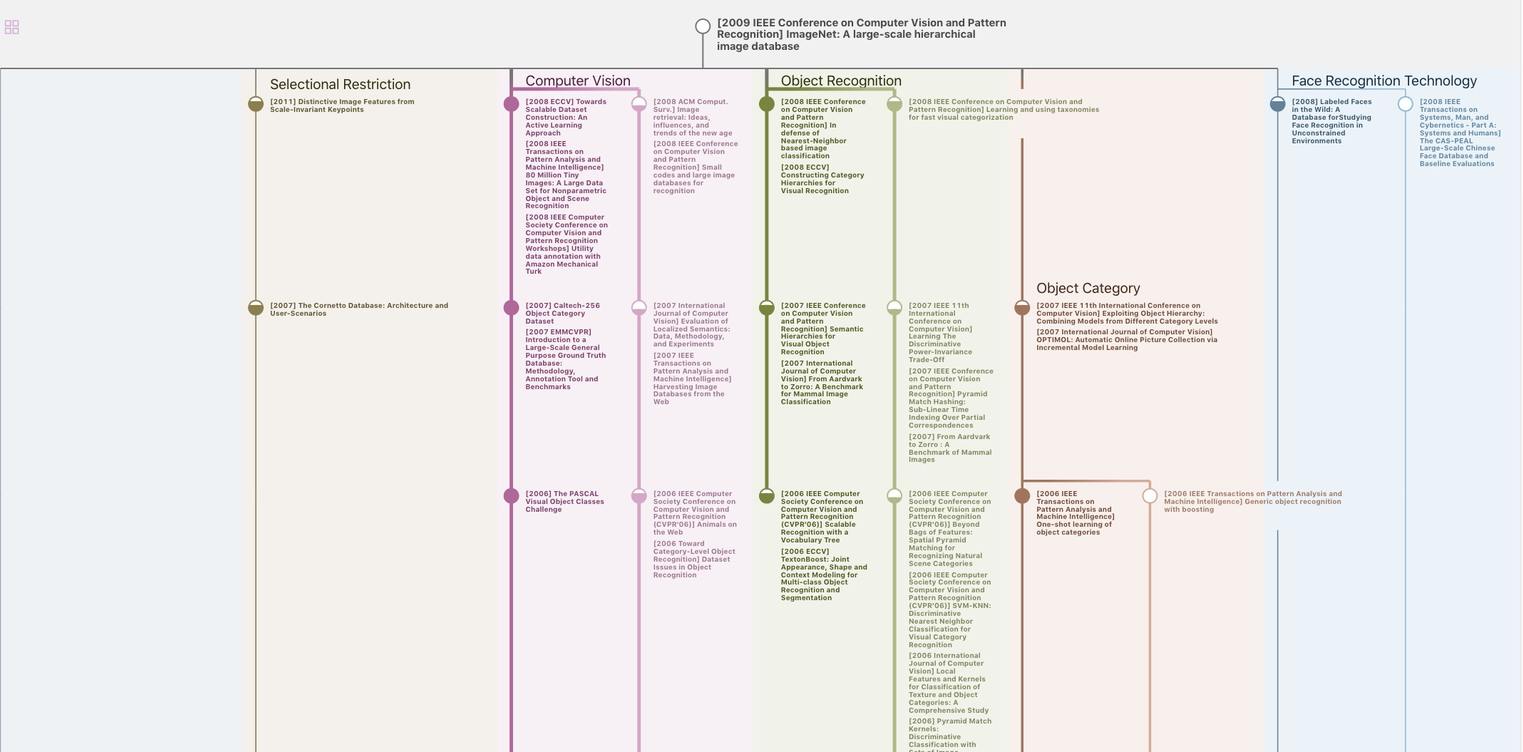
生成溯源树,研究论文发展脉络
Chat Paper
正在生成论文摘要