Heterogeneous Defect Prediction Based on Federated Transfer Learning Via Knowledge Distillation.
IEEE access(2021)
摘要
Heterogeneous defect prediction (HDP) aims to predict defect-prone software modules in one project using heterogeneous data collected from other projects. There are two characteristics of defect data: data islands, and data privacy. In this article, we propose a novel Federated Transfer Learning via Knowledge Distillation (FTLKD) approach for HDP, which takes into consideration two characteristics of defect data. Firstly, Shamir sharing technology achieves homomorphic encryption for private data. During subsequent processing and operations, data remains encrypted all the time. Secondly, each participant uses public data to train convolutional neural networks(CNN), the parameters of the pre-trained CNN are transferred to a private model. A small amount of labeled private data fine-tunes the private model. Finally, knowledge distillation realizes the communication between the participants. The average of all softmax output (logits) is used for knowledge distillation to update the private models. Extensive experiments on 9 projects in 3 public databases (NASA, AEEEM and SOFTLAB) show that FTLKD outperforms the related competing methods.
更多查看译文
关键词
Federated learning,homomorphic encryption,data island,knowledge distillation
AI 理解论文
溯源树
样例
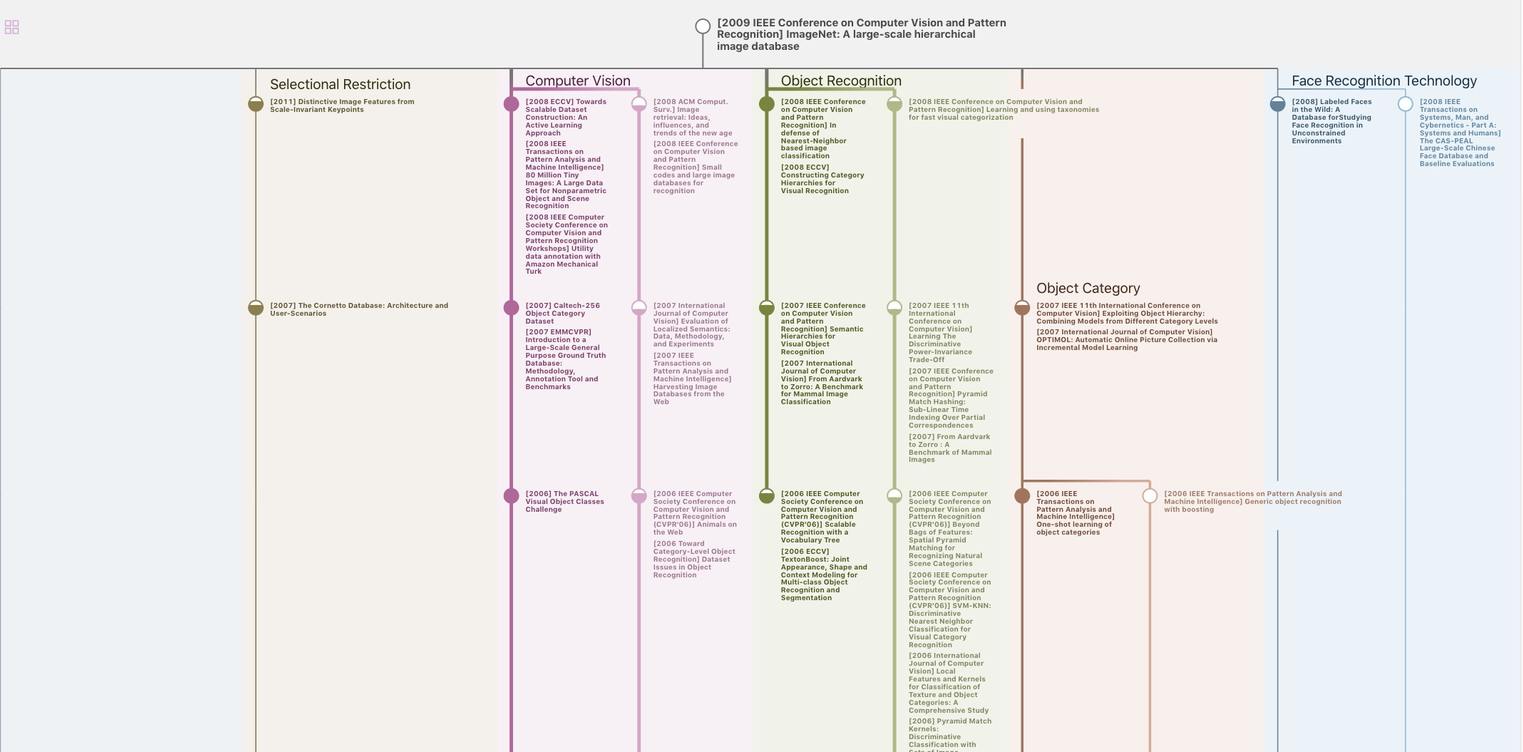
生成溯源树,研究论文发展脉络
Chat Paper
正在生成论文摘要