An exploration on the machine learning approaches to determine the erosion rates for liquid hydrocarbon transmission pipelines towards safer and cleaner transportations
Journal of Cleaner Production(2021)
摘要
Erosion is commonly found in pipelines with existence of interactions between solid particles, fluid product transported and the surrounding materials. Erosion may lead to material degradation in pipeline and may eventually cause pipeline failure. This failure may cause catastrophic consequence to the surrounding health, property and the environment. Therefore, a better estimation on the pipeline erosion rate is critical for the pipeline engineers to maintain a safer, more cost-effective and sustainable operating condition. In this study, two erosion rate models were used to create an erosion rate database and three machine-learning approaches were developed to help pipeline engineers evaluate the optimal flow velocity. The major results of this study are threefold: First, a purely visual approach was used to present critical information to decision makers. Second, three machine learning approaches (classification tree, artificial neural network and Bayesian network) were established for the erosion rate determination. Third, the benefits and limits of each approach were discussed and evaluated. As novel methodologies to estimate pipeline erosion rate, the machine learning approaches can serve as simple or comprehensive tools to address the various pipeline operating scenarios, with certain or uncertain information. This study provides innovative, intuitive and cost-effective tools for the pipeline operators to predict the erosion rate under various operating conditions and handles the uncertainty via a probabilistic approach, aiming to build safer and more environmental-friendly pipelines.
更多查看译文
关键词
Artificial neural network,Bayesian network,Classification tree,Erosion,Machine learning,Pipeline safety
AI 理解论文
溯源树
样例
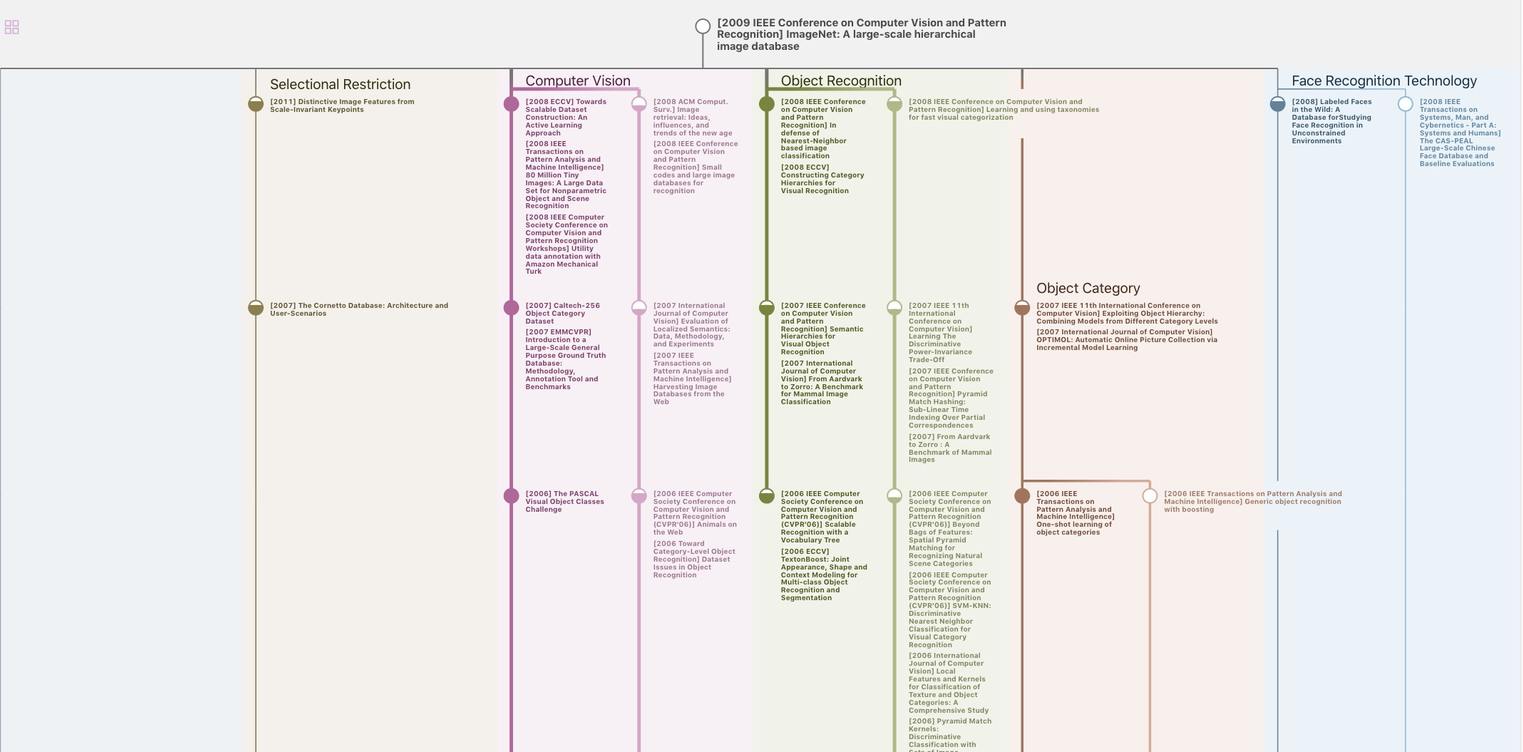
生成溯源树,研究论文发展脉络
Chat Paper
正在生成论文摘要