A Reinforcement Learning-Based Reconstruction Method for Complex Defect Profiles in MFL Inspection
IEEE TRANSACTIONS ON INSTRUMENTATION AND MEASUREMENT(2021)
摘要
Magnetic flux leakage (MFL) inspection is one of the most commonly used nondestructive evaluation (NDT) methods for detecting anomalies of ferromagnetic materials. Sizing of the defect with MFL signal is the key problem of inspection, which is important for evaluating the health condition of the material. However, it is an ill-posed inverse problem that is hard to solve and harder to be accurate. Forward models that give a highly accurate simulated signal with a corresponding depth profile are widely used in solving the inverse problem iteratively. Unfortunately, the policy which determines the iteration process is hard to design. In this article, a reinforcement learning (RL)-based algorithm is proposed to reconstruct the depth of defects with a complex depth profile. Instead of designing the policy, the iterative process of the classic iteration-based method is embedded into the learning process of the RL-based algorithm proposed in this article. The policy is learned from the data generated during the reconstructing iteration. By designing the states, actions, and rewards in the RL structure, the most computationally costly part of calling the forward model during iteration is avoided. An adaptive limited exploration process is given to balance the exploration and exploitation in the inverse problem of MFL inspection in this article. The effectiveness of the proposed algorithm is demonstrated with simulation results under different noise levels. The results demonstrate that the proposed algorithm is robust with good reconstruction accuracy.
更多查看译文
关键词
Complex defect, defect reconstruction, inverse problem, magnetic flux leakage (MFL) inspection, reinforcement learning (RL)
AI 理解论文
溯源树
样例
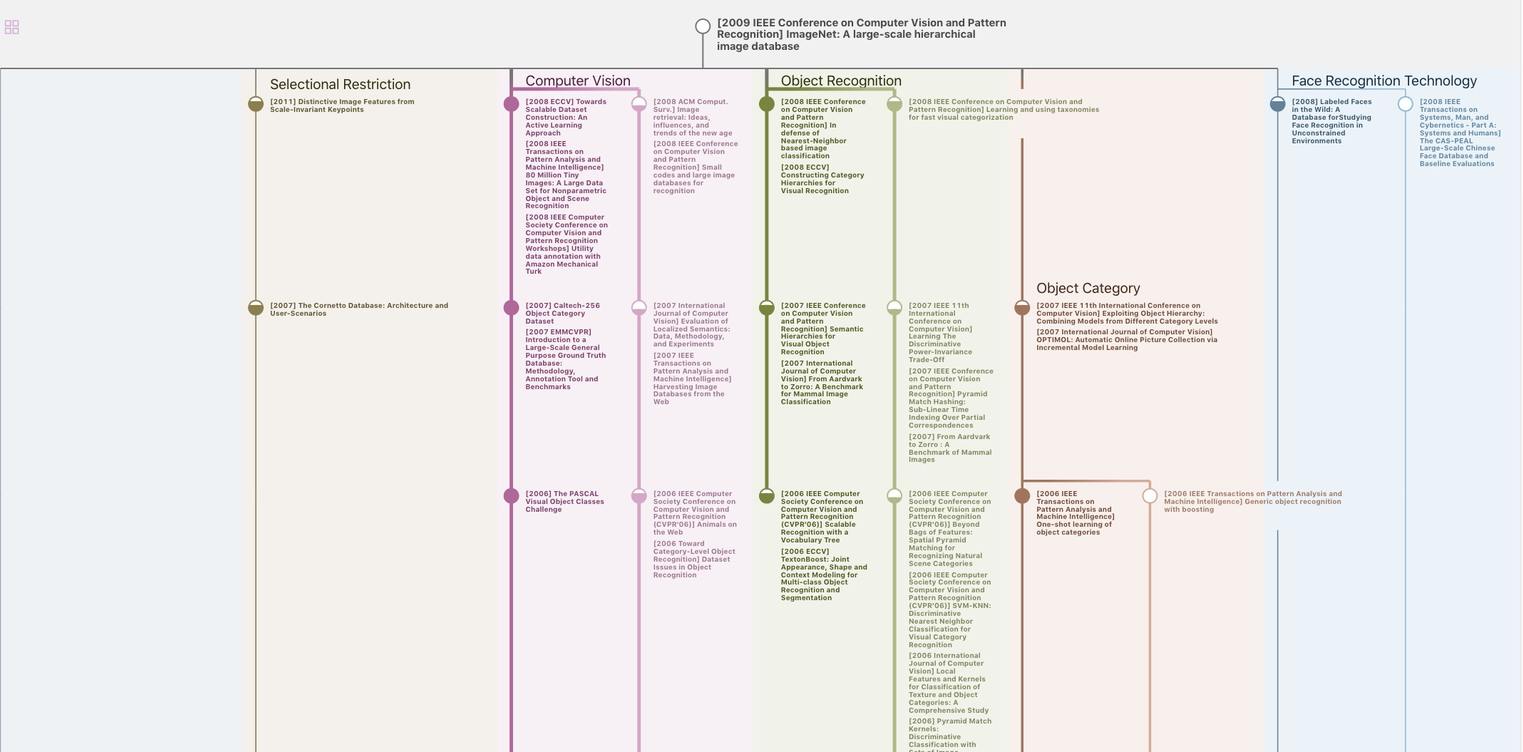
生成溯源树,研究论文发展脉络
Chat Paper
正在生成论文摘要