DND: Driver Node Detection for Control Message Diffusion in Smart Transportations
IEEE Transactions on Network and Service Management(2021)
关键词
Controllability,Vehicles,Vehicle dynamics,Internet,Computational modeling,Smart transportation,Peer-to-peer computing,IoT,dynamic network,Internet of Vehicles,network controllability,driver nodes
AI 理解论文
溯源树
样例
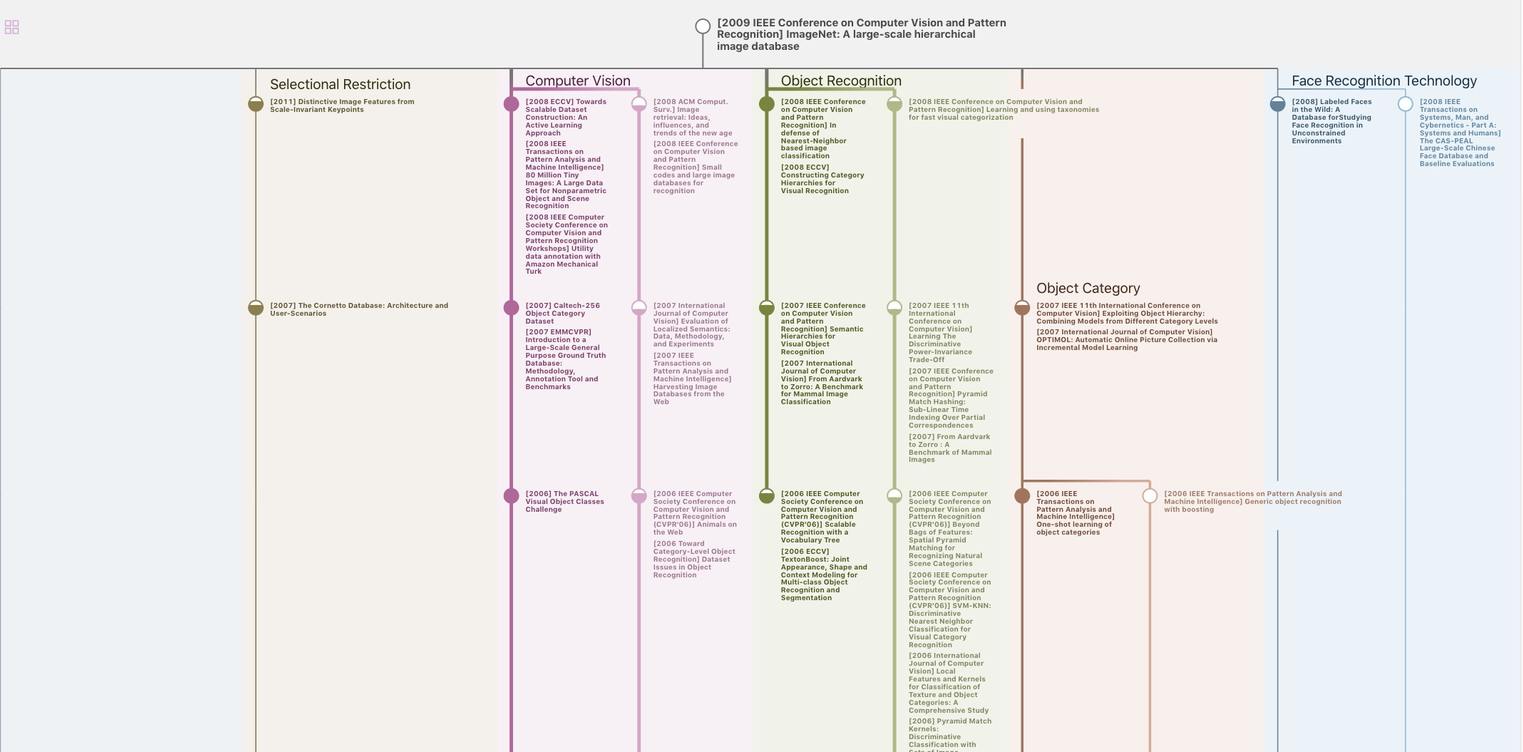
生成溯源树,研究论文发展脉络
Chat Paper
正在生成论文摘要