A Deep Reinforcement learning based Approach for Channel Aggregation in IEEE 802.11ax
GLOBECOM(2020)
摘要
Channel aggregation (CA) is proposed in IEEE 802.11ax to allow wireless users to aggregate multiple available channels, either contiguous or non-contiguous, to improve the network throughput. In this paper, the performance of CA is extensively investigated. It is shown that a simple CA that aggregates all available channels does not always promote but may degrade the network performance due to the increased inter-channel contentions in a random access wireless local area network (WLAN). Thus, it is of critical importance to select an appropriate set of channels for CA. To this end, we propose an efficient probabilistic channel aggregation scheme to maximize the network throughput under the quality of service constraints. That is, an ax user aggregates each secondary channel with a certain probability based on the traffic load of the secondary channel. A Proximal Policy Optimization (PPO) based approach is further applied to intelligently tune the aggregating probabilities of secondary channels to maximize the network throughput. Numerical results show that the proposed algorithm can greatly improve the network throughput compared with existing CA algorithms in the literature.
更多查看译文
关键词
IEEE 802.11ax, probabilistic Channel aggregation, Proximal Policy Optimization (PPO)
AI 理解论文
溯源树
样例
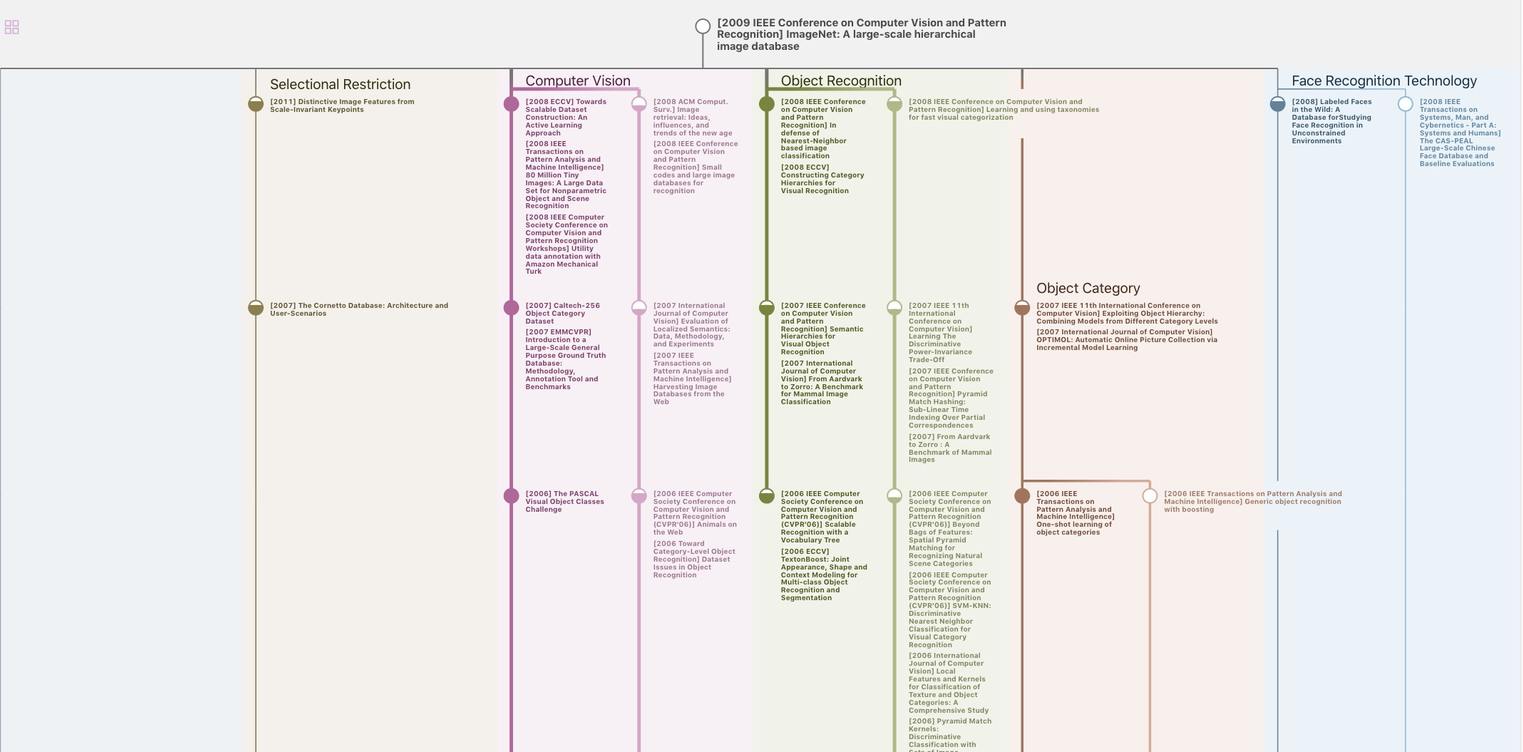
生成溯源树,研究论文发展脉络
Chat Paper
正在生成论文摘要