Resource Management And Model Personalization For Federated Learning Over Wireless Edge Networks
JOURNAL OF SENSOR AND ACTUATOR NETWORKS(2021)
摘要
Client and Internet of Things devices are increasingly equipped with the ability to sense, process, and communicate data with high efficiency. This is resulting in a major shift in machine learning (ML) computation at the network edge. Distributed learning approaches such as federated learning that move ML training to end devices have emerged, promising lower latency and band-width costs and enhanced privacy of end users' data. However, new challenges that arise from the heterogeneous nature of the devices' communication rates, compute capabilities, and the limited observability of the training data at each device must be addressed. All these factors can significantly affect the training performance in terms of overall accuracy, model fairness, and convergence time. We present compute-communication and data importance-aware resource management schemes optimizing these metrics and evaluate the training performance on benchmark datasets. We also develop a federated meta-learning solution, based on task similarity, that serves as a sample efficient initialization for federated learning, as well as improves model personalization and generalization across non-IID (independent, identically distributed) data. We present experimental results on benchmark federated learning datasets to highlight the performance gains of the proposed methods in comparison to the well-known federated averaging algorithm and its variants.
更多查看译文
关键词
federated learning, wireless edge networks, resource management, personalization
AI 理解论文
溯源树
样例
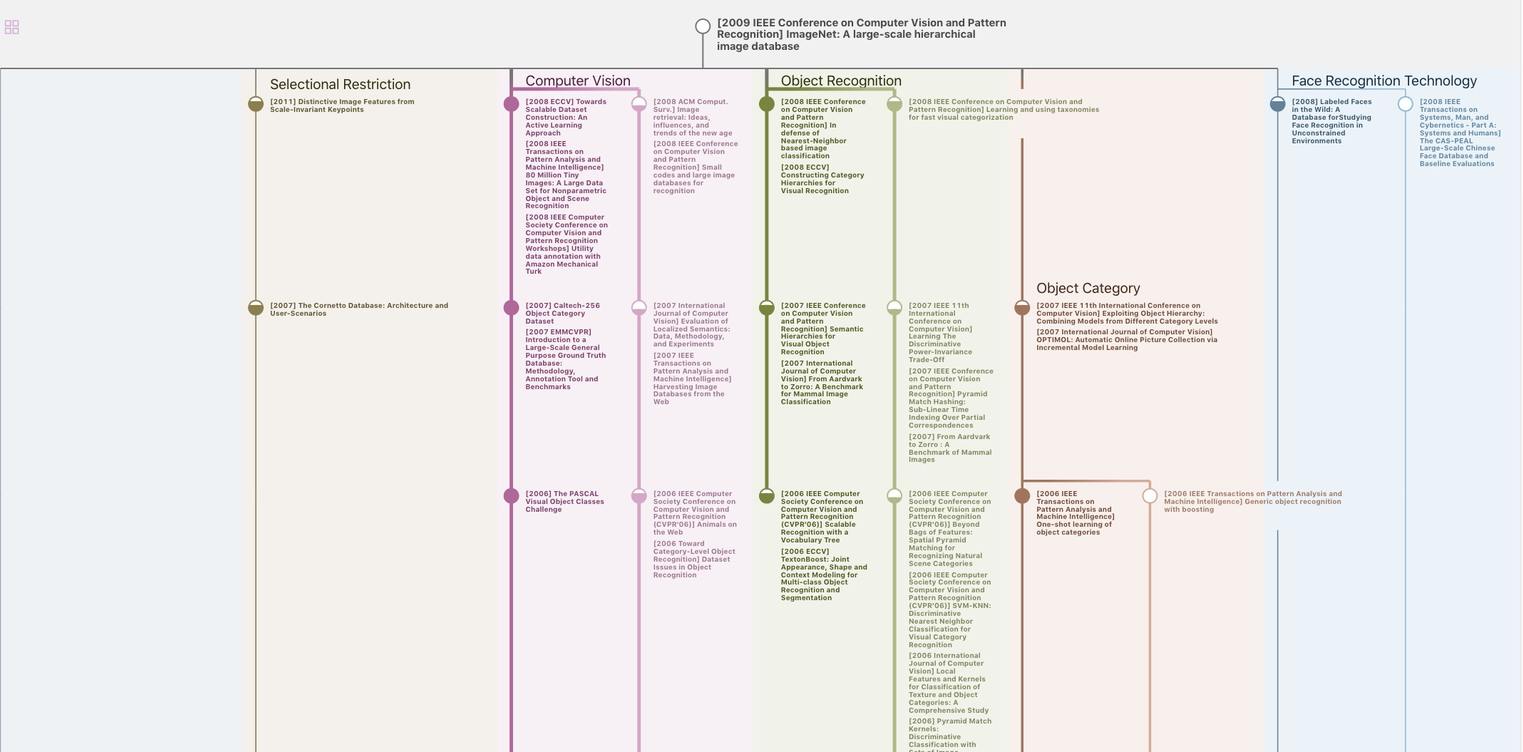
生成溯源树,研究论文发展脉络
Chat Paper
正在生成论文摘要