Explicit Pareto Front Optimization for Constrained Reinforcement Learning
user-5f8cf9244c775ec6fa691c99(2021)
摘要
Many real-world problems require that reinforcement learning (RL) agents learn policies that not only maximize a scalar reward, but do so while meeting constraints, such as remaining below an energy consumption threshold. Typical approaches for solving constrained RL problems rely on Lagrangian relaxation, but these suffer from several limitations. We draw a connection between multi-objective RL and constrained RL, based on the key insight that the constraint-satisfying optimal policy must be Pareto optimal. This leads to a novel, multi-objective perspective for constrained RL. We propose a framework that uses a multi-objective RL algorithm to find a Pareto front of policies that trades off between the reward and constraint(s), and simultaneously searches along this front for constraint-satisfying policies. We show that in practice, an instantiation of our framework outperforms existing approaches on several challenging continuous control domains, both in terms of solution quality and sample efficiency, and enables flexibility in recovering a portion of the Pareto front rather than a single constraint-satisfying policy.
更多查看译文
关键词
Lagrangian relaxation,Reinforcement learning,Multi-objective optimization,Mathematical optimization,Energy consumption,Scalar (physics),Computer science,Pareto optimal
AI 理解论文
溯源树
样例
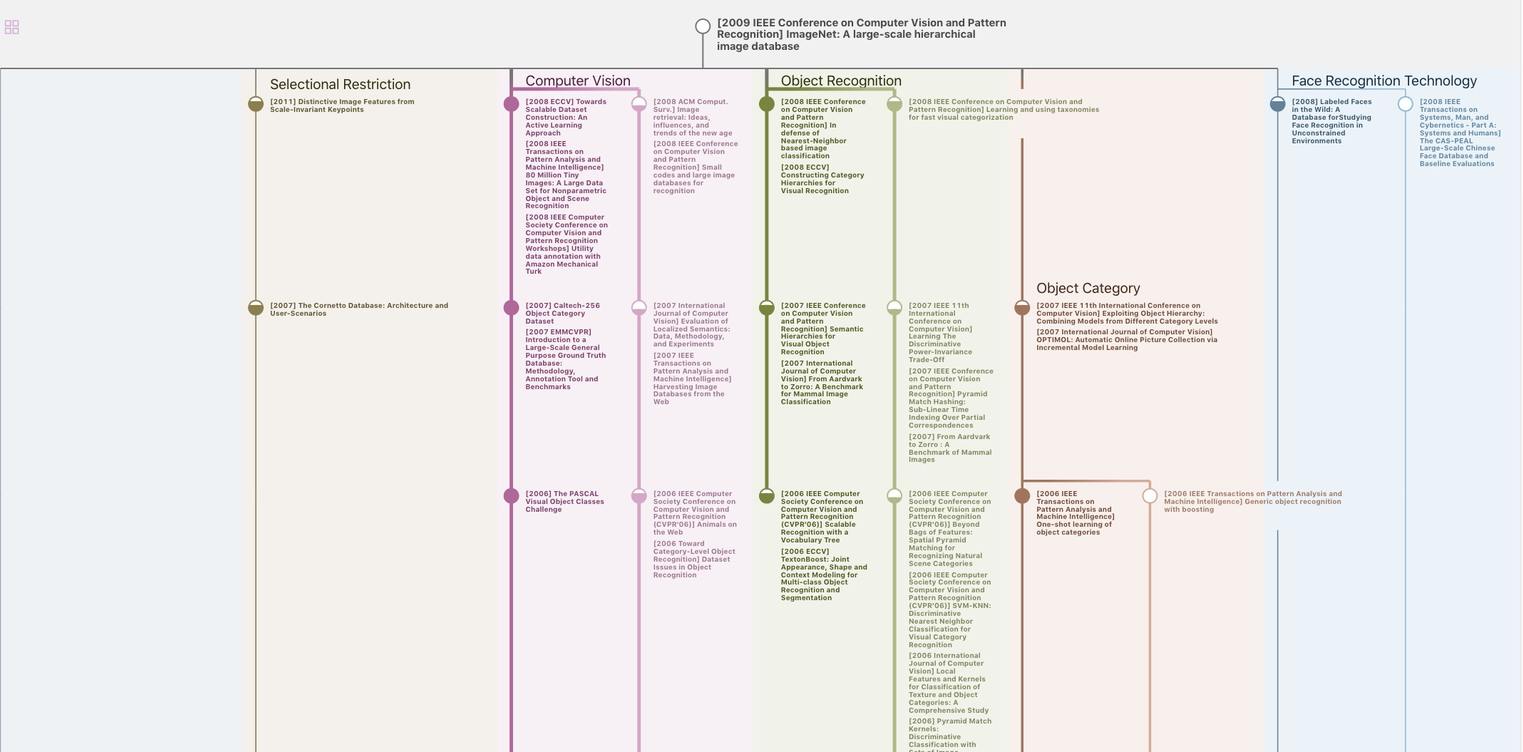
生成溯源树,研究论文发展脉络
Chat Paper
正在生成论文摘要