Non-parallel Sequence-to-Sequence Voice Conversion for Arbitrary Speakers
2021 12th International Symposium on Chinese Spoken Language Processing (ISCSLP)(2021)
摘要
Voice conversion (VC) aims to modify the speaker's tone while preserving the linguistic information. Recent works show that voice conversion has made great progress on non-parallel data by introducing phonetic posteriorgrams (PPGs). However, once the prosody of source and target speaker differ significantly, it causes noticeable quality degradation of the converted speech. To alleviate the impact of the prosody of the source speaker, we propose a sequence-to-sequence voice conversion (Seq2SeqVC) method, which utilizes connectionist temporal classification PPGs (CTC-PPGs) as inputs and models the non-linear length mapping between CTC-PPGs and frame-level acoustic features. CTC-PPGs are extracted by the CTC based automatic speech recognition (CTC-ASR) model and used to replace time-aligned PPGs. The blank token is introduced in CTC-ASR outputs to identify fewer information frames and get around consecutive repeating characters. After removing blank tokens, the left CTC-PPGs only contain linguistic information, and the phone duration information of the source speech is removed. Thus, phone durations of the converted speech are more faithful to the target speaker, which means higher similarity to the target and less interference from different source speakers. Experimental results show our Seq2Seq-VC model achieves higher scores in similarity and naturalness tests than the baseline method. What's more, we expand our seq2seqVC approach to voice conversion towards arbitrary speakers with limited data. The experimental results demonstrate that our Seq2Seq-VC model can transfer to a new speaker using 100 utterances (about 5 minutes).
更多查看译文
关键词
voice conversion,sequence-to-sequence,connectionist temporal classification,limited data
AI 理解论文
溯源树
样例
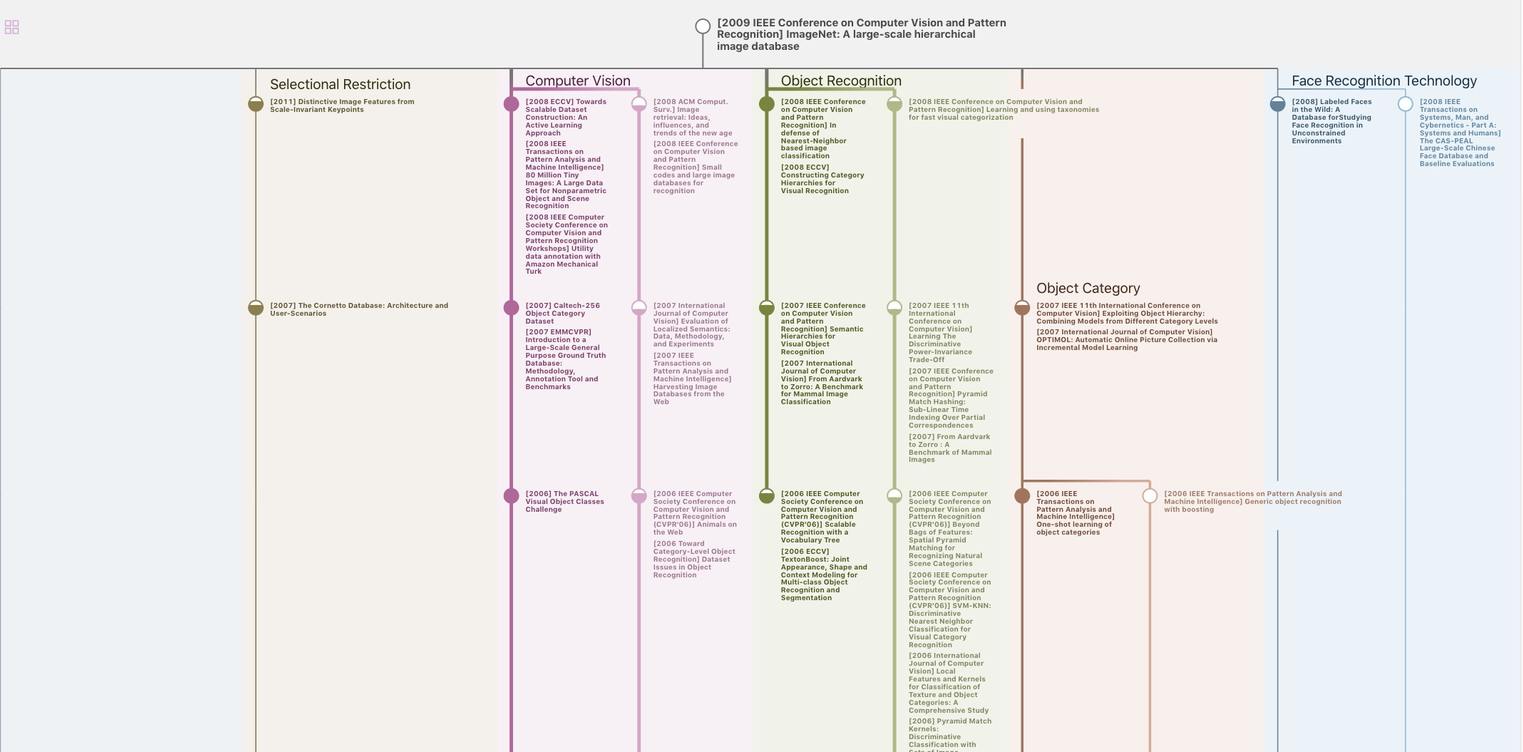
生成溯源树,研究论文发展脉络
Chat Paper
正在生成论文摘要