Trust Your Data Or Not-Stqp Remains Stqp: Community Detection Via Robust Standard Quadratic Optimization
MATHEMATICS OF OPERATIONS RESEARCH(2021)
摘要
We consider the robust standard quadratic optimization problem (RStQP), in which an uncertain (possibly indefinite) quadratic form is optimized over the standard simplex. Following most approaches, we model the uncertainty sets by balls, polyhedra, or spectrahedra, more generally, by ellipsoids or order intervals intersected with subcones of the copositive matrix cone. We show that the copositive relaxation gap of the RStQP equals the minimax gap under some mild assumptions on the curvature of the aforementioned uncertainty sets and present conditions under which the RStQP reduces to the standard quadratic optimization problem. These conditions also ensure that the copositive relaxation of an RStQP is exact. The theoretical findings are accompanied by the results of computational experiments for a specific application from the domain of graph clustering, more precisely, community detection in (social) networks. The results indicate that the cardinality of communities tend to increase for ellipsoidal uncertainty sets and to decrease for spectrahedral uncertainty sets.
更多查看译文
关键词
robust optimization, quadratic optimization, community detection, social networks
AI 理解论文
溯源树
样例
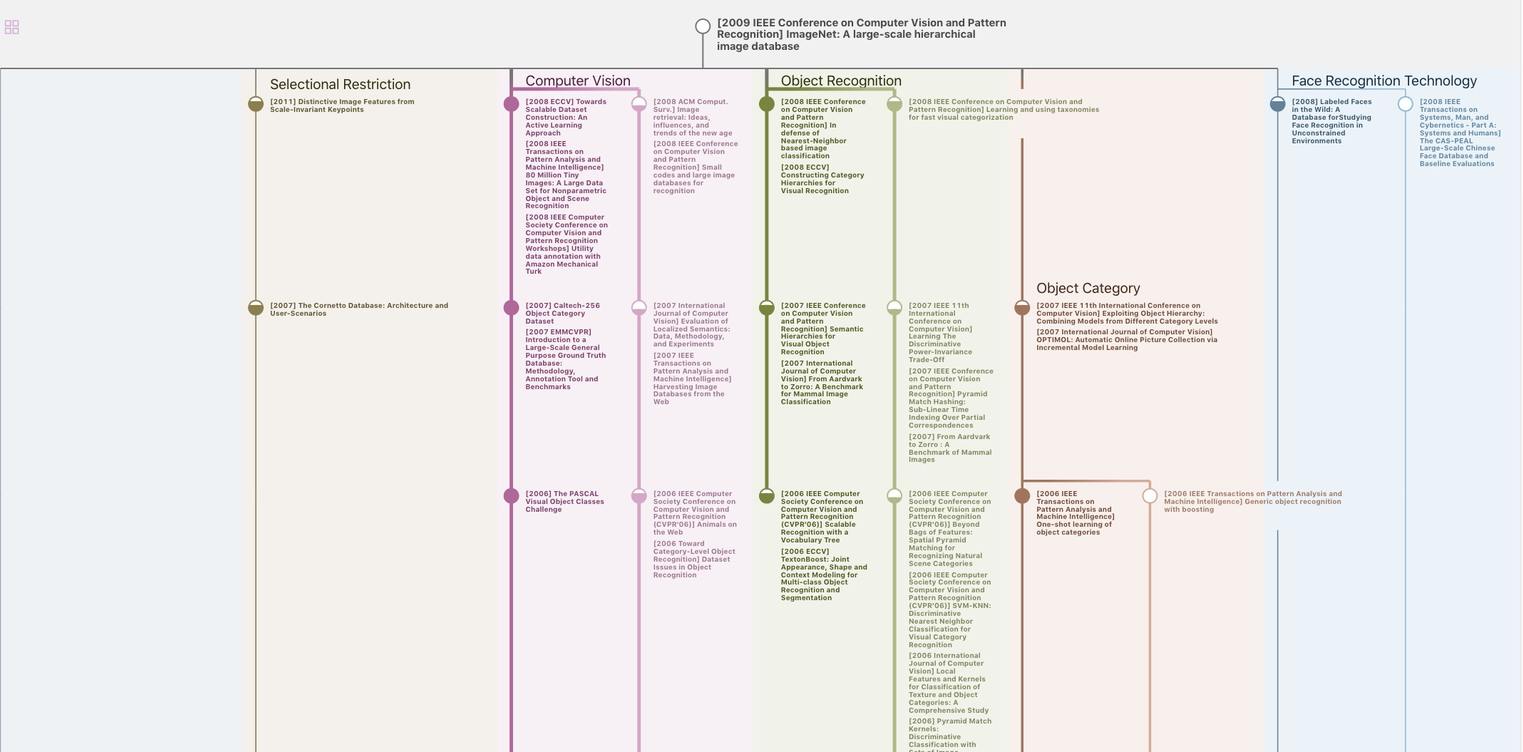
生成溯源树,研究论文发展脉络
Chat Paper
正在生成论文摘要